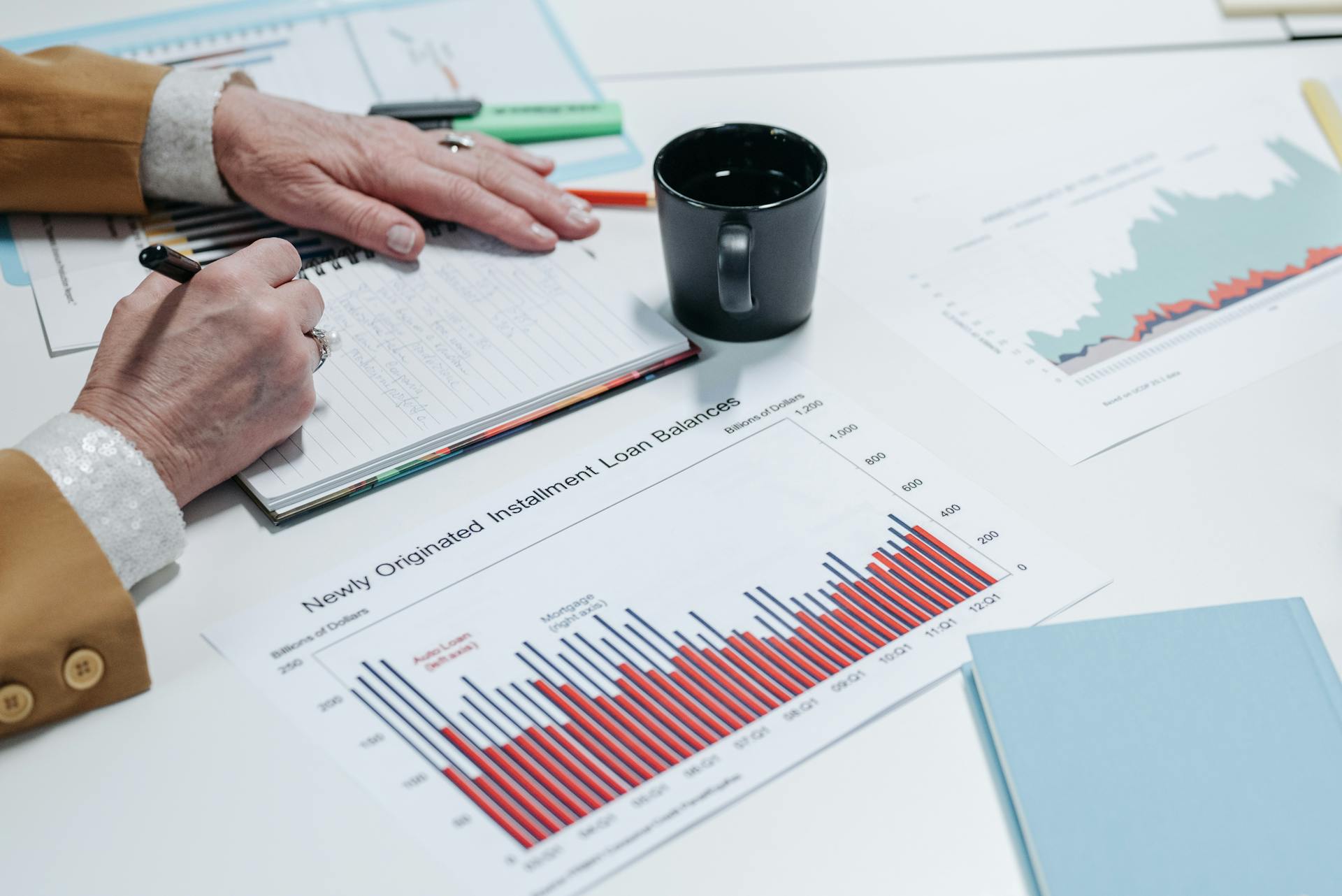
Time consistency in finance is a crucial concept that ensures investors can stick to their long-term plans despite changing circumstances. This means that a financial plan should be able to withstand unexpected events and still achieve its objectives.
A time-inconsistent investor is one who makes decisions based on current circumstances, which can lead to poor long-term outcomes. For instance, an investor might decide to save more in good times and spend more in bad times, but this can lead to inconsistent savings and investments.
The key to time consistency is to create a plan that is robust enough to withstand changing circumstances. This can be achieved by setting clear financial goals and sticking to a well-diversified investment portfolio.
Worth a look: The Role of AI in Revolutionizing the Financial Industry
Literature Review
Time consistency in finance refers to the ability of an individual or organization to stick to a plan over time. This concept is crucial in personal finance and investment decisions.
Research has shown that people tend to be overly optimistic about their future behavior, which can lead to inconsistent decision-making. This phenomenon is known as the "planning fallacy."
The planning fallacy is a cognitive bias that causes individuals to underestimate the time and resources required to complete a task. This bias can lead to poor financial decisions, such as overspending or under-saving.
Studies have found that people are more likely to stick to a plan if they have a clear understanding of their goals and values. This is why setting specific, measurable, and achievable goals is essential for time consistency.
The concept of time consistency is closely related to the idea of hyperbolic discounting, which refers to the tendency to value immediate rewards more highly than future rewards. This can lead to impulsive decisions that compromise long-term goals.
Incorporating time consistency into financial decision-making can lead to better outcomes and increased financial stability. By recognizing and addressing cognitive biases, individuals can make more informed choices that align with their long-term goals.
Here's an interesting read: Exploring Credit Inquiry Solutions for Smarter Financial Decisions
Mathematical Preliminaries
In finance, time consistency is a crucial concept that ensures the stability of risk management strategies over time. This concept is closely related to the mathematical framework of filtered probability spaces.
A filtered probability space is a mathematical construct that allows us to model uncertainty and randomness in financial markets. It consists of a set of possible outcomes, a set of information available at each time step, and a probability measure that assigns a likelihood to each outcome.
The filtered probability space is denoted as (Ω, F, F = {Ft}t∈T, P), where Ω is the set of possible outcomes, F is the set of information available at each time step, and P is the probability measure.
In this framework, the set of all random variables that are measurable with respect to the information available at time t is denoted as Lt. The set of all random variables that are measurable with respect to the information available at time t and have values in the interval [−∞, ∞] is denoted as L0t.
The set of all probability measures on (Ω, F) that are absolutely continuous with respect to P is denoted as M(P). The set of all probability measures on (Ω, F) that are absolutely continuous with respect to P and agree with P on the information available at time t is denoted as Mt(P).
In this context, a dynamic risk measure is a mathematical object that assigns a risk value to a random variable at each time step. A dynamic risk measure is time consistent if it satisfies a certain condition: if the risk value of a random variable at time t+1 is greater than or equal to the risk value of another random variable at time t+1, then the risk value of the first random variable at time t must be greater than or equal to the risk value of the second random variable at time t.
This condition ensures that the risk management strategy is stable over time and does not lead to unexpected consequences. It is a fundamental requirement for any risk management strategy to be effective and reliable.
You might enjoy: Value of Money
Semi-Weak
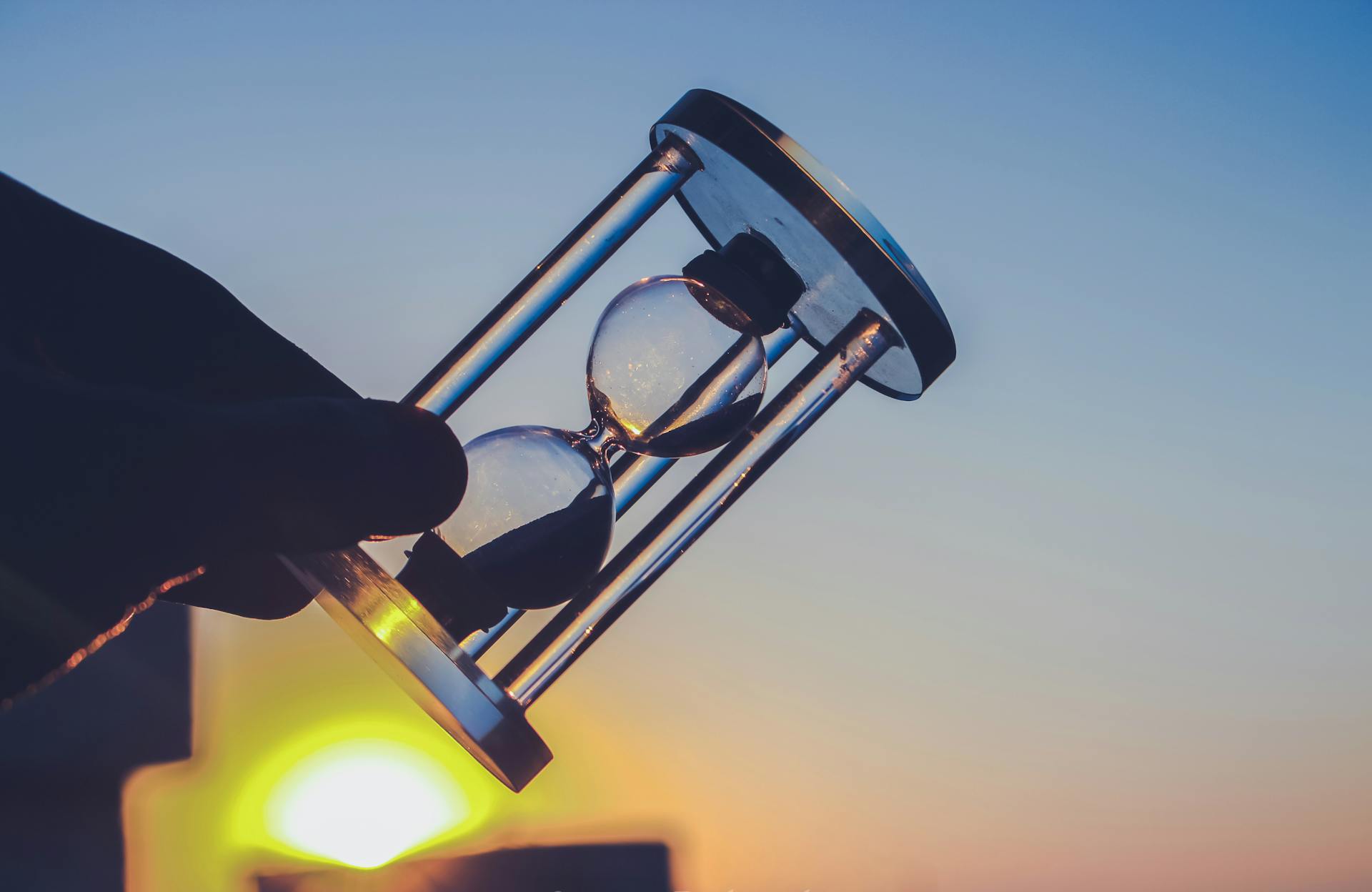
Time consistency in finance is a complex concept, but let's break it down.
Time consistency refers to the consistency of measurements in time, which means that the assessment of preferences remains the same over time.
In the context of dynamic LM-measures, time consistency is studied via numerical representations of preferences. Various numerical representations are surveyed in the literature, and we'll focus on the case of random variables.
The space Lp is used to study risk measures and performance measures, where p ∈ {0, 1, ∞}. This is because a certain topological structure is required for a robust representation of such measures.
Time consistency refers only to consistency of measurements in time, and no particular topological structure is needed. This means that most results obtained hold true for p = 0.
A semi-weak approach to time consistent assessment of preferences is not explicitly mentioned in the article, but we can infer from the context that it might be a type of time consistency that can be represented within the generic approaches outlined in the article.
A different take: Risk Measure
However, it's worth noting that the article does mention projective update rules, which are a type of update rule that is used to assess preferences. It's not clear if this is related to semi-weak time consistency, but it's an interesting area of study nonetheless.
In the article, it's mentioned that there is a dynamic LM-measure that is μ-time consistent but not μ-time consistent. This shows that time consistency can be a nuanced concept, and different measures may have different levels of consistency.
The article also mentions that the approaches outlined in Section "Idiosyncratic approaches" are specific to certain types of time consistency. This suggests that semi-weak time consistency might be a specific type of time consistency that is suited to a particular class of dynamic LM-measures or spaces.
However, without more information, it's difficult to say for sure what semi-weak time consistency entails. More research is needed to fully understand this concept and its implications for finance.
Time Consistency Approaches
Time consistency in finance is a crucial concept that ensures decision-making is consistent over time. There are two main approaches to achieving time consistency: generic and idiosyncratic approaches.
Generic approaches include the update rules and benchmark families, which are used to characterize different types of time consistency. The update rule approach involves updating the preference level at time s to the preference level at time t using an update rule, while the benchmark family approach involves taking the preference levels at both times s and t as φs(Y) and φt(Y), respectively, for any reference object Y.
Time consistency can also be achieved through idiosyncratic approaches, which exploit the unique properties of a specific dynamic LM-measure. For example, dynamic convex or monetary risk measures can be characterized in terms of the relevant properties of associated acceptance sets and/or the dynamics of the penalty functions.
The following table summarizes the main differences between the two approaches:
In conclusion, time consistency is a critical concept in finance that ensures decision-making is consistent over time. By understanding the different approaches to achieving time consistency, we can make more informed decisions and avoid potential pitfalls.
Generic Approaches
Generic approaches to time consistent assessment of preferences can be characterized in terms of two key concepts: update rules and benchmark families.
An update rule is a tool that is applied to preference levels, used for relating assessments of preferences done using a dynamic LM-measure at different times. It's a way to update our preferences over time.
The update rule approach was developed in Bielecki et al. (2014a) and is a key part of understanding time consistency.
The benchmark family approach is another generic approach, where preference levels at different times are taken as φs(Y) and φt(Y) for any reference object Y.
These two approaches are strongly related to each other, and for any LM-measure φ and for any benchmark family Y, one can construct an update rule μ such that φ is time consistent with respect to Y if and only if it is μ-time consistent.
The update rule approach is more general, and time consistency of a dynamic coherent acceptability index cannot be expressed in terms of a single benchmark family. This shows the importance of understanding the different approaches to time consistency.
For another approach, see: Coherent Risk Measure
Idiosyncratic Approaches
Idiosyncratic approaches to time consistency are tailored to specific subclasses of dynamic measures, exploiting their unique properties. These approaches often focus on the characteristics of acceptance sets, penalty functions, and probability measures.
In the case of dynamic convex or monetary risk measures, time consistency can be characterized by the rectangular property of the families of probability measures. This property is crucial for ensuring consistency in decision-making.
Each idiosyncratic approach is suited for a specific subclass of dynamic measures, making them unique and tailored to their needs. For example, automatic payroll deductions for retirement savings can help individuals save consistently over time, serving as a commitment device.
Institutional checks, such as independent central banks or fiscal policy rules, can help mitigate the effects of time-inconsistent policies by removing short-term political pressures from critical economic decisions.
Commitment and Optimal Policy
Time inconsistency can lead to suboptimal decision-making and welfare loss. For individuals, it can result in poor financial planning, inadequate savings, and failure to invest in long-term health and education. For policymakers, it can result in economic instability, inefficient taxation, and suboptimal public spending.
Commitment devices, such as automatic payroll deductions for retirement savings, can help individuals save consistently over time. Institutional checks, like independent central banks or fiscal policy rules, can help mitigate the effects of time-inconsistent policies by removing short-term political pressures from critical economic decisions.
Understanding time inconsistency helps in designing mechanisms to ensure commitment to long-term goals. Time-consistent risk measures, such as the dynamic superhedging price, can provide a framework for making optimal policy decisions.
Here are some common commitment devices:
- Automatic payroll deductions for retirement savings
- Independent central banks or fiscal policy rules
- Contractual agreements with penalties for non-compliance
These devices can help individuals and policymakers make decisions that align with their long-term goals, rather than being swayed by short-term interests. By understanding time inconsistency and using commitment devices, we can make more informed decisions and achieve better outcomes.
Take a look at this: Short Trading Term Definitions
Update Rules
Update rules are a tool that can be applied to preference levels to relate assessments of preferences done using a dynamic LM-measure at different times.
The approach to time consistency using update rules was developed in Bielecki et al. (2014a), providing a way to make assessments of preferences more consistent over time.
An update rule is applied to preference levels, and it's used to update the preference level at time s to the preference level at time t.
The update rule approach and the benchmark family approach differ in how they choose preference levels. In the update rule approach, the preference level at time s is chosen as any ms ∈ ℒ0s, and then updated to the preference level at time t, using an update rule.
For any LM-measure φ and for any benchmark family Y, one can construct an update rule μ such that φ is time consistent with respect to Y if and only if it is μ-time consistent.
The locality of φ makes it easy to note that a certain equation is equivalent to another one, which involves the update rule μ.
Setting a specific equation involving the update rule μ and using another equation, we deduce that φ satisfies the first equation if and only if φ is time consistent with respect to the update rule μt,s.
For another approach, see: Wash Sale Rule
Robust Expectations and Martingales
Time consistency in finance is a crucial concept that ensures the stability of financial decisions over time. It's connected to the concept of robust expectations, which are a type of expectation that can handle uncertainty.
Robust expectations are generated by conditional expectations and determining families of sets. A determining family of sets is a collection of sets that satisfy certain properties, including being non-empty, closed, and convex.
In the context of dynamic risk measures, robust expectations are used to determine the minimal penalty functions, which are essential for time consistency. These minimal penalty functions are used to calculate the penalty for a given probability measure.
Here's a breakdown of the key components of robust expectations and martingales:
Robust expectations are closely related to supermartingales and submartingales, which are types of stochastic processes that satisfy certain properties. A supermartingale is a stochastic process that is non-negative and has a certain property called "supermartingale property".
In summary, robust expectations are a crucial concept in finance that ensures the stability of financial decisions over time. They are generated by conditional expectations and determining families of sets, and are used to determine the minimal penalty functions, which are essential for time consistency.
Proofs and Examples
Time consistency is a fundamental concept in finance, and understanding its proofs and examples is crucial for making informed decisions. One way to prove time consistency is to show that a given map φ satisfies certain properties, such as locality and monotonicity.
In particular, if φ satisfies property (10), which states that there exists a Z in X such that φs(Z) = 0, then φ is time consistent. This is because φ can be extended to a map ϕt,s that is local and monotone on Xφs.
The map ϕt,s is defined as follows: for any X' in Xφs, ϕt,s(X') = φt(X), where X is the unique element in X such that X = φs(X). This map is well-defined due to the strong time consistency of φ.
Here's a summary of the key properties of ϕt,s:
- Locality: ϕt,s is local on Xφs, meaning that for any A in Ft, ϕt,s(X) ≥ ϕt,s(Y) if (AX) ≥ (AY).
- Monotonicity: ϕt,s is monotone on Xφs, meaning that for any X, Y in Xφs, ϕt,s(X) ≥ ϕt,s(Y) if X ≥ Y.
These properties are crucial for proving time consistency, and they have important implications for financial modeling and decision-making.
Corollary 1
Corollary 1 is a game-changer in the world of stochastic processes. It states that if we have a dynamic LM-measure φ on \(\mathbb {V}^{p}\), then the family of maps \(\widetilde {\varphi }_{t}: L_{t+1}^{p}\to \bar {L}^{0}_{t}\) given by \(\widetilde {\varphi }_{t}(x) = \varphi _{t}(x)\) is a strongly time consistent dynamic LM-measure on \(\mathbb {V}^{\infty }\).
This means that φ is a strongly time consistent dynamic LM-measure on \(\mathbb {V}^{\infty }\) if and only if it satisfies certain properties, such as being monotone and local.
In fact, φ is monotone and local on \(\mathbb {V}^{p}\), which implies that \(\widetilde {\varphi }_{t}\) is local and monotone on \(L_{t+1}^{p}\).
Here's a quick summary of the key points:
- φ is a dynamic LM-measure on \(\mathbb {V}^{p}\)
- \(\widetilde {\varphi }_{t}\) is a strongly time consistent dynamic LM-measure on \(\mathbb {V}^{\infty }\)
- \(\widetilde {\varphi }_{t}\) is local and monotone on \(L_{t+1}^{p}\)
This corollary is a crucial building block for understanding time consistency in stochastic processes, and it has important implications for various applications.
Examples
This section presents examples that illustrate the different types of time consistency for dynamic risk measures and dynamic performance measures, as well as the relationships between them.
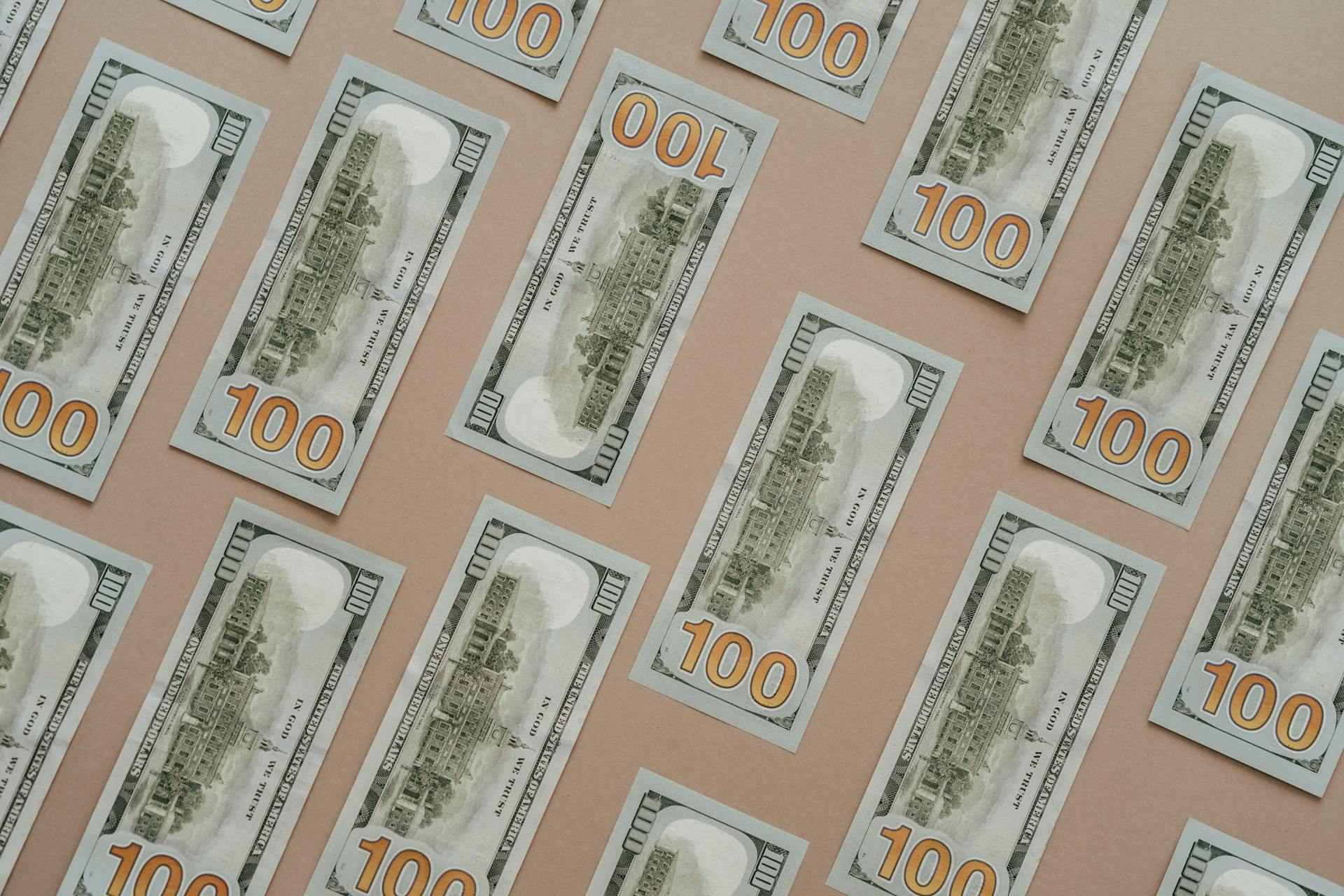
According to the convention adopted in this paper, dynamic LM-measures representing risk measures are the negatives of their classical counterparts.
We'll be skipping the term "negative" in the titles of examples representing risk measures, which will make them easier to understand.
Time consistency is crucial for making informed decisions, and these examples will help illustrate its importance.
Proof of 17
Proof of 17 is a fascinating topic that delves into the properties of a dynamic LM-measure. Specifically, it explores the adaptivity, monotonicity, locality, and extension of a dynamic LM-measure.
The proof of 17 is built upon the concept of an extension of a dynamic LM-measure. An extension is a dynamic LM-measure that satisfies certain properties, such as monotonicity and locality. In the context of proof 17, the extension is denoted as φ.
Adaptivity is a key property of an extension. It states that for any X in the set of random variables and A in the filtration, φ+ (X) is equal to the essential infimum of the set of φt (Y) for all Y in the set of random variables that are greater than or equal to X and belong to the set A.
Broaden your view: Spectral Risk Measure
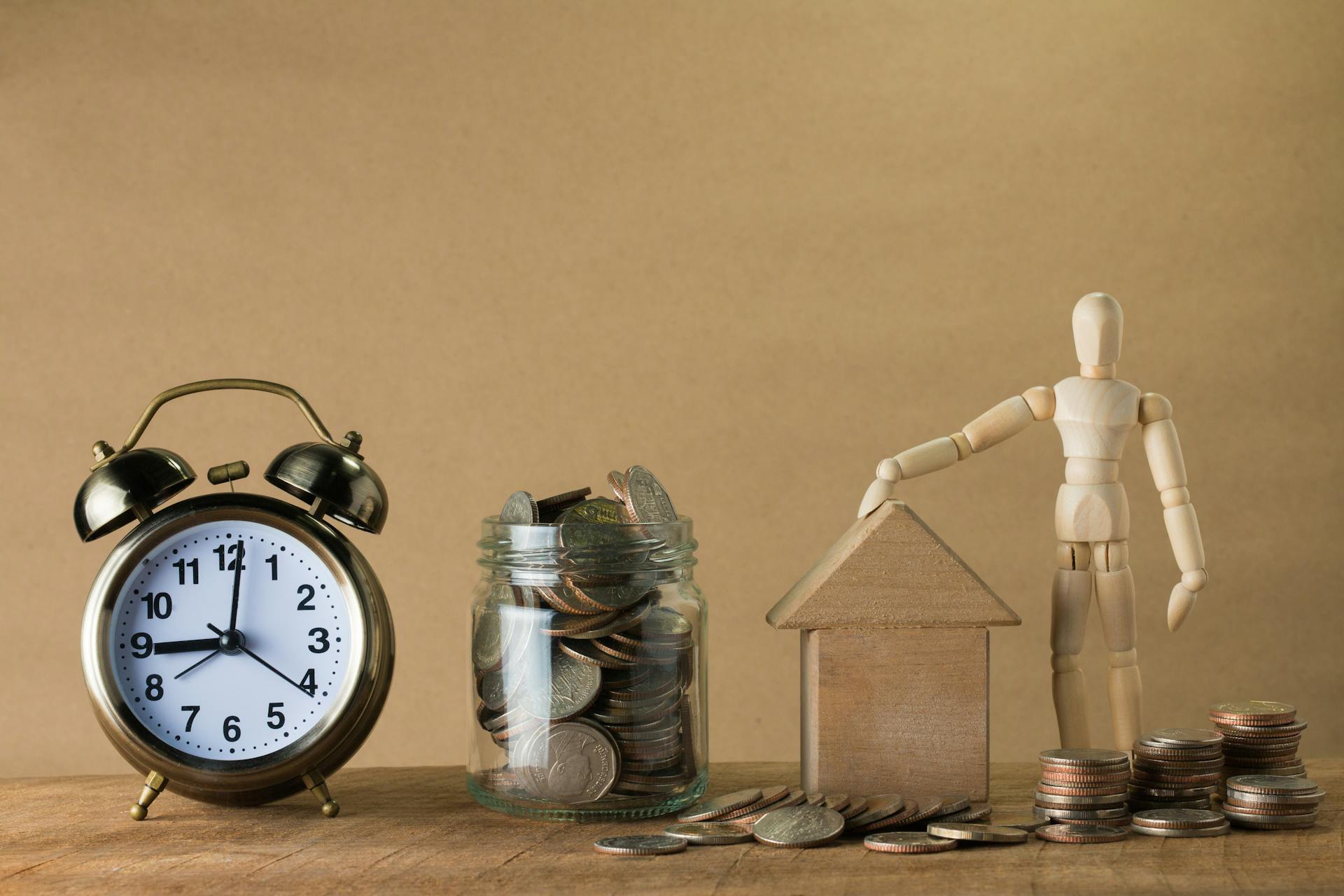
Monotonicity is another crucial property of an extension. It states that if X is greater than or equal to X', then φt (X) is greater than or equal to φt (X') for all t in the time set.
Locality is a property that states that φt (X) is equal to the essential infimum of the set of φt (Y) for all Y in the set of random variables that belong to the set A and are greater than or equal to X.
The proof of 17 also explores the concept of an extension being an extension of another dynamic LM-measure, denoted as φ. This means that φ is an extension of φ, and satisfies the same properties as φ.
The essential infimum is a concept that plays a crucial role in the proof of 17. It is the greatest lower bound of a set of random variables. In the context of proof 17, the essential infimum is used to define the properties of an extension.
Here is a summary of the properties of an extension:
These properties are essential in understanding the proof of 17 and the concept of an extension of a dynamic LM-measure. By examining these properties, we can gain a deeper understanding of the behavior of dynamic LM-measures and their extensions.
Proof of 6
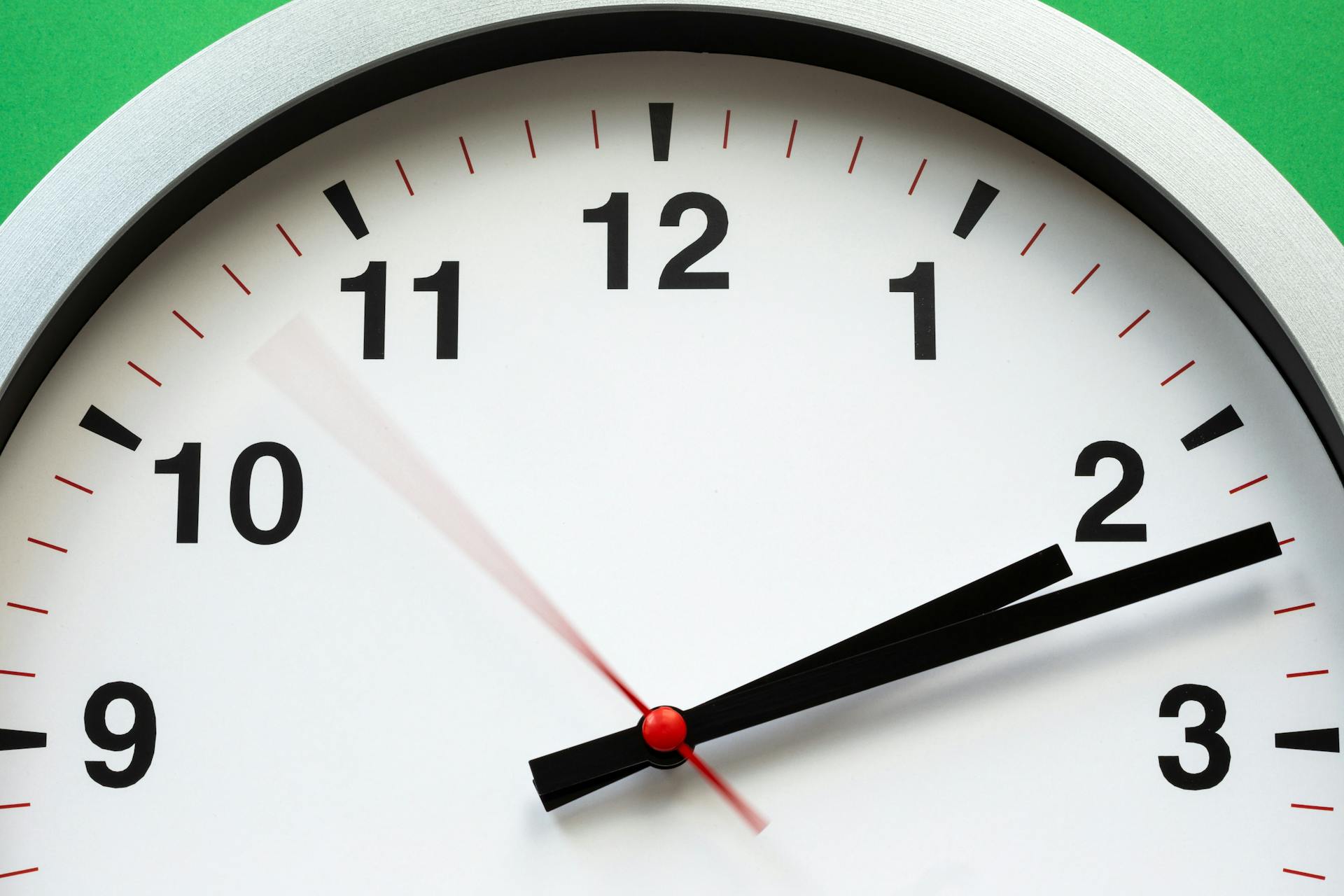
The proof of proposition 6 is a straightforward application of the properties of dynamic LM-measures.
In this case, the proof of monotonicity and locality is similar to the one for the conditional essential infimum and supremum, Proposition 16.
For any t∈ℝ+, Z∈Dt, and m∈ℝ+, since EZ|ℱt=1, we immediately get ϕt(m)=m, for any m∈ℝ+.
This shows that {ϕt}t∈ℝ+ is projective.
Let φ be a dynamic LM-measure which is ϕ-rejection time consistent, and g:ℝ→ℝ be an increasing, concave function.
Then, for any X∈L, we get g(φt(X))≥E[g(φs(X))|ℱt].
Recall that any Z∈Dt is a Radon-Nikodym derivative of some measure Q with respect to P, and thus we have EZX|ℱt=EQ[X|ℱt].
Hence, by Jensen’s inequality, we deduce g(φt(X))≥E[g(φs(X))|ℱt].
Combining these results, ϕ-acceptance time consistency of {g∘φt}t∈ℝ+ follows.
Proof of 14
In the proof of proposition 14, we see a dynamic LM-measure φ being used in conjunction with an update rule μ to establish time consistency.
The update rule μ is defined such that for any t
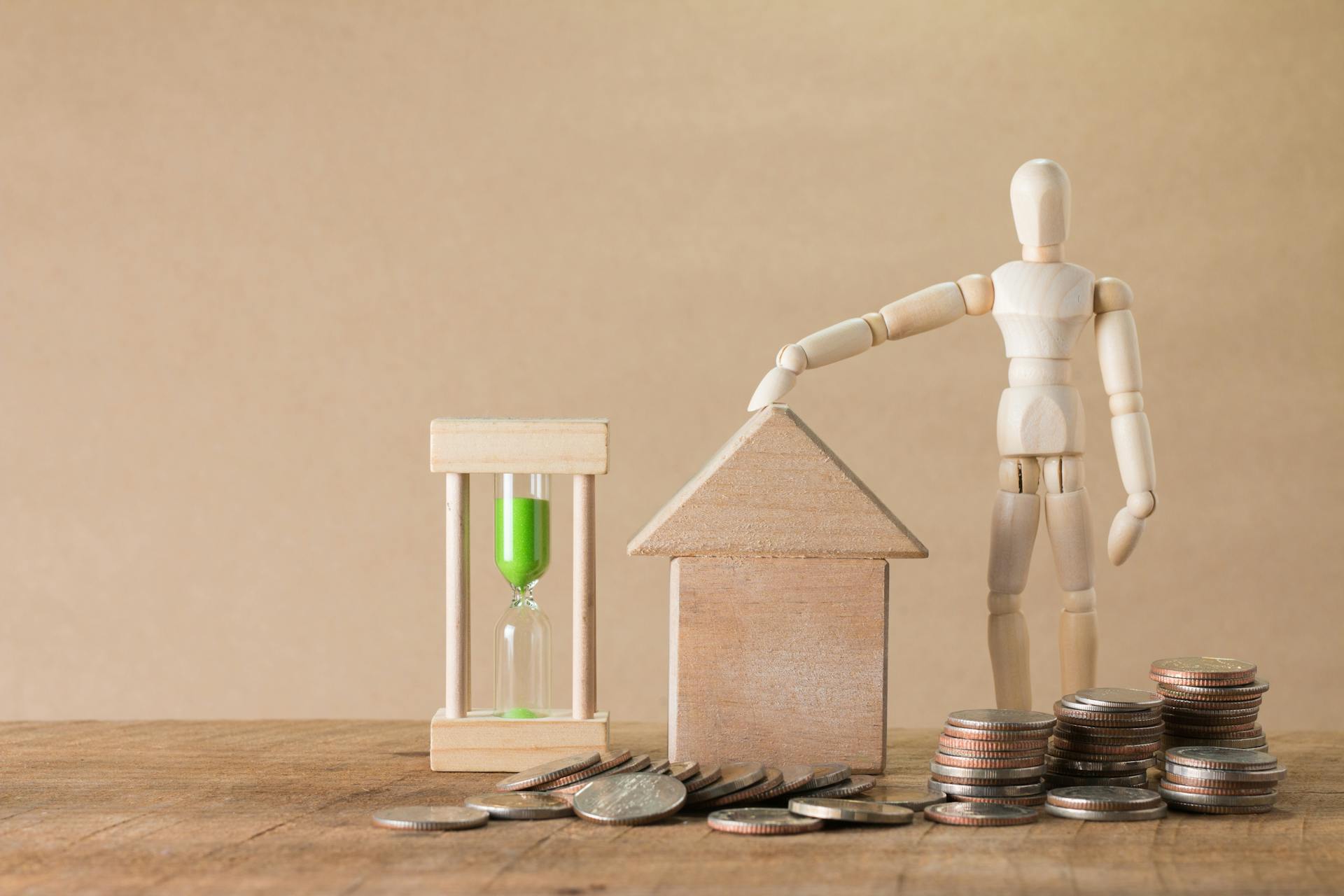
To establish strong time consistency, we need to show that for any V,V'∈ℝⁿ and t
The proof involves showing that the update rule μ satisfies certain properties, including being local and monotone on the space of random variables. This is done by constructing a map ϕt,t+1 that satisfies these properties, and then using this map to define the update rule μ.
Here are the key properties of the update rule μ:
- For any m∈ℝⁿ and V,V'∈ℝⁿ such that Vt=V't, we have μt,t+1(m,V)=μt,t+1(m,V').
- The family φ is both one-step μ-acceptance and one-step μ-rejection time consistent.
These properties ensure that the update rule μ is consistent with the dynamic LM-measure φ, and therefore establishes strong time consistency.
Frequently Asked Questions
What is time consistency risk measure?
A time consistent risk measure is a policy that remains effective throughout a decision-making process, ensuring that the approach taken at each stage aligns with the overall goal of minimizing risk. This consistency is crucial for making informed decisions in complex, multi-stage problems.
What is time consistency of optimization problems?
Time consistency of optimization problems refers to whether the optimal solution or policy remains unchanged regardless of when the problem is solved. This property ensures that decisions made today will still be optimal in the future, without relying on future information.
Sources
- https://probability-risk.springeropen.com/articles/10.1186/s41546-017-0012-9
- https://www.wikiwand.com/en/articles/Time_consistency_(finance)
- https://www.clevelandfed.org/publications/economic-commentary/2012/ec-201219-time-consistent-rules-in-monetary-and-fiscal-policy
- https://www.frbsf.org/research-and-insights/publications/economic-letter/2003/04/time-inconsistent-monetary-policies-recent-research
- https://quickonomics.com/terms/time-inconsistency/
Featured Images: pexels.com