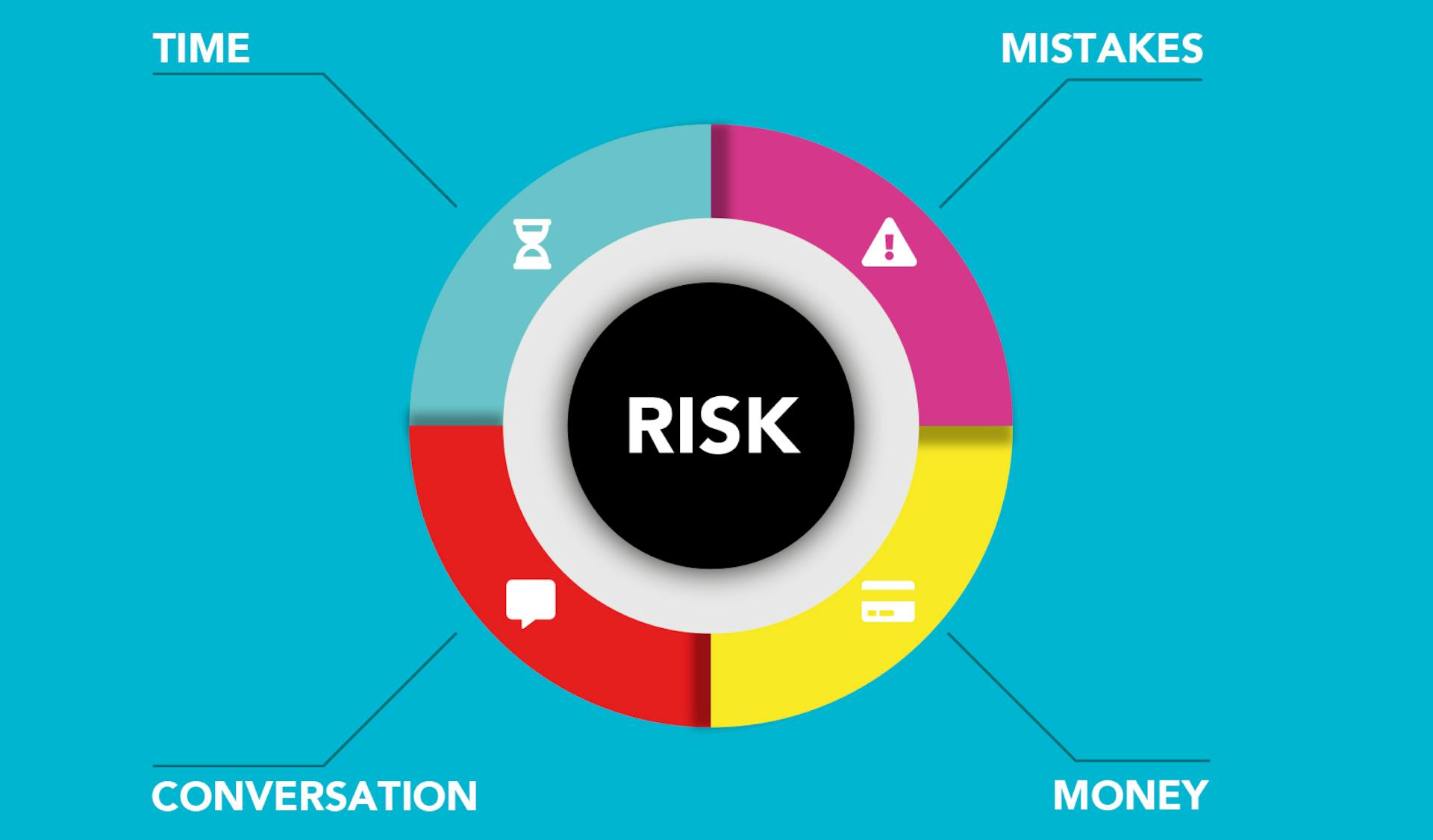
Dynamic risk measures are a crucial tool for financial institutions to assess and manage risk in a rapidly changing market environment. They provide a more accurate and comprehensive picture of risk than traditional static measures.
A dynamic risk measure is a type of risk measure that takes into account the time-varying nature of risk. As a result, it can provide a more accurate assessment of risk than a static risk measure, which is based on historical data. This is especially important for institutions with complex portfolios.
Dynamic risk measures can be used to identify potential risks and opportunities in a portfolio. For example, a dynamic risk measure can help an institution identify potential risks in a portfolio that are not apparent using a static risk measure.
By using dynamic risk measures, financial institutions can make more informed decisions and better manage their risk. This can lead to improved risk management and more effective use of resources.
Intriguing read: What Are the Risks of Getting Braces?
Understanding Dynamic Risk Measure
Dynamic risk measures are a step beyond static risk measures, which assess risk at a single point in time. They incorporate the concept of time and the potential changes that could occur in the future, making them more realistic and applicable to real-world trading scenarios.
Dynamic risk measures are based on the principle of time consistency. This means that the measure of risk at any given time should be consistent with the measure of risk at any other time, given the same information.
Time consistency is a crucial property for any risk measure, as it ensures that the measure is reliable and can be used to make consistent decisions over time.
For more insights, see: Standard Deviation Measures Which Type of Risk
Mathematics Behind Dynamic Risk Measure
The mathematics behind dynamic risk measures can be quite complex, involving concepts from probability theory, stochastic calculus, and financial mathematics. This complexity is due in part to the need to model the market as a stochastic process and solve differential equations to obtain the risk measure.
Dynamic risk measures involve sophisticated mathematical and computational techniques, which can be difficult to understand and implement. This can make them less accessible to traders and investors without a strong background in mathematics or finance.
At a high level, a dynamic risk measure is a function that assigns a numerical value to a financial position at each point in time, based on the potential losses that could be incurred in the future. This function can take various forms, such as Value-at-Risk (VaR), Expected Shortfall (ES), and Conditional Value-at-Risk (CVaR).
The Mathematics Behind
The Mathematics Behind Dynamic Risk Measures is a complex subject, involving concepts from probability theory, stochastic calculus, and financial mathematics.
At a high level, dynamic risk measures are functions that assign a numerical value to a financial position at each point in time, based on the potential losses that could be incurred in the future.
These functions can vary in form depending on the particular dynamic risk measure being used, with some common examples including Value-at-Risk (VaR), Expected Shortfall (ES), and Conditional Value-at-Risk (CVaR).
Related reading: Financial Risk and Non Financial Risk
Each of these measures has its own strengths and weaknesses, and is suitable for different types of trading strategies and risk profiles.
Stochastic calculus and numerical methods are mathematical techniques often used to calculate dynamic risk measures, involving modeling the market as a stochastic process and solving a set of differential equations to obtain the risk measure.
These methods can provide more accurate results than Monte Carlo simulation, especially for simple trading strategies and markets.
However, they can be more complex to implement and require a good understanding of the mathematical theory behind them.
The complexity of dynamic risk measures can make them less accessible to traders and investors, especially those without a strong background in mathematics or finance.
This complexity can also make them more prone to errors and misinterpretations, which can lead to inaccurate risk assessments and poor decision-making if not properly managed.
If this caught your attention, see: Risk Measures
Expected Shortfall (ES)
Expected Shortfall (ES) is a measure of the expected loss in the worst-case scenarios.
See what others are reading: Expected Shortfall
It's a more sensitive measure of risk than VaR, which makes it a more conservative approach. However, it's also more complex to calculate.
ES is also known as Conditional Value-at-Risk (CVaR), which gives you an idea of its focus on worst-case scenarios.
This measure can be less intuitive to interpret, which can make it challenging to understand.
Featured Images: pexels.com