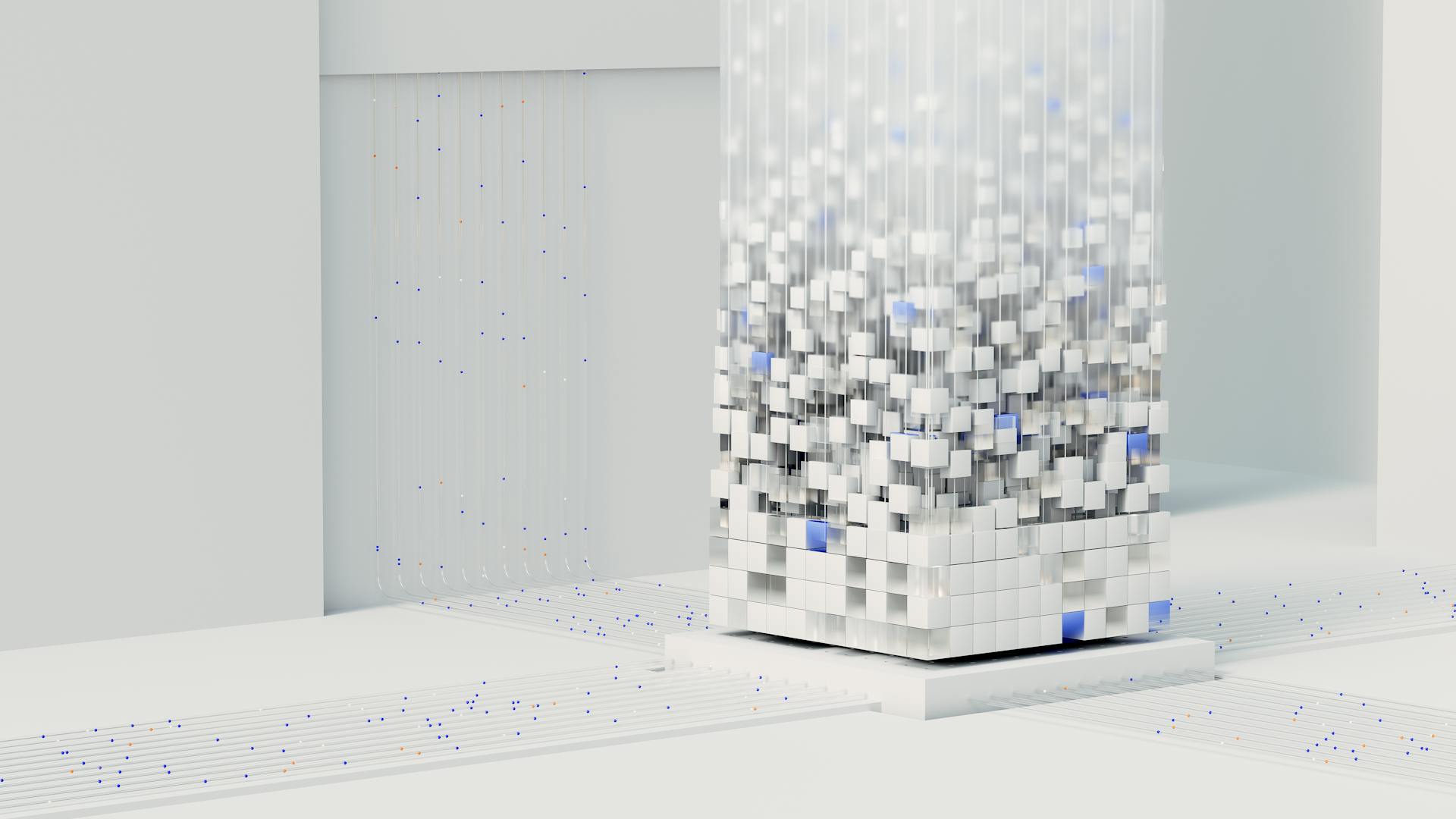
Credibility theory is a crucial concept that has far-reaching implications in various fields, including finance, marketing, and communication. In essence, credibility theory refers to the process of establishing trust and confidence between a sender and a receiver.
Credibility is built on a foundation of reliability, expertise, and likability. According to research, people are more likely to believe someone who is perceived as reliable and trustworthy. This is because reliability is a fundamental aspect of credibility, and it sets the stage for building trust.
A key aspect of credibility theory is the concept of source credibility, which suggests that the credibility of the sender is a critical factor in determining the credibility of the message. In other words, if the sender is perceived as credible, the message is more likely to be believed.
What is Credibility Theory?
Credibility theory is a statistical approach used primarily in actuarial science to make predictions about future claims or events based on past observations.
It helps actuaries understand the risks associated with providing coverage and allows insurance companies to limit their exposure to claims and losses.
Credibility theory uses mathematical models and methods for making experience-based estimates, which refers to historical data.
These models help actuaries balance past data with external sources such as global data or expert opinions to estimate parameters.
It's a powerful tool for insurance companies to make informed decisions and manage risk effectively.
Credibility theory is used to estimate risk by examining data and making experience-based estimates, which is a key part of actuarial science.
Here's an interesting read: A Report Which Is Based on Creditworthiness and Personal Characteristics
Types of Credibility Theory
Credibility theory helps us understand how likely it is that a particular experience or statistic belongs to a certain class or group. Bayesian credibility is one type of theory that breaks down each class into its own probability and then calculates the likelihood of our experience within each class.
We can find the probability of our class given our experience by weighing each statistic with the probability of the particular class. This means that if we have a high-scoring basketball team, its unique performance will greatly increase the variance of the population.
Bühlmann credibility, on the other hand, looks at the variance across the population to determine credibility. It separates the total variance into two parts: the variance of the hypothetical mean and the expected value of the process variance.
History and Development
Credibility theory has a rich history that dates back to the 18th century.
Thomas Bayes is credited with developing the theory, which aims to make more accurate forecasts of uncertain future events by incorporating new information.
The goal of credibility is to update and revise forecasts based on new information, making it a dynamic and adaptive approach to forecasting.
Who Developed?
Thomas Bayes is credited with developing the credibility theory in the 18th century.
Credibility theory aims to make more accurate forecasts of future events by incorporating new information to update and revise those forecasts.
Buhlmann
Buhlmann theory relies on past experience to update estimates and provide a credible interval, similar to Bayes' theorem.
The Buhlmann model, also known as the Cape Cod model, applies random effects to prior experience to come up with proportional weighting.
This model is used by actuaries and insurance companies to calculate their loss reserves.
If this caught your attention, see: Merton Model
Actuarial Science and Credibility Theory
Actuarial science heavily relies on credibility theory, particularly in the field of insurance and risk management. This is because the primary aim is to predict the likelihood of future events, making it essential to balance company-specific data with extensive industry-wide studies.
Actuaries use credibility theory to calculate premiums by deciding how much variance to attribute to individual claims data versus the collective industry risk. This helps in setting fair premiums while also maintaining company solvency.
The Bayesian approach plays a significant role in actuarial credibility, involving the updating of probability estimates for a hypothesis as new evidence or information becomes available. This makes predictions more adaptive and informed.
In actuarial applications, credibility theory often intersects with other statistical principles, such as blending Bayesian approaches with credibility theory to create a more dynamic system of analysis.
A small health insurance provider might initially heavily weigh national health statistics to set the base premium, but over time, they can adjust the credibility factor to better reflect actual experience, leading to more accurate premium calculations.
Explore further: Health Insurance Asking about Accident or Injury
Actuaries may use a small data set when creating an initial estimate, but large data sets are ultimately preferred because they have greater statistical significance.
Here are some advanced credibility techniques used in actuarial science:
- Conjugate Priors: These are used within Bayesian approaches to align prior distributions, offering mathematically convenient updates as new data arises.
- Mixed Linear Models: These are utilized to work with hierarchical datasets, allowing you to model complex nonlinear relationships present in actuarial data.
- Generalized Linear Models (GLMs): These models bring flexibility in modeling different types of data distributions, enabling robust predictions even in varied contexts.
Generalized Linear Models (GLMs) involve a link function that relates the expected value of the data distribution to the linear predictor, enhancing predictive capacities. This involves multiple independent variables influencing dependent outcomes, broadening application scopes.
In actuarial contexts, employing GLMs allows companies to handle varied insurance products systematically, properly coordinating link functions, expected values, and coefficients.
A health insurer might employ GLMs to estimate policyholder risk by using extensive historical health data, predicting future claims more precisely, and tuning policy conditions accordingly.
If this caught your attention, see: Expected Shortfall
Techniques by Industry
Credibility theory is a valuable tool across various sectors, including the insurance industry, financial institutions, and healthcare analytics. It helps organizations predict future trends and outcomes by synthesizing both historical data and industry benchmarks.
In actuarial science, credibility techniques are foundational in calculating reserves for future payouts. Companies analyze historical claims data alongside mortality rates to better set aside funds, ensuring sustainability.
Marketing and consumer analytics harness credibility techniques for customer segmentation and personalization strategies. Data from customer interactions are paired with market research to achieve refined audience targeting.
Operations management employs credibility estimates for inventory forecasting. By combining sales history with market dynamics, firms optimize stock levels and minimize waste.
A manufacturing company predicts future product demands using credibility theory. They merge sales data with industry trends to determine the best stock levels.
The key to employing credibility in these contexts involves gathering extensive historical data, using statistical models to calculate the credibility factor (Z), and integrating internal insights with larger market datasets for balanced outcomes.
Here's a breakdown of the different industries and their corresponding credibility techniques:
In actuarial contexts, employing Generalized Linear Models (GLMs) allows companies to handle varied insurance products systematically. The model's structure leverages both categorical and continuous data.
A health insurer employs GLMs to estimate policyholder risk. By using extensive historical health data, they predict future claims more precisely, tuning policy conditions accordingly.
The Buhlmann Model provides a structured way to determine the credibility factor by balancing past experiences with broader datasets pertaining to specific metrics. This model is useful for assessing premiums in the insurance industry.
The Empirical Bayes Method refines predictions as additional data becomes available, adapting to new patterns. This method is suitable for diverse and dynamic datasets.
The Bayesian Approach updates predictions by combining prior distributions with new evidence. It is a more advanced method that is adaptive and robust, making it suitable for various applications.
Discover more: Bornhuetter–Ferguson Method
Decision Making
Credibility theory plays a crucial role in decision making by providing a framework for evaluating how much you should trust specific data sources over others. This ensures informed and reliable business decisions, minimizing risks and uncertainties.
In business studies, credibility is often viewed through the lens of credibility theory, which focuses on quantifiable metrics that can predict future outcomes based on historical data. The credibility factor (Z) is at the heart of credibility theory, ranging from 0 to 1, indicating the weight assigned to one's data in contrast to the weight given to an external data source.
See what others are reading: Risk Management in Insurance Business
The credibility factor can be adjusted as more internal data becomes available, increasing accuracy in predictions over time. A common formula used to calculate the credibility factor is: Z = n / (n + k), where n is the number of observations and k is a value that reflects the variance.
To better illustrate, consider a case where you have observed historical data regarding product sales. The calculation of the credibility factor typically depends on the variance and volume of past data. A common formula used is: Z = n / (n + k), where n is the number of observations (i.e., the volume of your historical data) and k is a value that reflects the variance.
Credibility theory offers the statistical methodologies to balance current and past data, leading to more accurate and consistent decision-making outcomes. Understanding this enhances your company's adaptability to ever-changing environments.
By using credibility theory, organizations are better equipped to assess the reliability of their data, integrate external sources, and make decisions that stand the test of time.
Here are some ways credibility theory impacts business decisions:
- Risk Management: Helps in evaluating risks by combining company-specific data with industry benchmarks.
- Forecasting: In business forecasting, credibility theory aids in more accurate predictions by giving due weight to different datasets based on their reliability.
- Strategic Planning: By integrating data from various sources, it becomes easier to plan long-term strategies which are robust against data variability and market shifts.
Sources
- https://en.wikipedia.org/wiki/Credibility_theory
- https://link.springer.com/book/10.1007/3-540-29273-X
- https://encyclopediaofmath.org/wiki/Credibility_theory
- https://www.investopedia.com/terms/c/credibility-theory.asp-0
- https://www.vaia.com/en-us/explanations/business-studies/actuarial-science-in-business/credibility-theory/
Featured Images: pexels.com