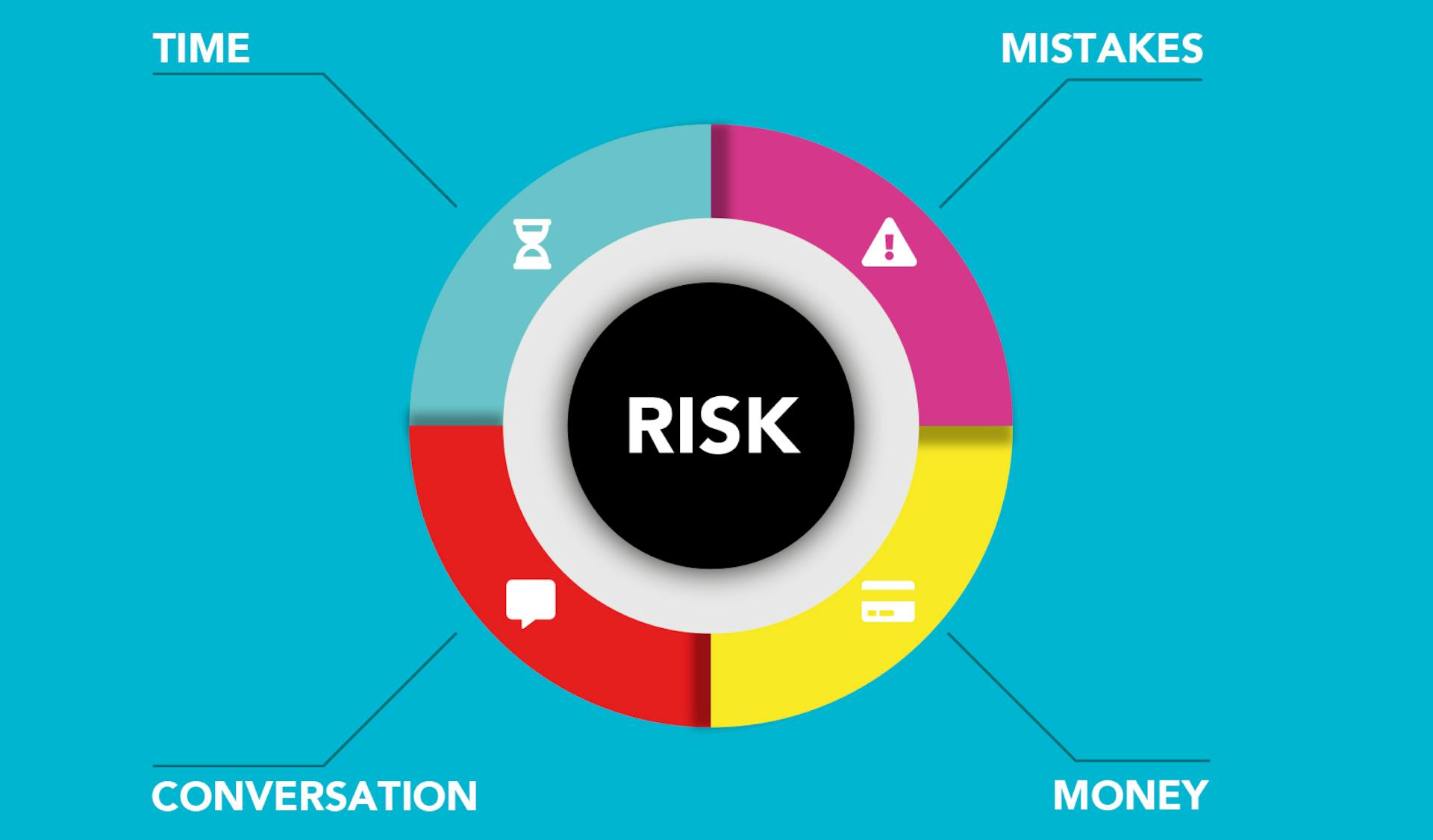
Historical simulation is a widely used method for calculating Value-at-Risk (VaR) in finance. This method involves analyzing historical data to estimate the potential loss of a portfolio over a specific time horizon.
Historical simulation is particularly useful for calculating VaR because it allows for the consideration of extreme events that may not be captured by other methods. By analyzing historical data, financial institutions can gain a better understanding of the potential risks associated with their portfolios.
The accuracy of historical simulation depends on the quality and quantity of the historical data used. A large and representative dataset is essential for generating reliable VaR estimates.
See what others are reading: Historical Sites
Methodology
The Historical Simulation Method is a widely used approach for calculating Value at Risk (VaR). It involves collecting historical data for the assets in the portfolio, calculating returns, sorting them in ascending order, and determining the VaR level by selecting the return at the desired percentile.
To calculate VaR using the Historical Simulation method, you need to follow these steps: collect historical data, calculate returns, sort returns, and determine the VaR level.
See what others are reading: The Dhandho Investor the Low-risk Value Method to High Returns
The Historical Simulation approach assumes that the set of possible future outcomes is fully represented by what occurred in a definite historical time frame/window. This approach is most frequently used by organisations and is particularly useful when you have data on all risk factors over a justified historical period.
Here are the key steps in the Historical Simulation Method:
- Collect Historical Data
- Calculate Returns
- Sort Returns
- Determine the VaR Level
Simulation Method for Calculating
Simulation Method for Calculating VaR can be achieved through the Historical Simulation method, which is one of the most commonly used methods for calculating VaR.
This method involves collecting historical data for the assets in the portfolio, calculating returns from the historical data, sorting returns in ascending order, and determining the VaR level by selecting the return at the desired percentile.
To calculate VaR using the Historical Simulation method, you need to collect historical data for a sufficiently long period to capture different market conditions. For example, the article mentions collecting data for 265 days.
Recommended read: Bhp Billiton Stock Symbol
The returns are then sorted in ascending order, and the VaR level is determined by selecting the return at the desired percentile. For instance, if you're calculating a 1-day 5% VaR, you would select the return at the 5th percentile.
Here's a step-by-step guide to the Historical Simulation method:
- Collect historical data for the assets in the portfolio.
- Calculate returns from the historical data.
- Sort returns in ascending order.
- Determine the VaR level by selecting the return at the desired percentile.
For example, if you're calculating a 1-day 5% VaR, the VaR would be -2% based on the returns in the article.
The VaR at 99% confidence level can be estimated by reading the corresponding value in the series of sorted simulated P&Ls of the portfolio at the desired confidence level and then taking it away from the mean of the series of simulated P&Ls. This can be formulated as follows:
VaR (1 - α) = μ(R) - R α
Where:
VaR (1 - α) is the estimated VaR at the confidence level 100 × (1 - α)%
μ(R) is the mean of the series of simulated returns or P&Ls of the portfolio
R α is the worst return of the series of simulated P&Ls of the portfolio
For another approach, see: Mint Money Manager
What Sets Them Apart
Historical Simulation approach assumes that the set of possible future outcomes is fully represented by what occurred in a definite historical time frame/window.
This approach places more emphasis on recent observations, but still considers daily changes in past/historical values to compute the likelihood of the variations in values of the current portfolio between a given time frame.
Model-building approach involves assumptions about the joint probability distributions of the returns on the market variables, which makes it easy to calculate variance.
However, this approach assumes that daily returns on the investments are normally distributed, which can be its biggest drawback.
The Model Building approach is also more complex to use when a portfolio comprises of nonlinear products such as options.
Historical Simulation approach determines the joint probability distribution of the market variables by historical data, which doesn't involve any estimation of variances or covariance’s.
This approach may be a bit slow for computation, but it's a good choice when you have data on all risk factors over a justified historical period.
Readers also liked: H B L Power Share Price
Data and Portfolio
The data used for historical simulation in finance is crucial for accurate results. The article mentions a dataset of 2665 observations of daily closing values of global equity indices spanning from April 27, 1993, to July 14, 2003.
This dataset is used to examine the daily closings of the global equity index data. The initial level of each index has been normalized to unity to facilitate comparison of relative performance.
A portfolio weight vector is specified in preparation for subsequent modeling. An equally weighted portfolio is assumed, but you can change the weight vector to examine any other portfolio composition or even a single country.
The portfolio weights are fixed throughout the risk horizon, and the simulation ignores any transaction costs required to rebalance the portfolio.
Advantages and Limitations
Historical simulation is a method for calculating VaR that has its advantages and limitations. It's a simple and easy to understand approach that doesn't assume any particular distribution for the returns.
However, it's not without its flaws. One major limitation is that it assumes the future will be like the past, which may not always be the case.
This method also has a hard time being accurate if the historical data doesn't include periods of stress.
Here are some key advantages and limitations of historical simulation:
- Simple and easy to understand
- Does not assume any particular distribution for the returns
- Captures the actual historical relationships between different assets
- Assumes the future will be like the past, which may not always be the case
- May not be accurate if the historical data doesn't include periods of stress
- Does not take into account the changes in the portfolio composition over time
Advantages and Disadvantages
The historical simulation method for calculating VaR has its advantages and disadvantages. This method is simple and easy to understand, making it a great choice for many users.
One of the biggest advantages is that it doesn't assume any particular distribution for the returns, giving it a lot of flexibility. It also captures the actual historical relationships between different assets, which can be very useful for risk management.
However, there are also some significant disadvantages to consider. The method assumes that the future will be like the past, which may not always be the case, and can lead to inaccurate results.
A fresh viewpoint: Second Mortgages Advantages and Disadvantages
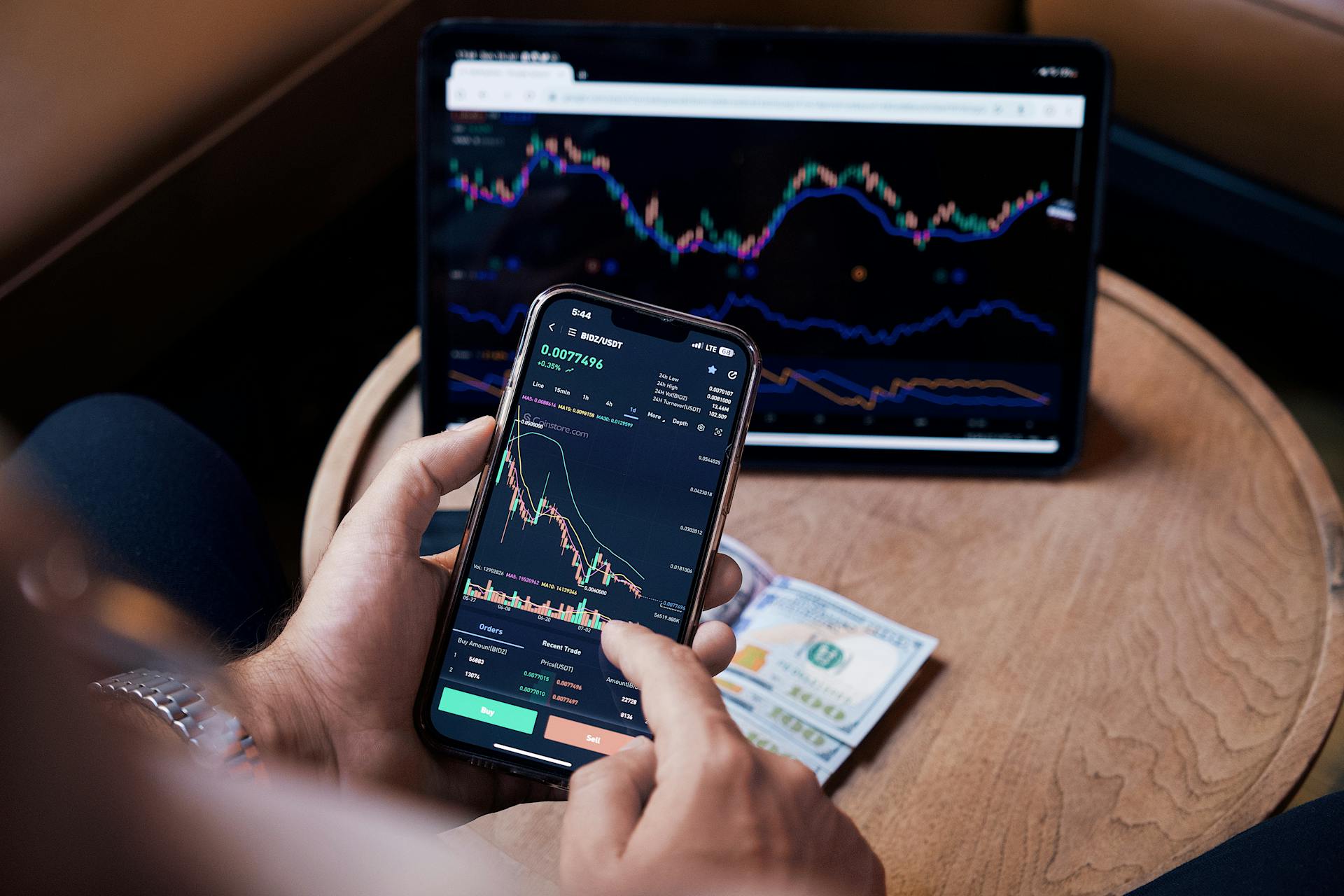
Here are some of the main advantages and disadvantages of the historical simulation method:
- Simple and easy to understand
- Does not assume any particular distribution for the returns
- Captures the actual historical relationships between different assets
- Assumes that the future will be like the past
- May not be accurate if the historical data does not include periods of stress
- Does not take into account the changes in the portfolio composition over time
Problems with VaR
The concept of Value-at-Risk (VaR) has its limitations, and one of the biggest problems is "ghost effects", where big spikes in volatility or price shocks have an outsize effect on VaR much later down the road.
This issue is a result of the way VaR methods work, and it's not entirely avoidable, even with more advanced methods.
Dowd's textbook lists an exhaustive list of pros and cons, but the "ghost effects" issue is a significant one that needs to be addressed.
Our more advanced methods try to correct for this, but they can't escape it entirely, which means VaR may not always be a reliable measure of risk.
A fresh viewpoint: What Is Tier One Credit
Related Topics
Historical simulation is a powerful tool for managing financial risk. It involves generating scenarios based on historical data to estimate potential losses.
The process of historical simulation can be complex, but it's essential for understanding the risks associated with financial instruments. Banks use historical simulation to estimate potential losses, as seen in the example where 500 scenarios were generated to rank losses from highest to lowest.
In practice, historical simulation is used to calculate key risk metrics, such as Value at Risk (VaR) and Expected Shortfall (ES). VaR is a measure of potential loss, and ES is a measure of potential loss beyond VaR.
VaR and ES are critical metrics for financial institutions. For example, a 99% VaR of £50,000 means that there is a 1% chance of losses exceeding £50,000. ES provides a more comprehensive view of potential losses, as it takes into account the severity of losses beyond VaR.
ABS CDOs, or asset-backed securities collateralized debt obligations, are complex financial instruments that involve multiple tranches. These tranches can have different risk profiles, making it challenging to compare them to traditional bonds.
In fact, the risk of an AAA-rated ABS CDO tranche is not directly comparable to a AAA-rated bond, as seen in the example where a £1 million tranche is discussed. The risk of an ABS CDO tranche depends on the underlying assets and the structure of the instrument.
Default rates and recovery rates are critical factors in understanding the risks associated with financial instruments. A bad year for defaults is doubly bad for a lender because it is usually accompanied by a low recovery rate, as discussed in the article.
Note: The table above highlights key metrics and concepts discussed in the article.
Frequently Asked Questions
What is an example of a historical simulation?
A historical simulation example is a 95% confidence level that 95% of monthly returns will exceed a -10% loss, based on past results. This method uses historical data to estimate future performance with a high degree of accuracy.
What is the simulation model in finance?
Simulation models in finance use historical data and market trends to generate possible future scenarios, helping traders and investors make informed decisions. This analytical tool predicts market behavior, enabling data-driven investment choices.
What is the difference between model building and historical simulation?
Historical simulation relies on past data, assuming future outcomes will mirror the past, whereas model-building approach uses probability distributions to forecast market returns. This fundamental difference in approach affects how each method predicts future market behavior.
Sources
- https://medium.com/@matt_84072/historical-simulation-value-at-risk-explained-with-python-code-a904d848d146
- https://www.mathworks.com/help/econ/using-bootstrapping-and-filtered-historical-simulation-to-evaluate-market-risk.html
- https://www.studocu.com/en-gb/messages/question/7308272/explain-the-historical-simulation-method-for-calculating-var
- https://financetrain.com/calculating-var-using-historical-simulation
- https://imarticus.org/blog/model-building-approach-historical-simulation-things-consider/
Featured Images: pexels.com