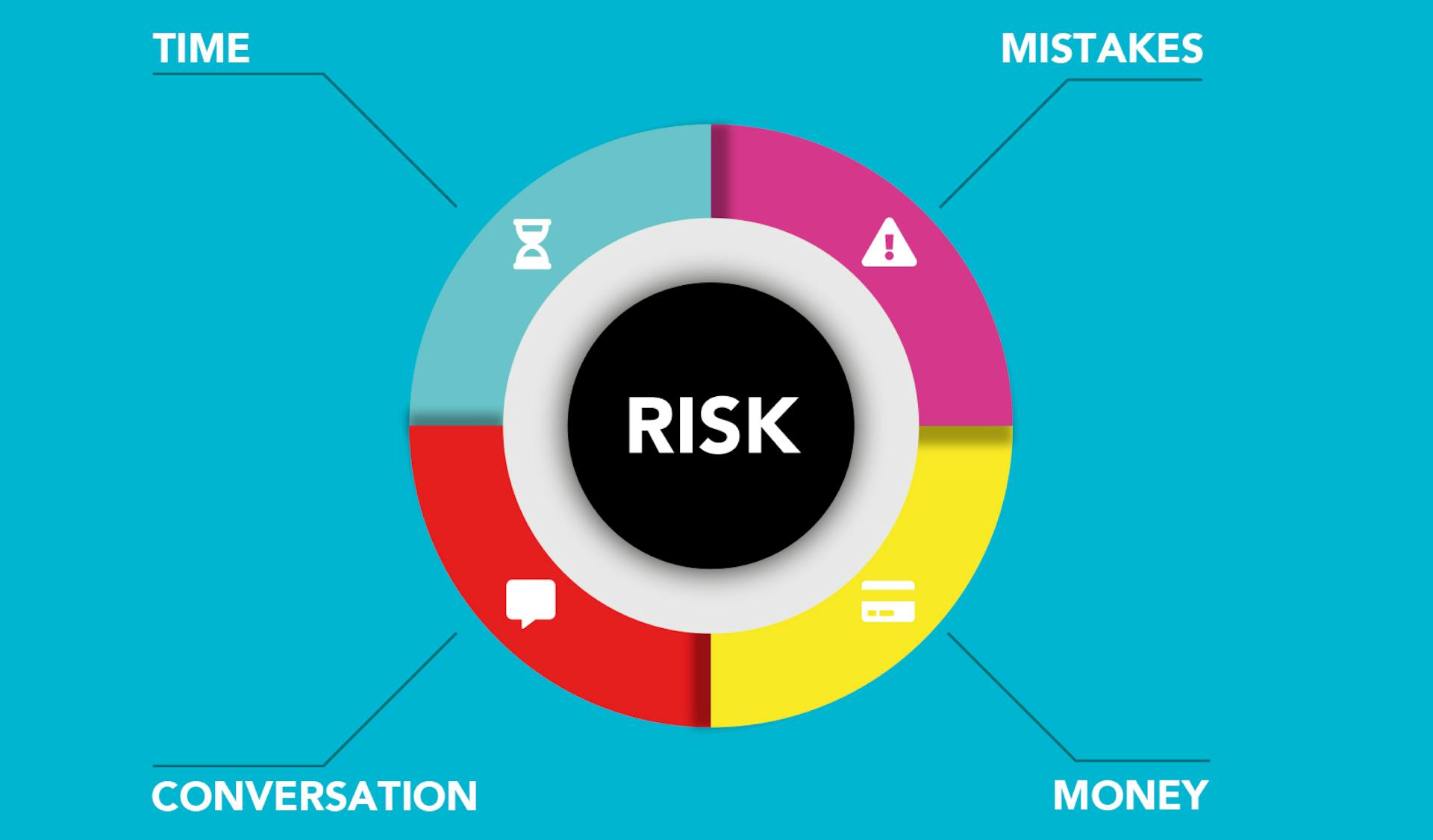
The expected shortfall formula is a crucial tool in risk management, and understanding how it works is essential for making informed decisions.
The expected shortfall formula is calculated using the following formula: ES = ∫(-∞)0 (x - VaR) f(x) dx, where ES is the expected shortfall, VaR is the value at risk, and f(x) is the probability density function.
This formula is derived from the concept of tail risk, which is the risk of extreme losses that occur with a low probability.
In simple terms, the expected shortfall formula measures the average loss that occurs in the worst 1% of scenarios.
Discover more: Expected Shortfall
Definition and Basics
Expected shortfall is a risk measure that helps us understand the potential losses of a portfolio. It's defined as the average loss exceeding a given confidence level, which is also known as the Value at Risk (VaR).
The expected shortfall is calculated using the formula: E[-X | X ≤ -VaRα(X)], where VaRα(X) is the Value at Risk. This formula gives us the expected loss beyond the VaR level, which is a more accurate measure of risk than VaR alone.
In simple terms, expected shortfall asks the question: "in case of losses so severe that they occur only alpha percent of the time, what is our average loss?"
Here's an interesting read: Coherent Risk Measure
Formal Definition
Expected shortfall is defined as the expected loss in case of extreme losses, and it's a crucial concept in finance. This definition is formalized as E[-X|X≤-VaRα(X)], where VaRα(X) is the value at risk.
The expected shortfall can also be expressed as E[-X|X≤-VaRα(X)] = α^(-1) * ∫_{-∞}^{-VaRα(X)} x * dF(x), where F(x) is the cumulative distribution function of X.
In simpler terms, expected shortfall is the average loss when losses are so severe that they occur only α percent of the time. This is a key concept in understanding risk management.
For continuous distributions, the expected shortfall is equivalent to the tail conditional expectation, TCEα(X) = E[-X|X≤-VaRα(X)]. This means that the expected shortfall can be calculated using the tail of the distribution.
In the case of a continuous distribution, the expected shortfall can be calculated as the negative of the expected value of the portfolio. This is because the 100%-quantile expected shortfall, ES1, equals the negative of the expected value of the portfolio.
Here's an interesting read: Tail Value at Risk
Value at Risk Basics
Value at Risk (VaR) is a concept used for financial risk measurement to evaluate a portfolio's market risk or credit risk. It's defined as the maximum possible loss over a given holding period within a fixed confidence level.
VaR satisfies the first three conditions of a risk measure: monotonicity, translation invariance, and homogeneity. However, it doesn't always satisfy the fourth condition, sub-additivity.
VaR measures the distribution quantile, disregarding severe losses beyond the VaR level. This can lead to ignoring critical information regarding the tails of the underlying distributions.
Here are the properties of a risk measure that VaR satisfies:
- Monotonicity: if a portfolio has lower returns than another, its VaR should be lower.
- Translation invariance: if we add a given amount of cash to a portfolio, its VaR should go down according to the proportion of cash added.
- Homogeneity: changing the size of a portfolio by a factor Y while keeping the relative amounts of different items in the portfolio the same should result in the VaR being multiplied by Y.
VaR has problems measuring extreme price movements, which can lead to different risk levels for portfolios with the same VaR.
Curious to learn more? Check out: Expected Shortfall vs Var
Expected Shortfall Formula
The Expected Shortfall formula is a crucial tool for measuring risk in finance. It's a single number that represents the average loss of the worst performing percent of the portfolio.
The formula for computing Expected Shortfall from the analytic formula is given by ESα=σportfolio(ϕ(Φ-1(α))1-α)-μportfolio. This is a mathematical equation that takes into account the standard deviation of the portfolio returns, the mean portfolio return, and the confidence level.
For instance, if we assume a mean portfolio return of 0, the formula simplifies to ESα=σportfolio(ϕ(Φ-1(α))1-α). This shows how the standard deviation of the portfolio returns plays a key role in determining the Expected Shortfall.
The probability distribution function (pdf) for the normal distribution, denoted by ϕ, is used to calculate the Expected Shortfall. The cumulative distribution function (cdf) of the normal distribution, denoted by Φ, is also used in the formula.
The confidence level, denoted by α, is typically set to 0.95. This means that the Expected Shortfall is calculated for the worst performing 5% of the portfolio.
If this caught your attention, see: Which Formula Can Be Used to Describe the Sequence?
Properties and Calculation
Expected shortfall increases as the confidence level q decreases.
The expected shortfall ESq is greater than or equal to the Value at Risk VaRq at the same q level. This means that as the confidence level decreases, the expected shortfall will be higher.
To calculate expected shortfall, you average all the returns worse than the portfolio's VaR at a given level of confidence.
Properties
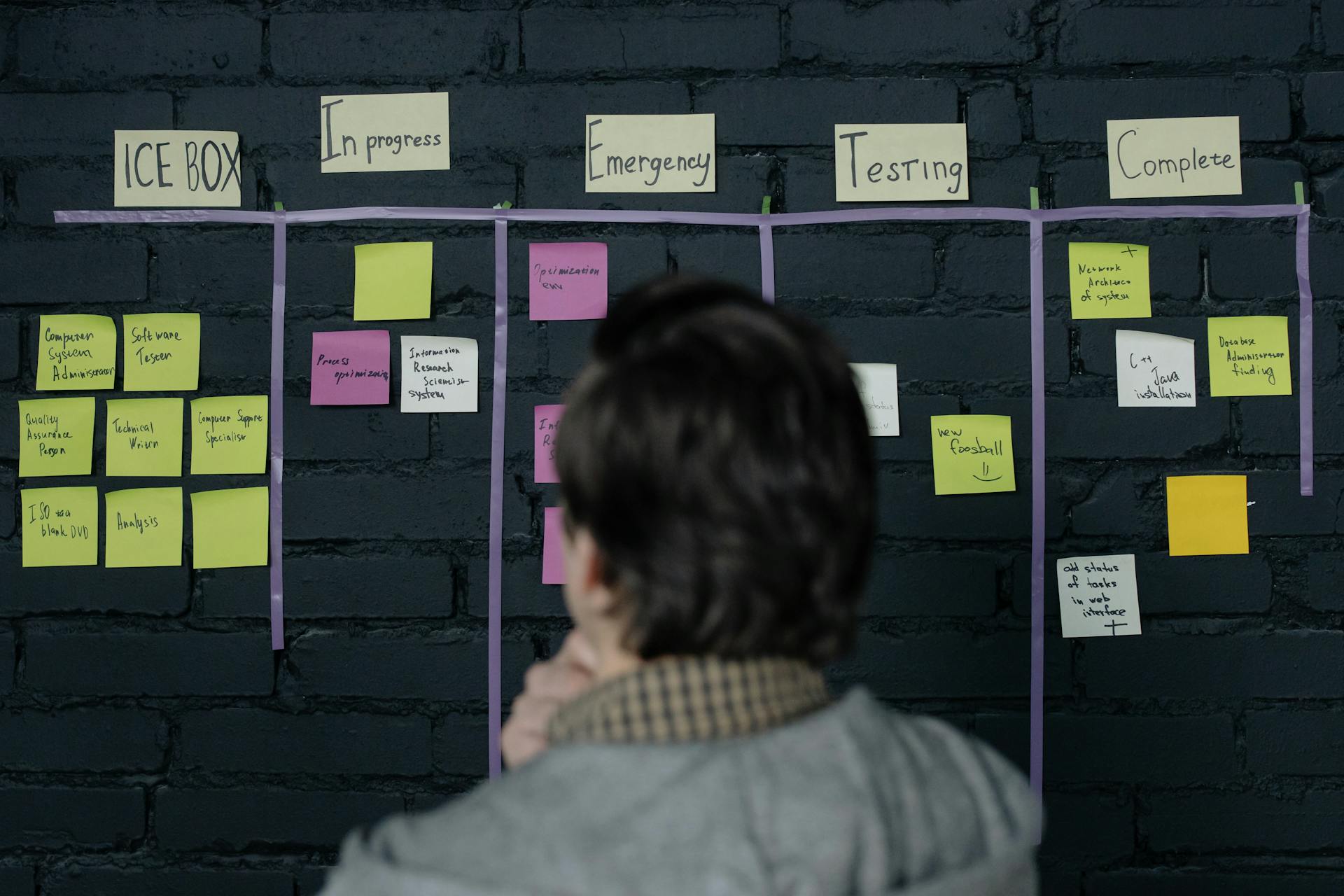
Expected Shortfall (ES) is always a larger number than Value at Risk (VaR) because it's the average of losses that exceed the VaR. This is a fundamental property of ES.
The ES measures the risk of an investment in a more conservative way, focusing on the less profitable outcomes. It's a more comprehensive measure than VaR, as it takes into account the entire distribution of potential losses, not just the worst-case scenario.
ES estimates the risk of an investment by averaging the losses that exceed the VaR threshold. This is done by calculating the average of the tail losses from the VaR threshold to the worst possible outcome.
The ES value is sensitive to the inclusion or exclusion of very low probability extreme events, which can lead to less stable estimates compared to VaR. This is because ES is more sensitive to outliers in the distribution of potential losses.
A key property of ES is that it's the expected return on the portfolio in the worst X% of cases. This means that a higher ES value indicates a more volatile investment with a greater potential for losses.
ES is often used in conjunction with VaR to provide a more complete picture of an investment's risk profile. By combining these two measures, investors can get a better sense of the potential risks and rewards of an investment.
You might enjoy: Risk Measures
Log-Logistic
The log-logistic distribution is a type of distribution that can be used to model portfolio payoffs and losses. It's a bit of a mouthful, but stick with me!
If a portfolio's payoff follows a log-logistic distribution, the expected shortfall can be calculated using a specific formula. The formula involves the regularized incomplete beta function, Iα, and is given by ESα(X) = 1 - e^μ / α * Iα(1+s, 1-s) * πs / sin(πs).
For a more generic case, the expected shortfall can be expressed using the hypergeometric function. This is useful when the incomplete beta function is not defined for certain arguments. The formula is ESα(X) = 1 - e^μ * α^s / (s+1) * 2F1(s, s+1; s+2; α).
The log-logistic distribution can also be used to model losses, and the expected shortfall can be calculated using a different formula. This formula involves the hyperbolic cosecant function and the incomplete beta function, and is given by ESα(L) = a / (1-α) * [π / b * csc(π/b) - Bα(1/b+1, 1-1/b)].
Calculation Methods
To calculate Expected Shortfall (ES), you need to average the returns worse than the portfolio's Value-at-Risk (VaR) at a given level of confidence.
The formula for ES is straightforward, but it requires knowing the potential tail losses of your investment. This can be done by using a statistical distribution or historical data.
To calculate ES using a statistical distribution, you need to ensure it correctly represents the tails of your distribution. This is especially important for stock returns, which have fat tails compared to a Gaussian distribution.
Collapse Then Estimate
In the "Collapse Then Estimate" method, we use the current weights to create a history of portfolio returns. This approach allows us to get the prediction distribution given the portfolio return history.
There are several ways to achieve this, including fitting an assumed distribution, simulation, GARCH prediction, and GARCH simulation.
Fitting an assumed distribution can be done by estimating the standard deviation to get your prediction distribution, assuming a normal distribution. Alternatively, if you assume a t-distribution, you need to estimate the degrees of freedom or assume the degrees of freedom.
You might like: Historical Simulation (finance)
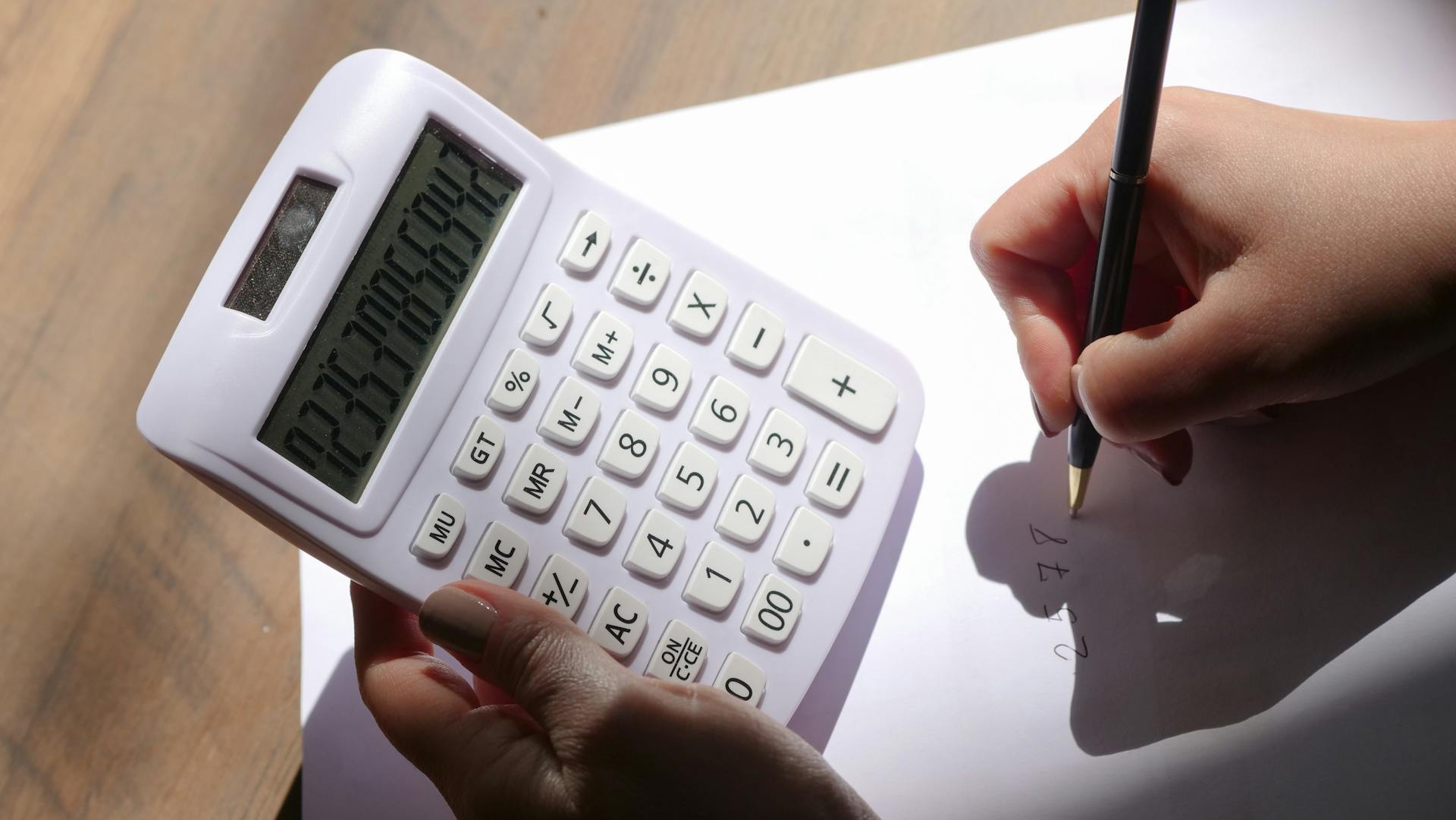
The simulation method uses the empirical distribution of a specific number of portfolio returns. Using a univariate GARCH model can also be a good way to estimate VaR and ES.
One approach for VaR is to directly estimate the quantile without worrying about the distribution, as seen in CaViaR by Engle and Manganelli.
Logistic
The logistic distribution is a type of distribution that can be used to model portfolio payoffs and losses. It's characterized by a probability density function (p.d.f.) and a cumulative distribution function (c.d.f.).
The p.d.f. of the logistic distribution is given by f(x) = 1/s * e^(-((x-μ)/s)) * (1 + e^(-((x-μ)/s)))^-2, where μ is the location parameter and s is the scale parameter. This function describes the probability of a portfolio payoff occurring at a given value x.
The c.d.f. of the logistic distribution is F(x) = (1 + e^(-((x-μ)/s)))^-1. This function gives the probability that a portfolio payoff will be less than or equal to a given value x.
The expected shortfall (ES) of a portfolio is a measure of its potential loss. For a portfolio that follows the logistic distribution, the ES can be calculated using the formula ESα(X) = -μ + s * ln((1-α)^(1-(1/α)) / α). This formula takes into account the probability of a portfolio payoff being less than a given threshold α.
In the case of a loss distribution, the ES can be calculated using the formula ESα(L) = μ + s * (-α * ln(α) - (1-α) * ln(1-α)) / (1-α). This formula is similar to the previous one, but it's adjusted for the fact that we're dealing with a loss distribution.
Generalized Hyperbolic Secant (GHS)
The Generalized Hyperbolic Secant (GHS) distribution is a mathematical concept that describes the behavior of a portfolio's payoff.
It has a probability density function (p.d.f.) given by f(x) = 1/(2σ) sech((π/2)(x-μ)/σ).
This distribution is particularly useful in finance, where it's used to model the behavior of assets and portfolios.
The cumulative distribution function (c.d.f.) of the GHS distribution is F(x) = 2/π arctan(exp((π/2)(x-μ)/σ)).
The expected shortfall of a portfolio following the GHS distribution is a critical metric that can help investors make informed decisions.
It's calculated using the formula ESα(X) = -μ - (2σ/π) ln(tan(πα/2)) - (2σ/π^2α)i[L2(-itan(πα/2)) - L2(itan(πα/2))].
The dilogarithm function, denoted as L2, plays a crucial role in this calculation, and its values can be used to derive the expected shortfall.
Optimization
Optimization is a crucial step in calculating expected shortfall, and it's made possible by transforming the problem into a linear program. This is achieved by introducing an auxiliary function Fα α (w,γ γ ) that is convex with respect to γ γ .
The key contribution of Rockafellar and Uryasev's 2000 paper is to introduce the auxiliary function Fα α (w,γ γ ), which makes expected shortfall a cornerstone of alternatives to mean-variance portfolio optimization.
To numerically compute the expected shortfall, it's necessary to generate J simulations of the portfolio constituents, often done using copulas. This results in an approximated auxiliary function F~ ~ α α (w,γ γ ) that can be used in optimization.
The approximated auxiliary function F~ ~ α α (w,γ γ ) is equivalent to a linear program, which can be solved using standard methods.
Value at Risk Estimation
To calculate VaR, you need to load the data, calculate the returns, and set the window variables for the rolling data window. This involves loading an equity portfolio to analyze, calculating the dollar value of exposure to each stock, and using norminv to find the z-score for the specified confidence level.
You'll also need to initialize the VaR, σportfolio, and profit and loss (P&L) vectors, which are used for backtesting in ES Backtest. For each trading day, you estimate VaR using the covariances of the returns, the amount invested in each asset, and the z-score for the confidence level.
A risk metric is a concept used for financial risk measurement to evaluate a portfolio's market risk or credit risk. It can be thought of as the amount of cash that must be added to a position to make its risk acceptable.
There are several properties that a risk measure should have, including monotonicity, translation invariance, homogeneity, and sub-additivity. A risk measure is coherent if it satisfies all four conditions.
Consider reading: Spectral Risk Measure
To estimate Value at Risk and Expected Shortfall, you can use R, and there are some hints available for doing it. An introduction to estimating Value at Risk and Expected Shortfall can be found in this article.
ES (Expected Shortfall) is essentially the probability-weighted average of tail losses and is calculated up from the VaR threshold. To estimate the ES for a given VaR value, you can average a set of VaR values with a greater confidence level.
Here's a step-by-step guide to estimating ES as VaR average:
- Create a grid of confidence levels greater than 95%
- Calculate the associated esZScores
- Multiply esZScores by the saved σportfolio values to calculate the new VaR values
- Average the computed VaR estimates using the mean function to get ES estimates
Abbreviations
Abbreviations can be a real challenge, especially in complex fields like finance.
The abbreviation for Value at Risk, VaR, has a potential pitfall: it can be confused with variance and vector autoregression.
To avoid confusion, a simple convention on capitalization can be used: VaR for Value at Risk, var for variance, and VAR for vector autoregression.
Here's a quick reference guide to keep them straight:
Plot Results for ES Methods
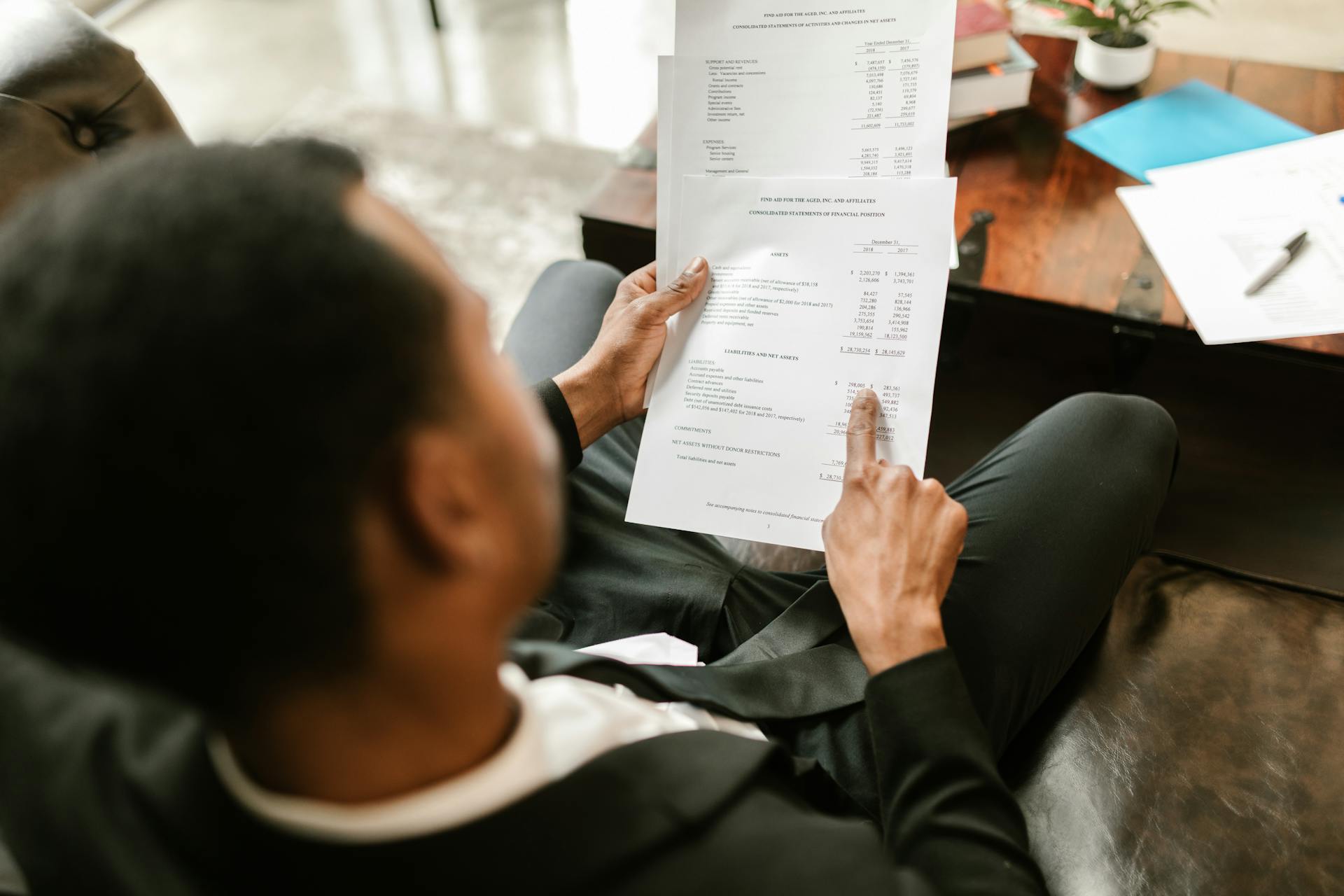
The plots of ES values versus trading day show that the three sets of ES values are similar.
In fact, the plots reveal that esByVaRAverage consistently comes in lowest, indicating a slightly lower ES estimate.
This is because the VaR average uses only nine VaR values, which misses a larger fraction of the tail of the returns distribution.
This results in a lower ES estimate compared to the other methods, which capture a larger portion of the tail.
Explore further: Conditional Tail Expectation
Estimation and Backtesting
Backtesting is a crucial step in validating the accuracy of expected shortfall (ES) values. You can use the Risk Management Toolbox's esbacktest function to do this, which has two tests: the unconditional normal and t tests. These tests don't require information about the portfolio return distribution, making them flexible, but also relatively limited in terms of accuracy.
The esbacktest function can be used with explicit distributional assumptions, but it's best to use esbacktestbysim for more comprehensive testing. This function runs a suite of tests on the ES data, including simulating a set of portfolio returns to compare against actual data.
If the computed ES values don't match the actual portfolio performance, it may indicate that the distribution assumptions are inadequate. In this case, consider using alternative methods, such as modeling portfolio returns with a t-distribution or using a nonparametric method like historical VaR.
Multivariate Estimation
Multivariate estimation is a way to understand the sources of risk in a portfolio. It's a more nuanced approach than just getting a single risk number.
By estimating a variance matrix of the asset returns, you can see how each asset contributes to the overall portfolio risk. This is especially useful if you want to identify which assets are driving the risk.
This approach is most likely to be used when you want to drill down into the specifics of portfolio risk, rather than just looking at a single number.
Curious to learn more? Check out: Redress Number
Univariate Estimation
Univariate estimation is a simpler approach to modeling, especially when dealing with a single time series of returns for the portfolio. You can get this by matrix-multiplying the matrix of simple returns of the assets in the portfolio by the portfolio weights.
In R notation, this is represented as: R1 <- R %*% w, where R is the matrix of simple returns and w is the vector of portfolio weights. Alternatively, you can use R1 <- rowSums(R * w), which is a safer approach.
Log returns are often preferred for modeling, and you can transform from simple to log returns using the formula R_log <- log(1 + R). This is a common choice, especially when working with historical data.
Some common methods for estimating the distribution of portfolio returns include:
- Historical estimation, which uses the empirical distribution over some number of the most recent time periods.
- Normal distribution estimation, which involves estimating parameters from the data and using the appropriate quantile.
- t-distribution estimation, which usually assumes the degrees of freedom rather than estimating them.
- Fitting a univariate GARCH model and simulating ahead.
These methods can be used to estimate the standard deviation, degrees of freedom, or other parameters of the distribution, depending on the chosen approach.
ES Backtest
ES Backtest is a crucial step in validating the accuracy of your Expected Shortfall (ES) values. The Risk Management Toolbox provides two functions for this purpose: esbacktest and esbacktestbysim.
The esbacktest function is a flexible tool that doesn't require information about the portfolio return distribution, but it's relatively limited in terms of accuracy. It has two tests: the unconditional normal and t tests.
For more accurate results, use esbacktestbysim, which runs a comprehensive suite of tests on the ES data. This function takes the same inputs as esbacktest, along with distribution information, currently only supporting normal and t distributions.
The computed ES values may not match the actual portfolio performance, suggesting inadequate distribution assumptions. This was also observed in the "Estimate VaR for Equity Portfolio Using Parametric Methods" example, where normal distribution assumptions led to inaccurate results.
Two possible alternatives to improve the accuracy of ES backtesting are:
- Model the portfolio returns with a t-distribution, which results in fatter tails than the normal distribution.
- Use a nonparametric method to calculate the portfolio VaR and ES by using a historical VaR method.
Frequently Asked Questions
What is the difference between VaR and ETL?
VaR focuses on potential losses up to a specified threshold, while ETL assesses average losses in extreme scenarios beyond that threshold, providing a more comprehensive risk evaluation. This key difference makes ETL a more robust tool for managing risk in uncertain markets.
Sources
- https://en.wikipedia.org/wiki/Expected_shortfall
- https://mayerkrebs.com/what-is-expected-shortfall-cvar/
- https://www.portfolioprobe.com/2012/10/23/the-basics-of-value-at-risk-and-expected-shortfall/
- https://www.mathworks.com/help/risk/expected-shortfall-estimates-for-asset-portfolios.html
- https://www.r-bloggers.com/2012/11/the-estimation-of-value-at-risk-and-expected-shortfall/
Featured Images: pexels.com