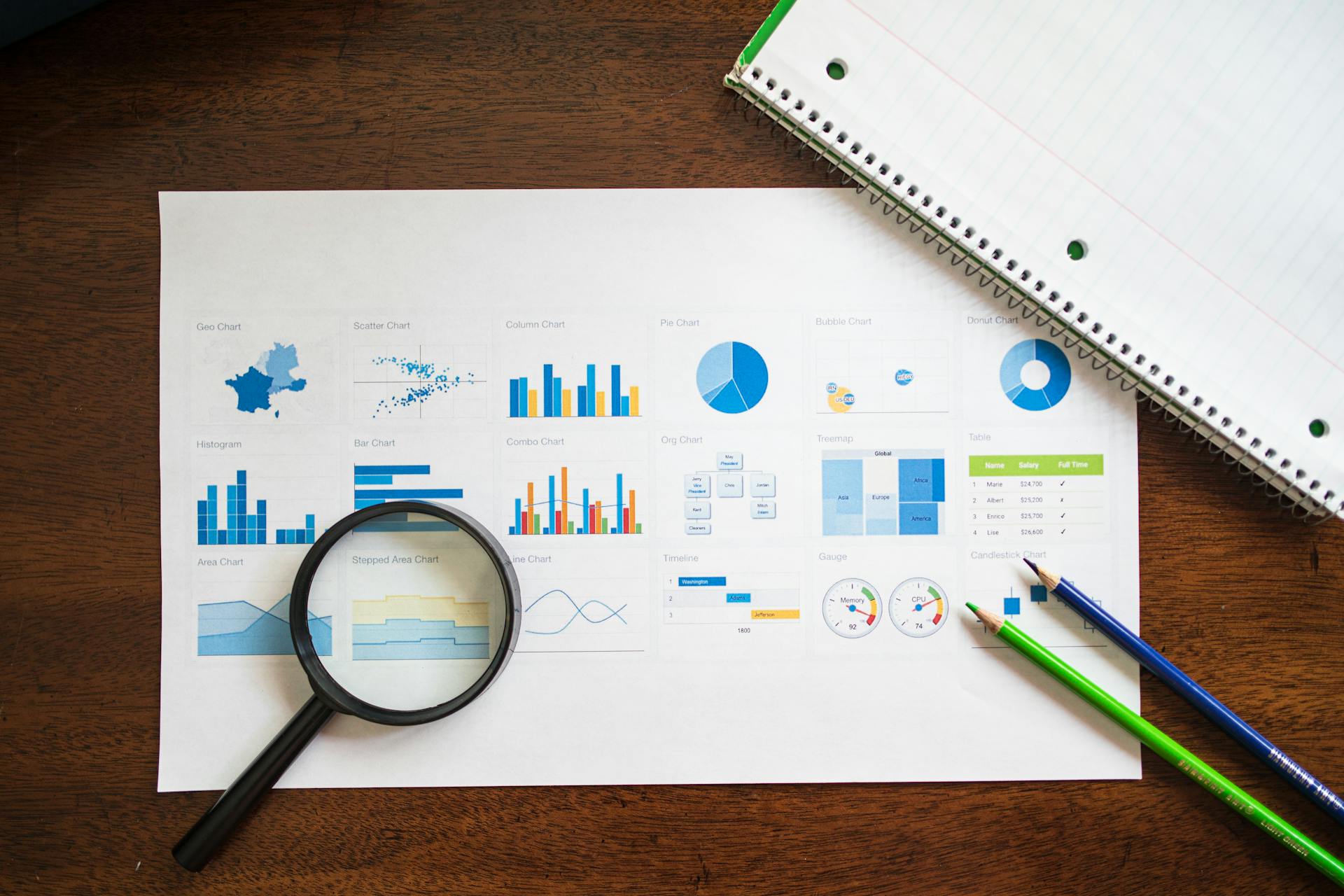
The Spectral Risk Measure is a powerful tool for evaluating the risk of a portfolio or investment. It's a type of risk measure that takes into account the tail risk of a distribution.
In simple terms, the Spectral Risk Measure is a way to quantify the risk of a loss. It's calculated using a formula that incorporates the probability of a loss and the magnitude of that loss.
The Spectral Risk Measure is particularly useful for investors who want to understand the risk of their portfolio, especially in times of market volatility.
Check this out: Sports Related Risk
What is Spectral Risk Measure?
A spectral risk measure is a risk measure that calculates a weighted average of outcomes based on a user's attitude towards risk. It's a function that measures portfolio returns and the number of outputs of the numeraire to be kept in reserve.
This type of risk measure is always coherent, but not all coherent risk measures are spectral risk measures. Spectral risk measures have an advantage in that they possess an accord with risk aversion, particularly to a utility function, by means of weights given to the possible portfolio returns to the expected possible returns.
On a similar theme: Angle Measures
Spectral risk measures are considered one of the most potential and interesting developments in financial risk areas, sharing common properties with coherent risk measures. They possess sub-additivity, which means that individual risks typically diversify when all the risky positions are put together.
A key characteristic of spectral risk measures is that they enable us to relate the risk measure to a user's perception and sensitivity towards risk. If a user is more risk-averse, other things being equal, then that user should face a higher risk, as given by the value of the spectral risk measure.
Spectral risk measures can be defined mathematically as a weighted average of the quantiles, q, of the loss distribution, where the weights depend on the user's risk aversion. This is represented by the formula: Mphi = ∫0^1 phi(p) qp dp, where phi(p) is the weighting function that reflects the user's risk aversion.
For another approach, see: Spectral Sea Emblem
Types of Spectral Risk Measures
Spectral risk measures are used to assess and manage risk in the finance industry. They're a way to quantify the potential losses of a portfolio or investment.
There are several types of spectral risk measures, each with its own characteristics and applications. Expected Shortfall (ES) is one such measure, which can be viewed as a spectral measure by representing the mean of the worst (1-α)% losses.
Exponential Spectral Risk Measures emphasize higher losses more heavily as the confidence level α increases. This makes them suitable for highly risk-averse investors. The function φ(α) = k * exp(kα) is used to define these measures, where k > 0.
Power Spectral Risk Measures, on the other hand, emphasize higher quantiles more gradually depending on the power γ. This makes them suitable for different risk aversion levels. The function φ(α) = k * α ^ (γ - 1) is used to define these measures, where γ > 1 and k is a normalizing constant.
Properties
Spectral risk measures inherit several properties from coherent risk measures, making them robust and appealing for practical applications.
One of the key properties is monotonicity, which means that if the loss from one portfolio is always worse than another, the risk measure will reflect higher risk for the worse-performing portfolio.
Spectral risk measures also exhibit subadditivity, which suggests that individual risks typically diversify (or, at worst, stop increasing) when all the risky positions are put together.
This property is crucial in promoting diversification, as it ensures that the combined portfolio risk will not exceed the sum of individual risks.
Translation invariance is another property of spectral risk measures, which means that adding a certain amount to all outcomes increases the risk measure by that amount, allowing for clear adjustments.
This property is useful in making adjustments to the risk measure, ensuring that it accurately reflects the changes in the portfolio.
Additional reading: Risk Management Principle
Positive homogeneity is also a property of spectral risk measures, which means that scaling all losses by a positive factor scales the risk measure by the same factor, ensuring proportionality.
This property ensures that the risk measure is consistent and proportional to the changes in the portfolio.
Here are the properties of spectral risk measures in a concise list:
- Monotonicity: Higher risk for worse-performing portfolios
- Subadditivity: Combined portfolio risk does not exceed individual risks
- Translation Invariance: Clear adjustments to risk measure
- Positive Homogeneity: Proportional to changes in portfolio
Common
Spectral risk measures are a way to quantify risk in finance, and several specific measures are commonly discussed and applied.
One of the most well-known spectral risk measures is Expected Shortfall, which can be viewed as a spectral measure that represents the mean of the worst (1-α)% losses.
Expected Shortfall is not strictly a spectral measure, but it can be derived from a spectral measure if the function φ(α) is concentrated at the tail.
Spectral risk measures can be defined in different ways, with some emphasizing higher losses more heavily than others.
Exponential Spectral Risk Measures are one type, where φ(α) = k * exp(kα), with k > 0. This emphasizes higher losses more heavily as α increases, making it suitable for highly risk-averse investors.
Another type is Power Spectral Risk Measures, where φ(α) = k * α ^ (γ - 1) for some parameter γ > 1 and normalizing constant k. This emphasizes higher quantiles more gradually depending on the power γ.
These measures can be used to assess risk in different financial scenarios, and they offer a way to quantify risk that's more nuanced than traditional measures.
Here are some key characteristics of these measures:
These measures are not mutually exclusive, and they can be used in conjunction with each other to get a more complete picture of risk.
Applications
Spectral risk measures have a wide range of applications in finance, particularly in algorithmic trading.
Their flexibility makes them a great fit for this field, allowing for a tailored approach to risk management.
Algorithmic trading relies on complex algorithms to execute trades, and spectral risk measures can help fine-tune these algorithms to perform well under realistic market conditions.
This is achieved by providing a comprehensive risk assessment that aligns with the risk appetite of stakeholders.
In portfolio construction, spectral risk measures can help balance returns and risk according to an investor's or fund manager's risk tolerance.
By integrating these measures into optimization algorithms, traders can develop portfolios that are efficient in terms of returns and tailored to specific risk preferences.
Spectral risk measures are a valuable tool for traders looking to optimize their portfolios and manage risk effectively.
Real-World Examples and Case Studies
Several financial institutions and hedge funds have adopted spectral risk measures to enhance their risk management practices and optimize trading strategies. Companies like MSCI, which provides risk management tools, have integrated complex risk measures into their product offerings.
Axioma, known for its advanced risk modeling and portfolio construction solutions, is another example of a company that has adopted spectral risk measures.
By adopting spectral risk measures, these firms can offer more personalized and accurate risk assessments, boosting investor confidence and promoting more sophisticated trading strategies.
Algorithm Calibration
Algorithm calibration is crucial for trading algorithms to perform well in realistic market conditions. This is where spectral risk measures come in, providing a comprehensive risk assessment that aligns with stakeholders' risk appetite.
Spectral risk measures help fine-tune algorithm parameters by offering a clear picture of potential losses. They give traders a better understanding of the risks involved.
To ensure algorithms perform well, parameters need to be calibrated. Spectral risk measures make this process more efficient by providing a risk assessment that's aligned with stakeholders' risk appetite.
Spectral risk measures offer a more nuanced view of risk, taking into account various market conditions. This makes them an essential tool for traders looking to optimize their algorithms.
Tools and Providers
Axioma is a notable provider of risk and portfolio management tools that incorporate advanced risk measures, including spectral risk measures.
Their solutions allow traders and risk managers to tailor their risk assessments to the specific risk aversion profiles of their investors, improving overall decision-making processes.
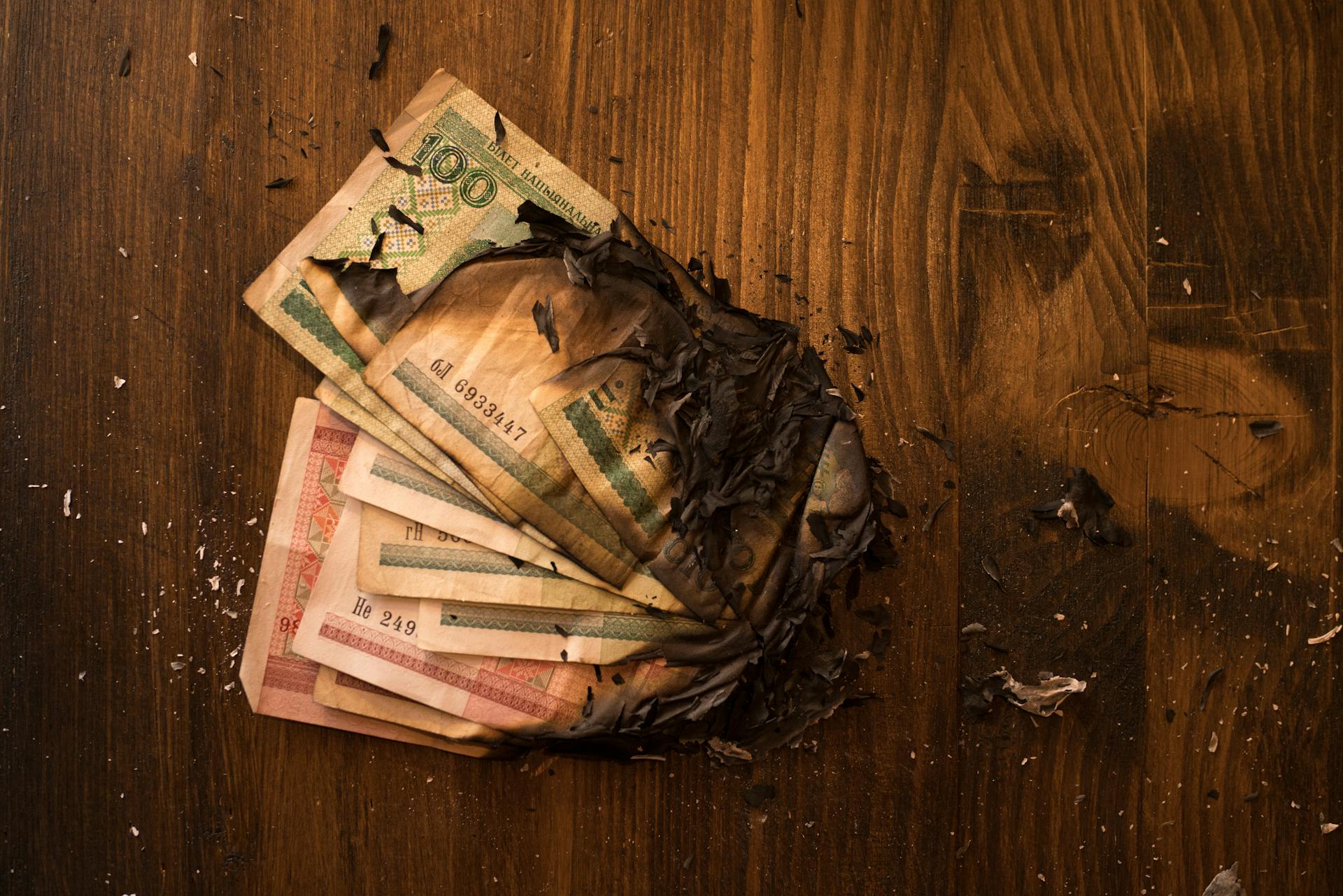
Axioma's tools provide a high degree of precision in risk evaluations, which is particularly useful in volatile markets.
MSCI provides a wide range of tools and analytics for risk management, including the adoption of spectral risk measures.
This allows MSCI clients to achieve a higher degree of precision in their risk evaluations, especially when dealing with complex portfolios in volatile markets.
Spectral risk measures are a valuable addition to any risk management toolkit, and providers like Axioma and MSCI are at the forefront of this technology.
Sources
- https://www.globalcapital.com/article/28mws7f6j02ac2felbs3l/derivatives/beyond-var-expected-shortfall-spectral-risk-measures
- https://algotradinglib.com/en/pedia/s/spectral_risk_measures.html
- https://blogs.cfainstitute.org/investor/2014/02/26/are-spectral-risk-measures-respectable-enough/
- https://link.springer.com/article/10.1007/s10693-008-0035-6
- https://myassignmenthelp.com/finance/spectral-risk-measure.html
Featured Images: pexels.com