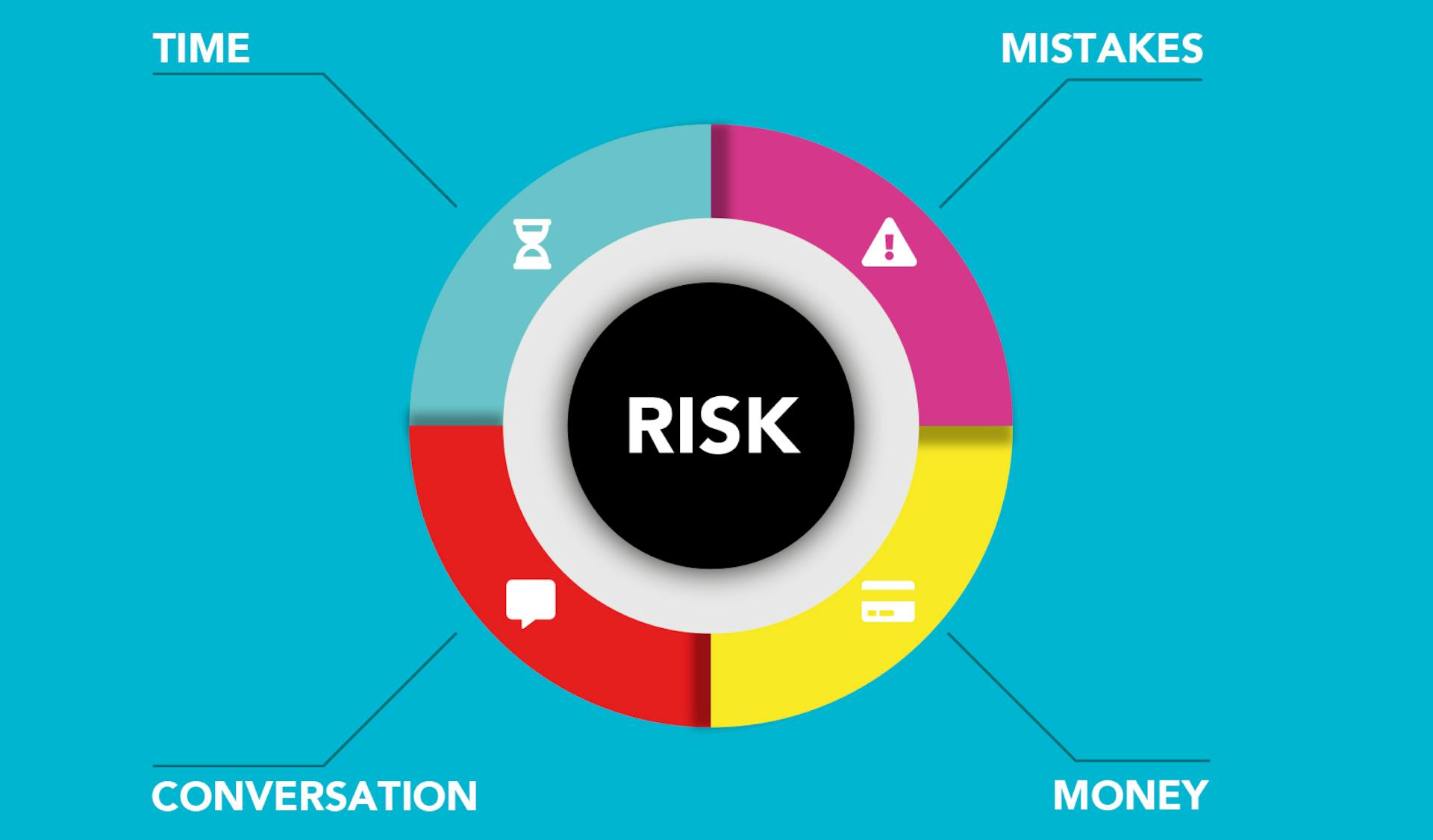
Expected Shortfall in Finance and Risk Assessment is a critical concept that helps institutions manage their risk exposure. It's a measure of the potential loss in a portfolio over a specific time horizon, with a given probability of occurrence.
Expected shortfall is closely related to Value-at-Risk (VaR), which estimates the maximum potential loss in a portfolio over a specific time horizon with a given confidence level. However, expected shortfall provides a more accurate picture of potential losses by considering the entire distribution of losses, not just the maximum loss.
In finance, expected shortfall is used to estimate the potential loss in a portfolio under stress conditions, such as a market crash or a sudden decline in asset values. This information is essential for risk managers to make informed decisions about their investment strategies and risk mitigation techniques.
Expected shortfall can be calculated using various methods, including the Conditional Tail Expectation (CTE) approach, which is based on the assumption that the distribution of losses follows a specific pattern.
Take a look at this: Time Consistency (finance)
Definition and Properties
Expected shortfall is a measure of risk that helps us understand the potential losses of a portfolio. It's defined as the average loss of a portfolio in the worst q% of cases, where q is a given probability level.
The expected shortfall is calculated using the value at risk (VaR) of a portfolio, which is the minimum loss we can expect to incur with a certain probability. The expected shortfall is essentially the average loss of a portfolio when the VaR is exceeded.
One important property of expected shortfall is that it increases as the probability level q decreases. This means that if we're looking at a smaller percentage of worst-case scenarios, the expected loss of a portfolio will be higher.
For a given portfolio, the expected shortfall is always greater than or equal to the VaR at the same probability level. This makes sense, because VaR is a more conservative measure of risk that only considers the minimum loss, while expected shortfall takes into account the average loss in the worst cases.
Readers also liked: Loan Loss Reserve Accounting
Properties
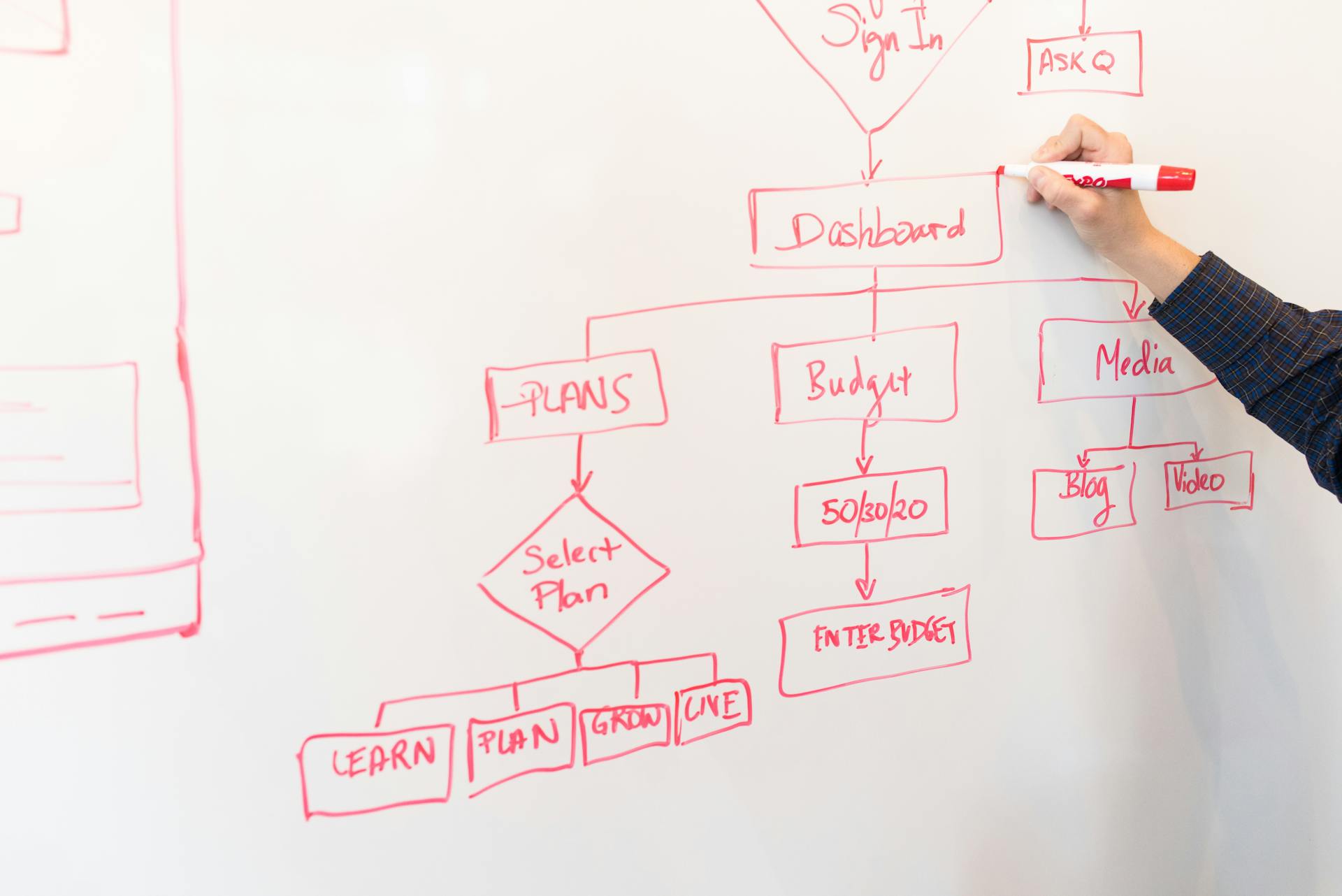
The expected shortfall, or ES, has some interesting properties that are worth noting.
The expected shortfall increases as the confidence level, or q, decreases. This means that as you move down the probability distribution, the expected shortfall grows.
For a given portfolio, the expected shortfall is greater than or equal to the Value at Risk at the same level of q. This is a key relationship between these two risk metrics.
Readers also liked: Us Economy Grew Much Faster than Expected in Second Quarter
Basics of
Value at Risk, or VaR, is defined as the maximum possible loss over a given holding period within a fixed confidence level. It's a way to measure risk, but it has its limitations.
VaR satisfies the first three conditions, but it doesn't always satisfy the fourth. This means it doesn't always meet the expected shortfall criteria, which is a measure of the average loss in extreme scenarios.
VaR has problems measuring extreme price movements, and it can ignore critical information regarding the tails of the underlying distributions. This is because it only measures the distribution quantile, disregarding severe losses beyond the VaR level.
For example, two hedge funds with a one-day 95% VaR of $100 can have very different risk profiles. One fund might have its losses limited to $105, while the other fund has no downside protection and can lose even more than $500.
Explore further: Coherent Risk Measure
Examples and Applications
Expected shortfall is a crucial concept in finance, and understanding it can help you make informed decisions about your investments.
In Example 1, we see that the expected shortfall is EUR 1000 for the 5% tail, which means that the average loss on the worst 5% of possible outcomes for the portfolio is EUR 1000.
To calculate the expected shortfall, you need to determine the worst performing cases. For example, to calculate ES0.05, you need to consider the cases with a profit of −100.
The expected shortfall can be calculated using the expected value formula. In the case of ES0.20, you would select 10 cases from row one and 10 cases from row two, and then calculate the expectation over those cases.
Here are some examples of expected shortfall values for different values of q:
You can see that the expected shortfall decreases as the value of q increases. This makes sense, as you're considering more and more of the possible outcomes.
The 100%-quantile expected shortfall ES1 equals negative of the expected value of the portfolio.
You might like: Expected Shortfall vs Var
Mathematical Framework
In the mathematical framework of expected shortfall, we have specific continuous distributions that allow for closed-form formulas.
These formulas can be used to calculate the expected shortfall when the payoff of a portfolio follows a particular distribution.
Typical values of α in the case of a portfolio are 5% and 1%.
For engineering or actuarial applications, it's more common to consider the distribution of losses, where α values are 95% and 99%.
The expected shortfall in this case corresponds to the right-tail conditional expectation above VaRα(L).
Discover more: Expected Shortfall Formula
Mathematical Framework
The expected shortfall is a measure of risk that takes into account the severity of losses. It's calculated as the average loss given that the loss exceeds the Value at Risk (VaR) by a certain percentage.
The expected shortfall can be written as ESα(X) = E[-X|X ≤ -VaRα(X)], where X is the payoff of a portfolio and VaRα(X) is the Value at Risk. This formula is equivalent to the tail conditional expectation.
For continuous probability distributions, closed-form formulas exist for calculating the expected shortfall. These formulas typically involve the left-tail or right-tail conditional expectation of the distribution.
The expected shortfall is a distortion risk measure given by the distortion function, and it can be written as a function of the Value at Risk (VaR). The typical values of α in this case are 5% and 1%.
In engineering or actuarial applications, it's more common to consider the distribution of losses, where the expected shortfall corresponds to the right-tail conditional expectation above VaRα(L). The typical values of α in this case are 95% and 99%.
Here's an interesting read: Conditional Tail Expectation
Exponential
The exponential distribution is a type of probability distribution that models the time between events in a Poisson process.
In finance, the exponential distribution is often used to model the loss of a portfolio, where the probability density function (p.d.f.) is given by f(x) = λe^(-λx) for x ≥ 0, and 0 otherwise.
The cumulative distribution function (c.d.f.) of the exponential distribution is F(x) = 1 - e^(-λx) for x ≥ 0, and 0 otherwise.
The expected shortfall of a portfolio that follows an exponential distribution can be calculated using the formula ESα(L) = (-ln(1-α) + 1) / λ.
Is Additive?
The concept of additivity is a fundamental one in mathematical frameworks. Yes, Expected Shortfall is indeed additive, satisfying the sub-additivity condition. This means that it can be broken down into smaller parts without changing the overall result.
In practical terms, this makes Expected Shortfall a useful tool for risk analysis and management. It's a property that's essential for many applications, including finance and insurance.
Distributions and Models
ES modeling considers the tail risk, focusing on potential losses beyond a specified confidence level. This is in contrast to traditional risk measures like Value at Risk (VaR).
Two common methods for ES modeling are the historical simulation approach and the Monte Carlo simulation. Historical simulation involves analyzing historical data to estimate potential losses, while Monte Carlo simulation generates numerous random scenarios based on specified probability distributions.
Both approaches can be used to estimate potential losses beyond a certain confidence level, providing a more comprehensive view of extreme events and tail risk.
See what others are reading: Historical Simulation (finance)
Laplace
The Laplace distribution is a type of distribution that can be used to model portfolio payoffs and losses. It's a useful tool for understanding risk and uncertainty.
One important property of the Laplace distribution is that it can be used to calculate the expected shortfall, which is a measure of the average loss in the worst α percent of cases. The expected shortfall formula for the Laplace distribution is quite straightforward: ESα(X)=-μ+b(1−ln2α).
The Laplace distribution is particularly useful for modeling losses, where the payoff is negative. In this case, the expected shortfall formula becomes ESα(L)=-μ+b(1−ln2α).
A unique perspective: How Do You Calculate Solvency Ratio
Logistic
The logistic distribution is a type of distribution that's used to model portfolio payoffs. It's characterized by a probability density function (p.d.f.) that looks like f(x)=1se^(x-μ)/s(1+e^(x-μ)/s)^-2.
In the logistic distribution, the expected shortfall (ES) is a key concept. ES is a measure of the average loss in the worst α percent of cases. For a portfolio X, the expected shortfall is equal to -μ + s ln((1-α)^(1-1/α))/α.
The expected shortfall for a portfolio L following the logistic distribution is a bit more complex, but it's still based on the same underlying principles. It's equal to μ + s(-α ln α - (1-α) ln(1-α))/(1-α).
Generalized Pareto Model (GPM)
The Generalized Pareto Model (GPM) is a crucial tool in risk management, particularly in estimating potential losses beyond a certain confidence level.
The Generalized Pareto distribution (GPD) is a specific type of distribution that can be used to model extreme events. It's often used in Expected Shortfall (ES) modeling, which is a technique for assessing potential losses beyond a specified confidence level.
The GPD has a probability density function (p.d.f.) that allows us to calculate the expected shortfall, which is a key metric in risk management.
Intriguing read: B H P Billiton Share Price
Generalized Hyperbolic Secant (GHS)
The Generalized Hyperbolic Secant (GHS) distribution is a probability distribution used to model portfolio payoffs. It's a type of distribution that can be used to analyze risk and uncertainty.
The GHS distribution has a probability density function (p.d.f.) given by f(x) = 1/2σ sech(π/2(x-μ)/σ), where σ is the scale parameter, μ is the location parameter, and x is the random variable.
The cumulative distribution function (c.d.f.) of the GHS distribution is F(x) = 2/π arctan(exp(π/2(x-μ)/σ)). This function gives the probability that the random variable x takes on a value less than or equal to a given value.
The expected shortfall of the GHS distribution is given by ESα(X) = -μ - 2σ/π ln(tan(πα/2)) - 2σ/π^2α i[L_i2(-i tan(πα/2)) - L_i2(i tan(πα/2))]. This formula can be used to calculate the expected loss of a portfolio given a certain confidence level α.
Take a look at this: Fixed vs Variable Cost
Johnson's SU
Johnson's SU-distribution is a special type of distribution that's used to model portfolio payoffs. It's defined by its cumulative distribution function (c.d.f.) F(x), which involves the standard normal distribution Φ.
The c.d.f. of Johnson's SU-distribution is given by F(x)=Φ[γ+δsinh^(-1)((x-ξ)/λ)]. This formula might look daunting, but it's actually quite straightforward once you understand the components.
Johnson's SU-distribution has several parameters: γ, δ, ξ, and λ. These parameters influence the shape of the distribution and its expected value. The expected shortfall, or ESα, is a key concept in risk management, and it's calculated using the parameters of the distribution.
The formula for expected shortfall is ESα(X)=-ξ-(λ/(2α))[exp((1-2γδ)/(2δ^2))Φ(Φ^(-1)(α)-(1/δ))-exp((1+2γδ)/(2δ^2))Φ(Φ^(-1)(α)+(1/δ))]. This formula looks complicated, but it's essential for understanding the risk associated with a portfolio.
Take a look at this: H B L Power Share Price
Lognormal
The lognormal distribution is a type of distribution that can be used to model portfolio payoffs. If a portfolio's payoff follows a lognormal distribution, the expected shortfall can be calculated using a specific formula.
The formula for expected shortfall under a lognormal distribution is ESα(X) = 1 - exp(μ + σ^2/2) * Φ(Φ^(-1)(α) - σ) / α. This formula is derived from the properties of the lognormal distribution.
The lognormal distribution is characterized by two parameters: μ and σ, which represent the mean and standard deviation of the underlying normal distribution. Understanding these parameters is crucial in modeling portfolio payoffs.
The expected shortfall under a lognormal distribution is a critical concept in risk management, as it provides a measure of the potential loss of a portfolio over a specific time horizon.
Log-Logistic
The log-logistic distribution is a type of distribution that can be used to model portfolio payoffs and losses.
It's defined by the random variable ln(1+X), which follows the logistic distribution with a specific probability density function (p.d.f.).
The expected shortfall of a portfolio following this distribution can be calculated using the formula ESα(X)=1−e^μ/αIα(1+s,1−s)πs/ sin πs.
For a more generic case, the expected shortfall can be expressed using the hypergeometric function: ESα(X)=1−e^μα^s/(s+1)2F1(s,s+1;s+2;α).
The log-logistic distribution can also be used to model losses, with a probability density function f(x)=ba(x/a)^b−1/(1+(x/a)^b)^2.
The expected shortfall of a portfolio following this loss distribution can be calculated using the formula ESα(L)=a/(1−α)[π/bcsc(π/b)−Bα(1/b+1,1−1/b)].
Explore further: How Is Interest Calculated on Credit Cards
Calculation and Estimation
Expected Shortfall (ES) is a risk metric that measures the potential losses beyond a certain threshold. To calculate ES, you need to determine the Value-at-Risk (VaR) level, which represents the threshold beyond which you want to measure the losses.
The VaR level is calculated by finding the value at the specified percentile of the sorted returns. For example, if you want to calculate the ES at a 95% confidence level, you would find the value at the 95th percentile of the sorted returns. This value represents the VaR level.
To calculate the ES, you need to find the average of all the returns that exceed the VaR level. This average is the ES. For instance, if the VaR level is 2% and the average of the returns below -2% is -3%, then the ES at the 95% confidence level is -3%.
ES is widely used in financial risk management, portfolio optimization, and regulatory compliance. It provides a more comprehensive assessment of tail risk compared to traditional risk measures like VaR. By calculating the average of losses beyond a specified VaR level, ES captures the potential magnitude of extreme downside risk.
Take a look at this: Risk Measure
Its Calculation
To calculate Expected Shortfall (ES), you need to determine the Value-at-Risk (VaR) level, which represents the threshold beyond which you want to measure losses. This VaR level is usually specified as a percentile, such as 95% or 99%.
The VaR is calculated by finding the value at the specified percentile of the sorted returns. For example, if you want to calculate the 95% VaR, you would find the value at the 95th percentile of the sorted returns.
Once you have the VaR level, you can calculate the ES by averaging all the returns that exceed the VaR level. This average is the Expected Shortfall. For instance, if the VaR at the 95% confidence level is 2%, you would calculate the average of the returns below -2% to determine the ES.
ES is typically calculated using historical data, and it's essential to consider the choice of loss distribution, as it significantly impacts ES. Commonly used distributions include normal, t-distribution, and empirical distributions. You should also consider the portfolio composition, as diversification can reduce ES, and correlations between assets play a crucial role.
The time horizon used for ES calculation also affects the result, as longer time horizons capture tail risk better but may lead to higher ES values.
For more insights, see: Roth Ira First Time Homebuyer
Estimating Bond Losses with Historical Simulation
Historical simulation is a method used to estimate bond losses by analyzing past data. It involves creating a history of portfolio returns based on the current weights of the portfolio.
You can get the prediction distribution from the portfolio return history in several ways, including fitting an assumed distribution, simulation, GARCH prediction, and GARCH simulation.
Assuming a normal distribution can help estimate the standard deviation, but a t-distribution requires estimating the degrees of freedom. The simulation method uses the empirical distribution of a specific number of portfolio returns.
Using a univariate GARCH model can be an effective way to estimate Value at Risk (VaR) and Expected Shortfall (ES).
Here's a summary of the methods:
The choice of method depends on the characteristics of the bond market and the portfolio. It's essential to consider the limitations of each method and adapt them to the specific needs of the portfolio.
Frequently Asked Questions
What is the difference between VaR and expected shortfall?
VaR measures the maximum potential loss with a certain confidence level, while Expected Shortfall calculates the average loss when VaR is exceeded. This difference highlights the limitations of VaR and the importance of considering extreme losses in risk management.
What is the 95 expected shortfall?
Expected shortfall at 95% confidence is the average of the worst 5% of portfolio returns. It measures the potential loss beyond the 95% Value-at-Risk threshold.
Sources
- https://en.wikipedia.org/wiki/Expected_shortfall
- https://fastercapital.com/topics/defining-expected-shortfall-and-its-calculation.html
- https://www.portfolioprobe.com/2012/10/23/the-basics-of-value-at-risk-and-expected-shortfall/
- https://www.investopedia.com/terms/c/conditional_value_at_risk.asp
- https://mayerkrebs.com/what-is-expected-shortfall-cvar/
Featured Images: pexels.com