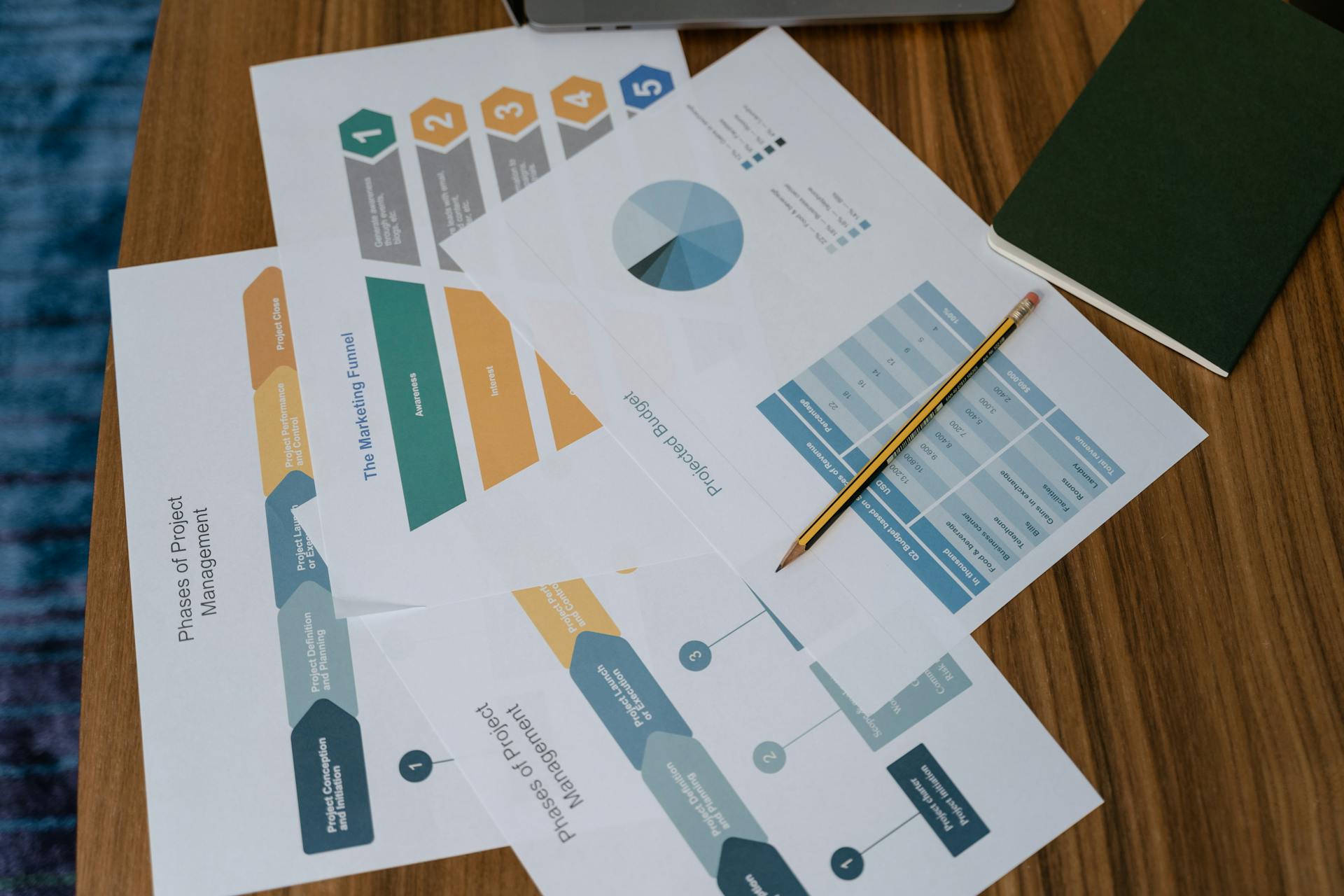
Brinson Performance Attribution Models for Portfolio Analysis is a crucial tool for investors and financial analysts.
The Brinson model breaks down portfolio returns into three components: policy, implementation, and market. This helps investors understand the sources of their returns and make informed decisions.
The policy component accounts for the investor's overall asset allocation strategy. This includes the mix of stocks, bonds, and other assets in the portfolio.
What is Brinson Performance Attribution
Brinson Performance Attribution is a method of breaking down a portfolio's return into its components, making it easier to understand how a manager achieved those returns. This analysis involves comparing the portfolio return with the benchmark return and attributing the difference to various components, such as allocation, selection, and interaction effects.
The allocation effect measures how much return is due to the weighting of different asset classes or sectors in the portfolio relative to the benchmark. The selection effect measures how much return is due to the choice of specific securities within each asset class or sector. The interaction effect measures how much return is due to the combined effect of allocation and selection decisions.
Here are the three main components of Brinson Performance Attribution:
- Allocation effect: measures the return due to the weighting of different asset classes or sectors
- Selection effect: measures the return due to the choice of specific securities
- Interaction effect: measures the combined effect of allocation and selection decisions
Overview
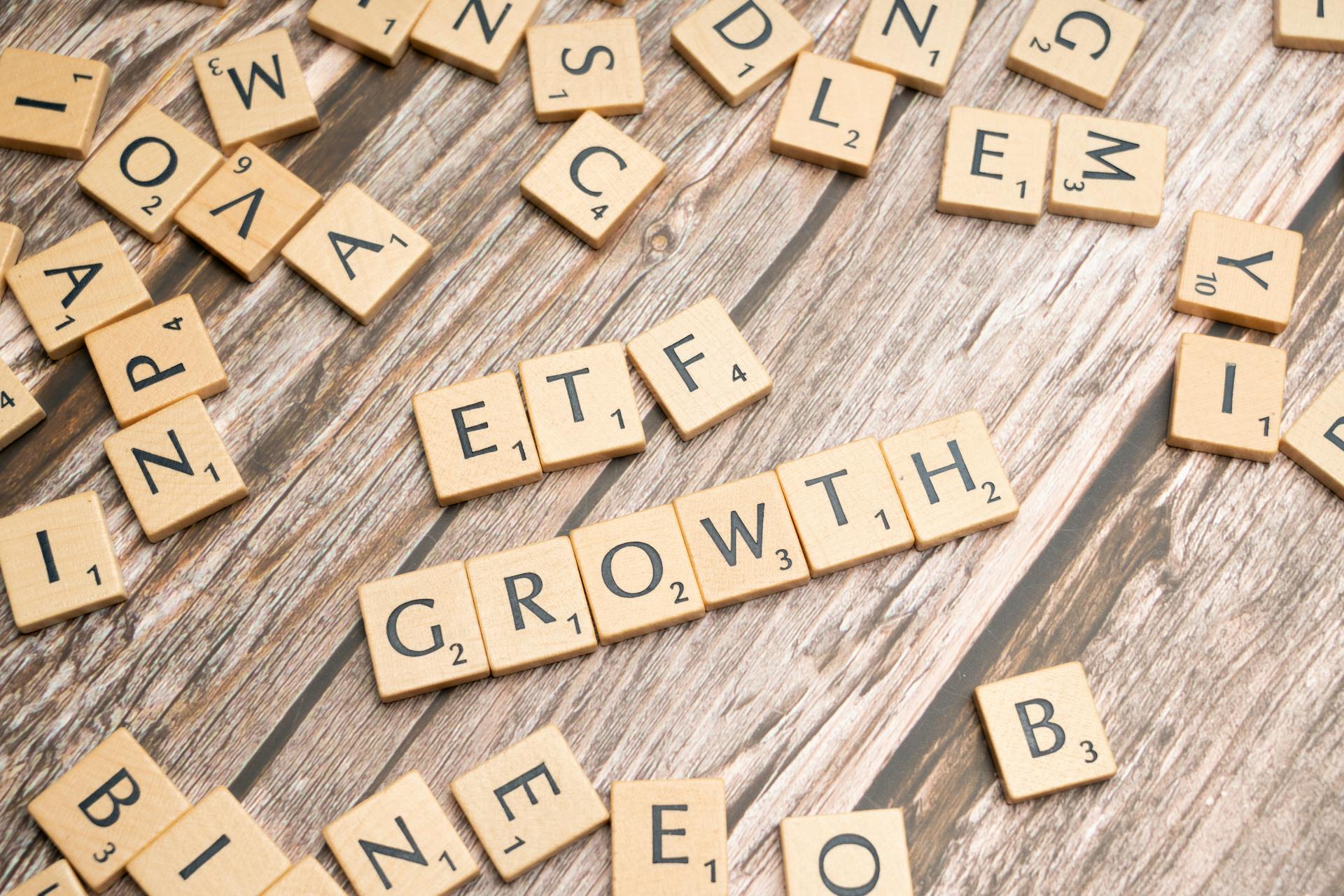
Brinson Performance Attribution is a tool that helps you understand the return drivers of your investment strategy. Portfolio Attribution is the broader concept that Brinson is a part of.
It's a simple and intuitive approach that's easy to grasp, and it's been provided in a pandas-based Python implementation in a previous post.
Basic Concepts
Brinson Performance Attribution breaks down a portfolio's return into sources like allocation (weighting of asset classes) and selection (performance of chosen securities) to understand how a manager achieved those returns. This helps portfolio managers identify the contributions from asset allocation, security selection, and market timing.
The most common components of performance attribution analysis are allocation, selection, and interaction effects. Allocation effect measures how much return is due to the weighting of different asset classes or sectors in the portfolio relative to the benchmark. Selection effect measures how much return is due to the choice of specific securities within each asset class or sector.
Explore further: Scion Asset Management Performance
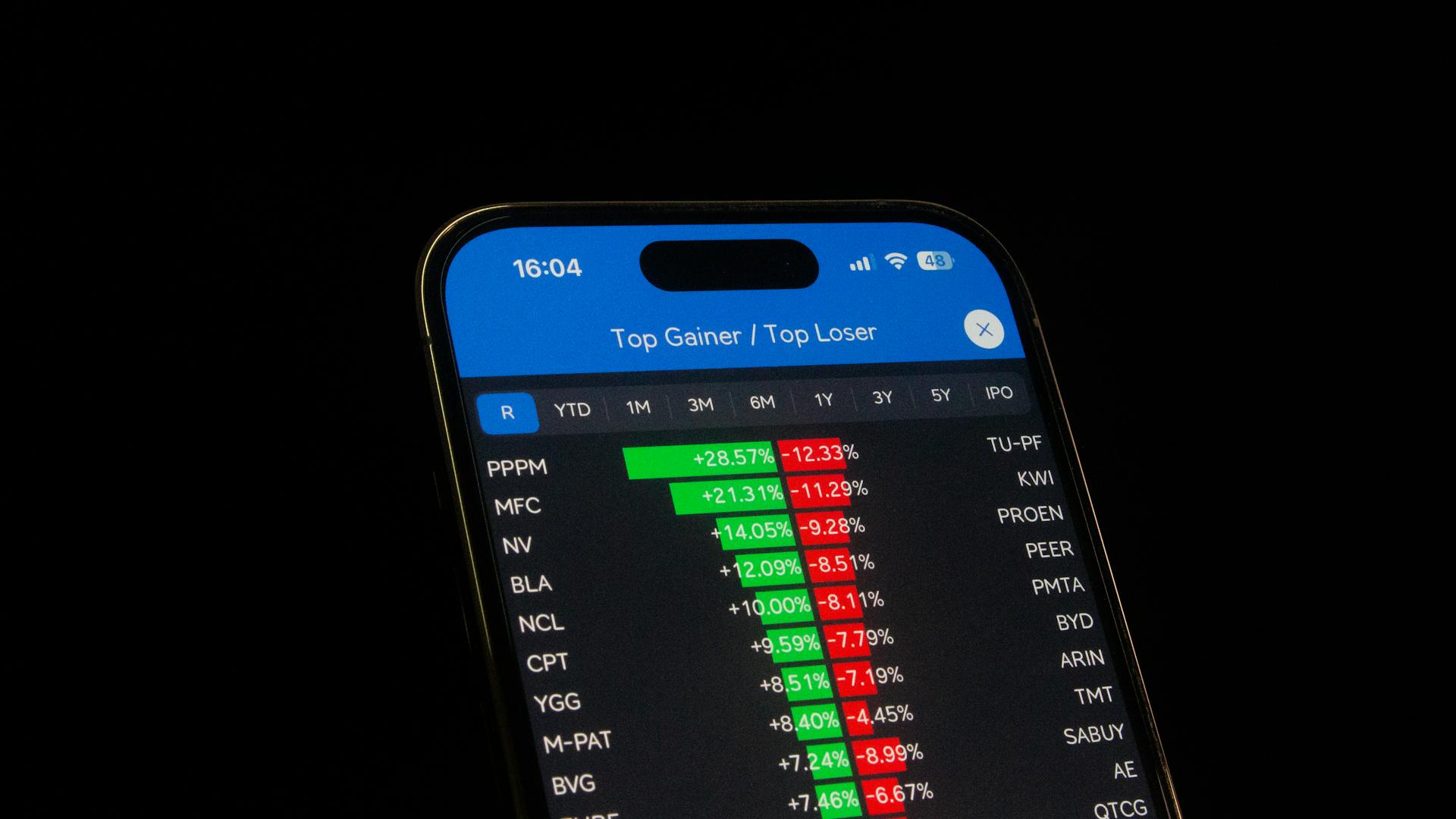
Here's a breakdown of these components:
Performance attribution analysis is a key tool in evaluating investment strategies' effectiveness and refining future decision-making. By comparing a portfolio's performance against a benchmark, it pinpoints which investment decisions drove returns.
Brinson Model
The Brinson Model is a method of decomposing portfolio returns into their constituent parts. It's a powerful tool for understanding how a portfolio's performance compares to a benchmark.
The Brinson Model works by calculating the total portfolio return as the sum of the weights and returns of each sector within the portfolio. This is done using the following formula:
$$
R_i = \sum w_i \times R_i
$$
Where $w_i$ is the weight of the i sector in the portfolio, $R_i$ is the return of the portfolio assets in the i sector, and $n$ is the number of sectors or securities.
To illustrate this, let's look at an example:
Using this data, we can calculate the total portfolio return as follows:
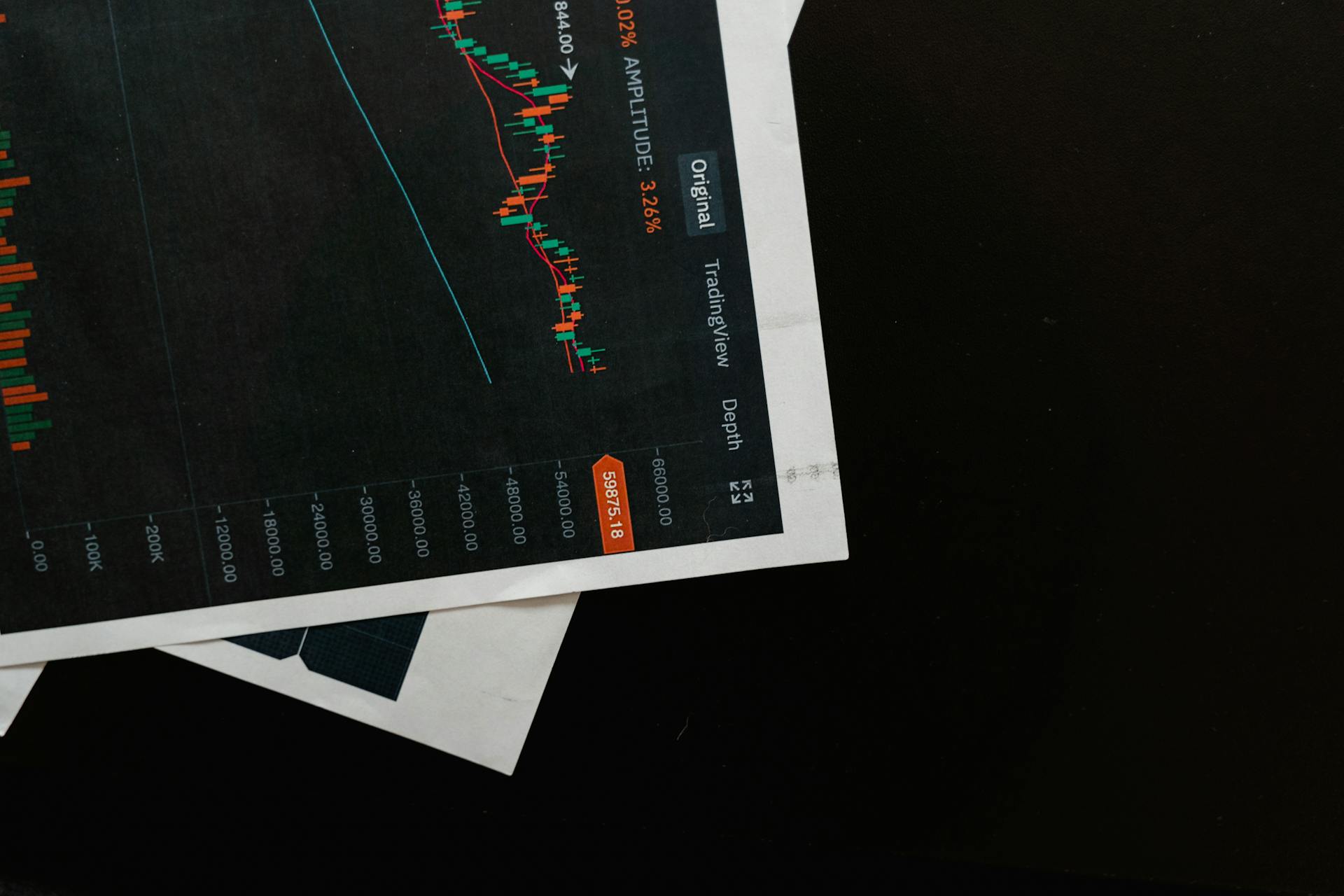
$$
60\% \times 18\% + 40\% \times 10\% = 10.8\% + 4\% = 14.8\%
$$
The Brinson Model can also be used to calculate the excess return, which is the difference between the portfolio return and the benchmark return.
The Brinson Model breaks down the excess return into three attribution effects: allocation, selection, and interaction.
Here's a summary of the three attribution effects:
- Allocation effect: the difference between the portfolio and benchmark weights for each sector, multiplied by the benchmark return.
- Selection effect: the difference between the portfolio and benchmark returns for each sector, multiplied by the benchmark weight.
- Interaction effect: the product of the difference between the portfolio and benchmark weights, and the difference between the portfolio and benchmark returns.
These attribution effects can be calculated using the following formulas:
- Allocation effect: $A_i = (w_i - W_i)B_i$
- Selection effect: $S_i = W_i(R_i - B_i)$
- Interaction effect: $I_i = (w_i - W_i)(R_i - B_i)$
Let's calculate the allocation effect for the Industrials sector:
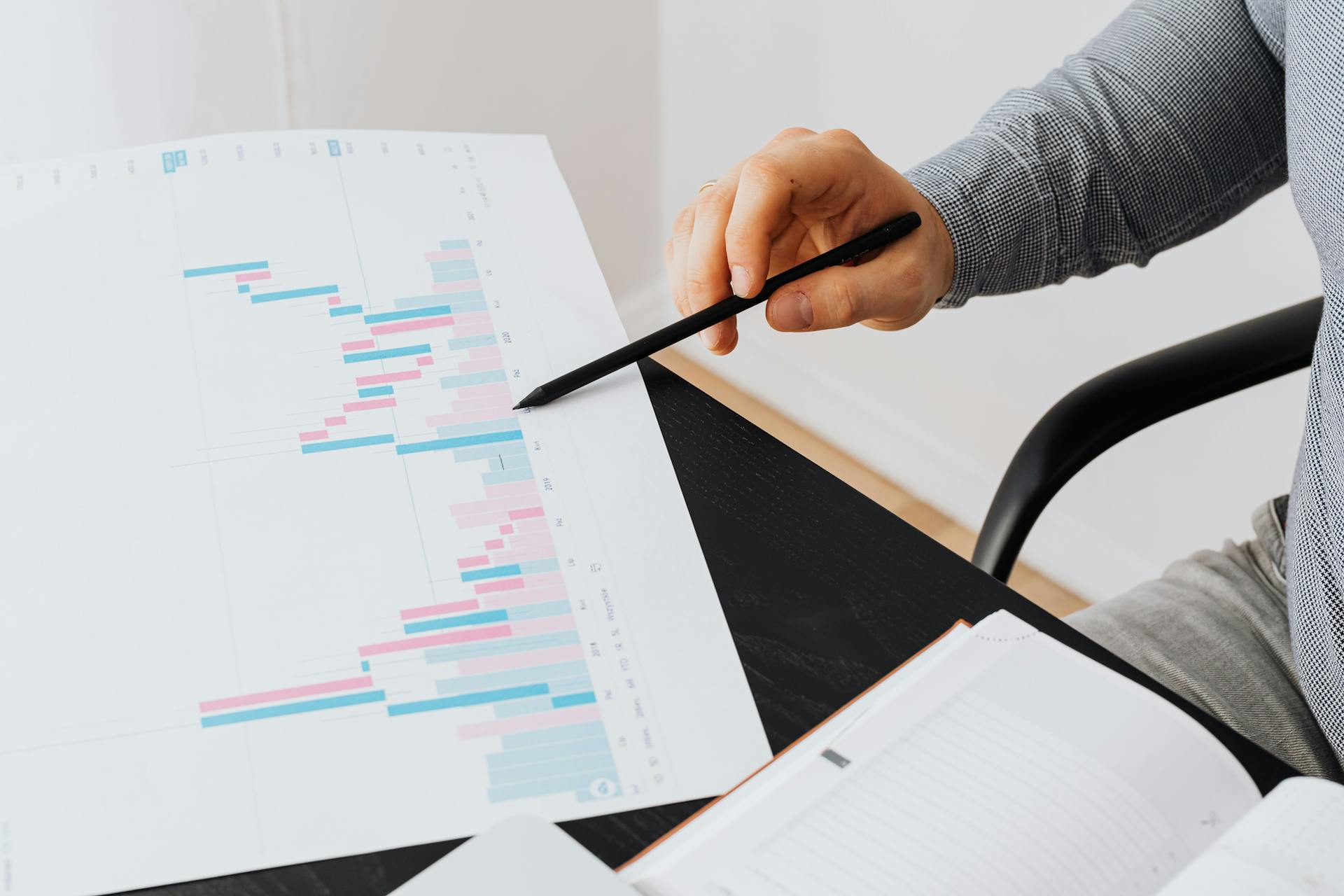
$A_i = (60\% - 50\%) \times 10\% = 1\%$
The total allocation effect is then calculated by summing the individual sector contributions:
Total allocation effect = 1% - 1.2% = -0.2%
Similarly, we can calculate the selection effect for the Industrials sector:
$S_i = 50\% \times (18\% - 10\%) = 4.8\%$
The total selection effect is then calculated by summing the individual sector contributions:
Total selection effect = 4.8% + -0.8% = 4.0%
Finally, we can calculate the interaction effect for the Industrials sector:
$I_i = (60\% - 50\%) \times (18\% - 10\%) = 0.8\%$
The total interaction effect is then calculated by summing the individual sector contributions:
Total interaction effect = 0.8% + 0.2% = 1.0%
By breaking down the excess return into these three attribution effects, the Brinson Model provides a powerful tool for understanding how a portfolio's performance compares to a benchmark.
Discover more: Buy Performer 8
Risk-Based Performance
Risk-Based Performance provides a more thorough view of return and risk, breaking down active risk and corresponding return from various factors. This allows for a granular understanding of risk exposures and how they drive returns.
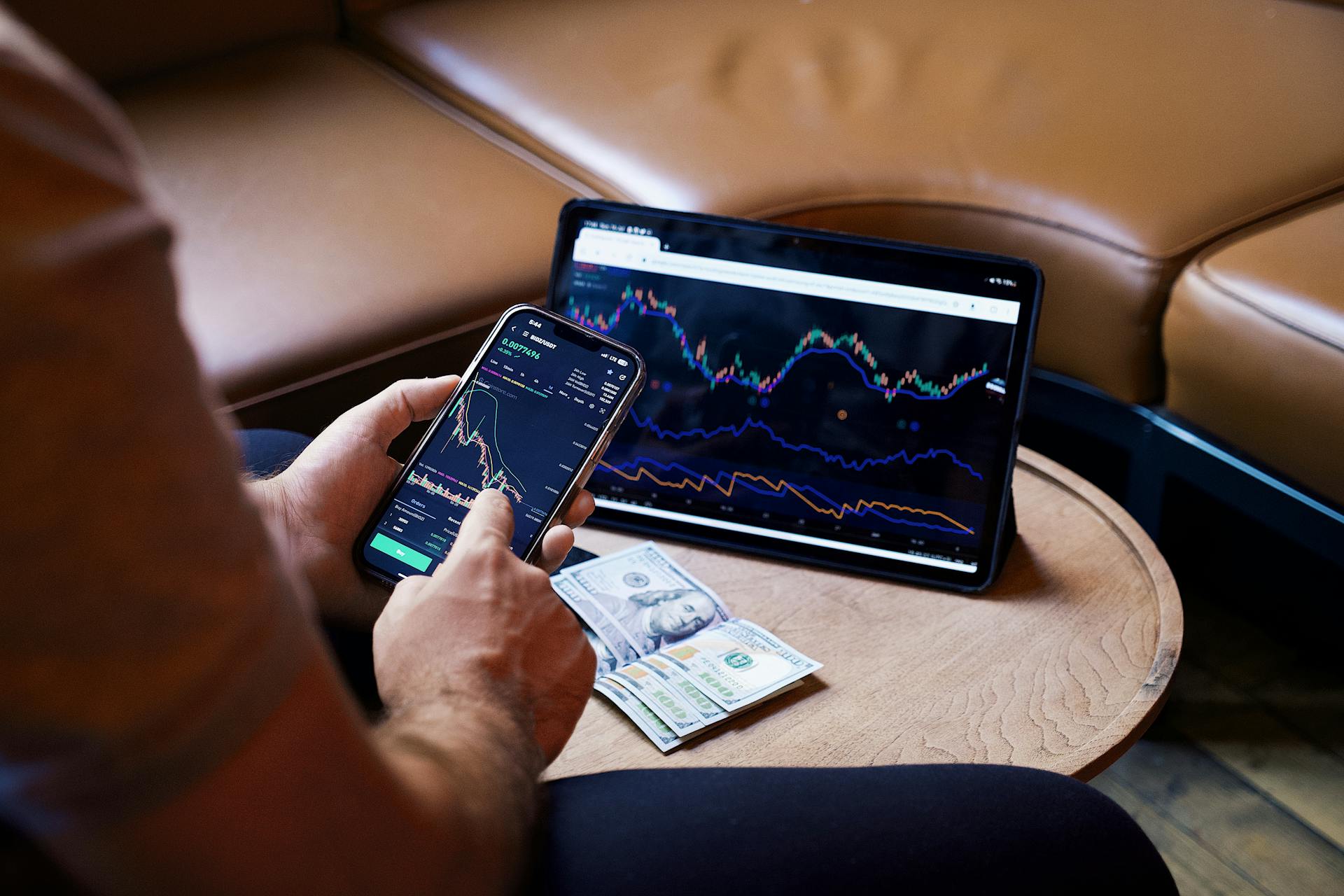
Unlike Brinson attribution, risk-based performance attribution uses factor risk models to provide a comprehensive picture of risk/return drivers and exposures within a portfolio. It decomposes active risk and return into factor active risk and return and specific active risk and return.
Factor active risk and return can be further broken down into style factors, industry exposures, and currency/country impacts, providing a detailed view of the portfolio's risk profile. This is in contrast to Brinson attribution, which lacks risk insight and does not provide analysis into underlying return drivers or intended vs. unintended exposures.
The Allocation Effect
The Allocation Effect is a crucial aspect of risk-based performance analysis. It helps us understand how a portfolio's sector allocation contributed to its overall return.
To calculate the allocation effect, we need to calculate the contribution to allocation for each sector. This is done by multiplying the portfolio's sector weight minus the benchmark's sector weight by the benchmark sector return.
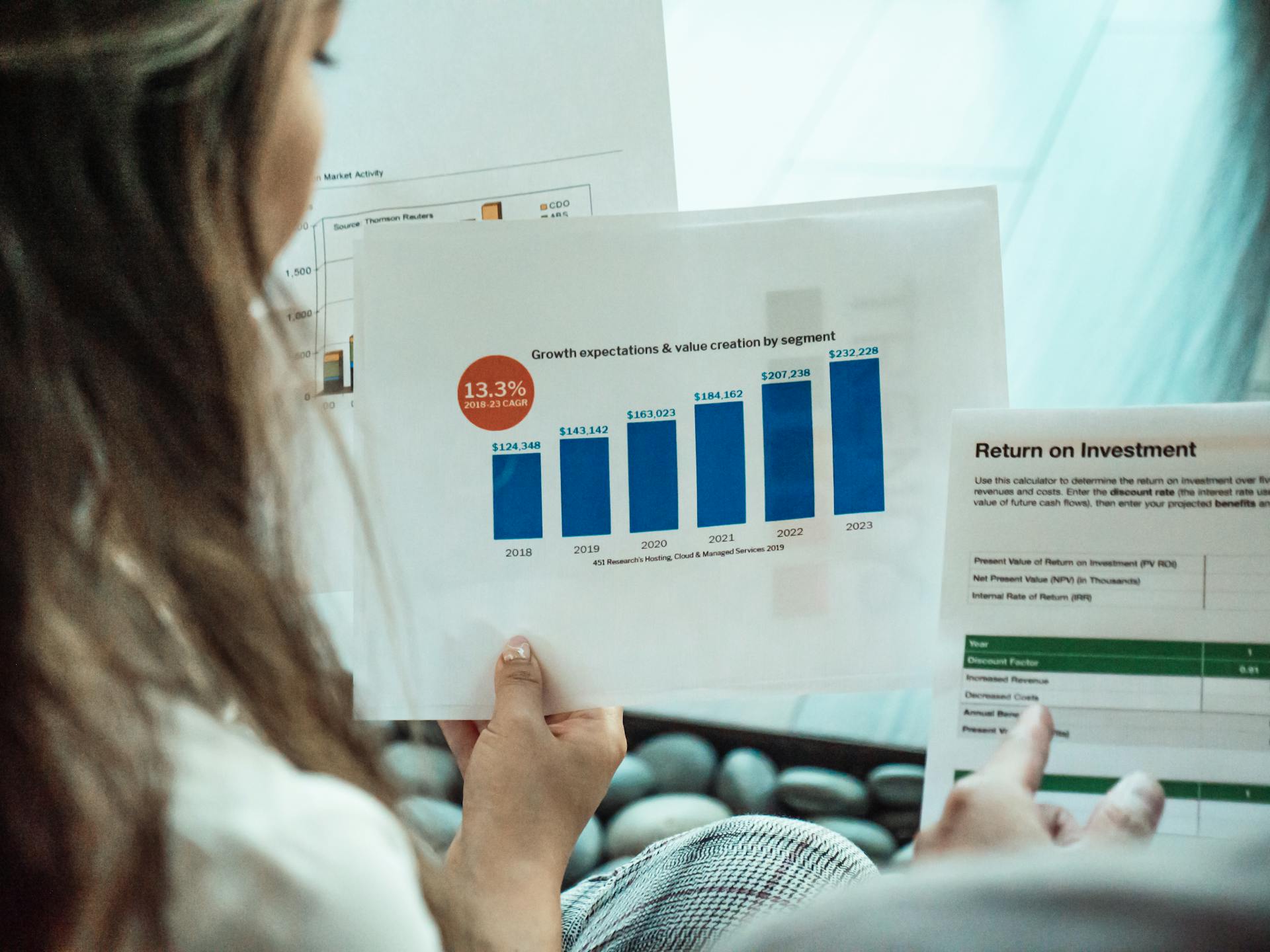
For example, let's take the Industrials sector, which had a portfolio weight of 60% and a benchmark weight of 50%. With a benchmark return of 10%, the allocation effect for Industrials would be (60% - 50%) x 10% = 1%.
The total portfolio allocation effect is then calculated by summing up the individual sector contributions to allocation. In this case, the total allocation effect would be 1% - 1.2% = -0.2%.
This means that the portfolio's allocation to Industrials actually detracted from its overall return.
Risk-Based Performance
Risk-Based Performance is a more detailed and insightful way to evaluate portfolio and manager performance. It uses factor risk models to provide a granular view of risk exposures and how they drive returns.
Active risk and active return are decomposed into factor active risk and return, and specific active risk and return. This breakdown allows for a comprehensive picture of risk/return drivers and exposures within a portfolio.
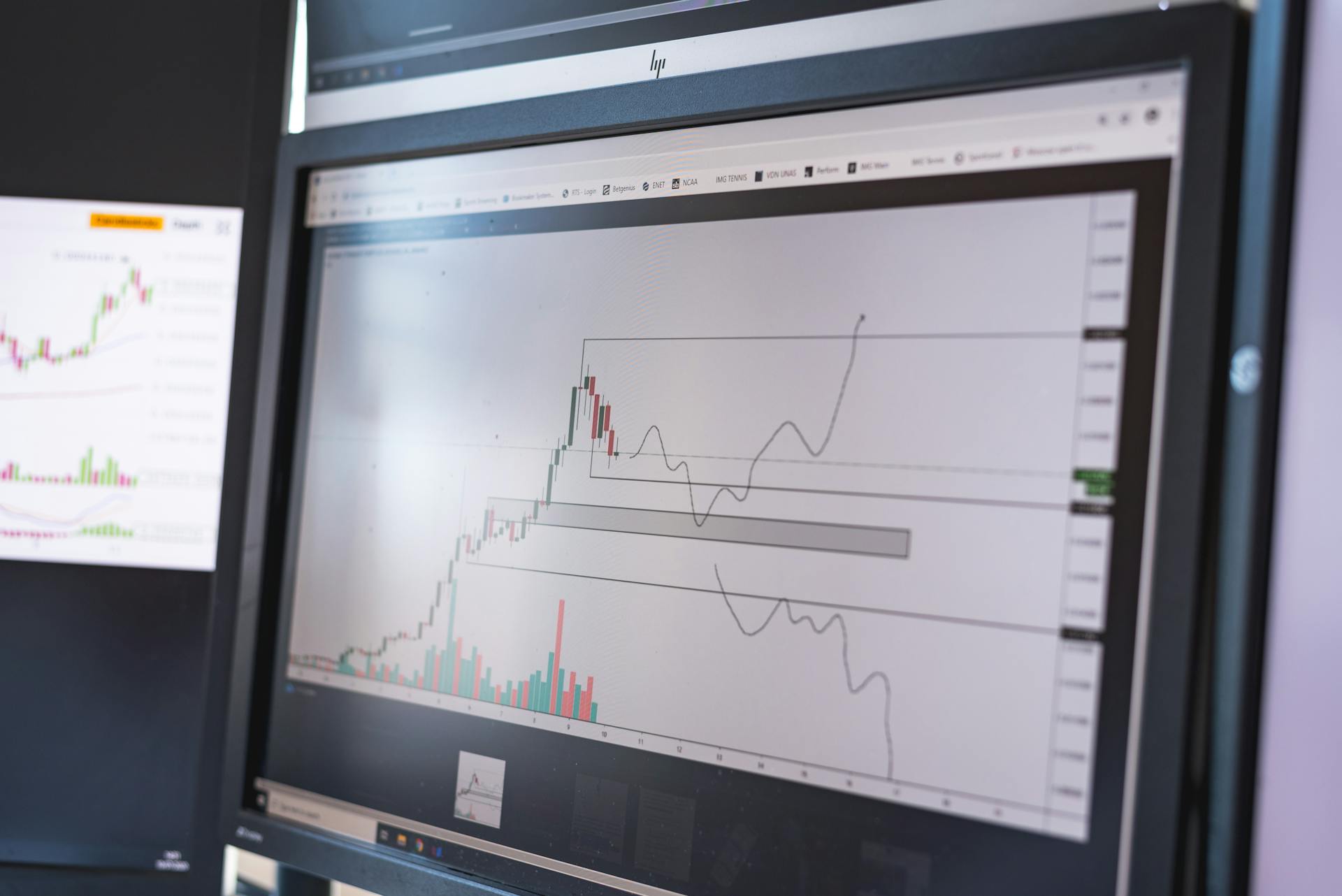
Factor active risk and return can be further broken down into style factors, industry exposures, and currency/country impacts. This level of detail is not available with traditional Brinson attribution methods.
Risk-based performance attribution can identify which exposures are driving returns and which are driving risk. For example, in a dividend-focused ETF, the underlying methodology is designed to capture dividend-paying stocks, but if the dividend yield factor is not performing well, it can actually hurt performance.
In a case study, a risk-based performance attribution analysis for a dividend-focused ETF revealed that the largest positive contribution to return came from market sensitivity, also known as beta. The strategy's low beta nature was the main positive driver of performance in 2021.
Market sensitivity accounted for more than one third of active risk in this example, highlighting the importance of beta in driving performance and risk.
A different take: Stock Market Performance History
Fachler Model
The Brinson-Fachler Model is an extension of the Brinson Model, with one key difference in how individual sector allocation effects are calculated.
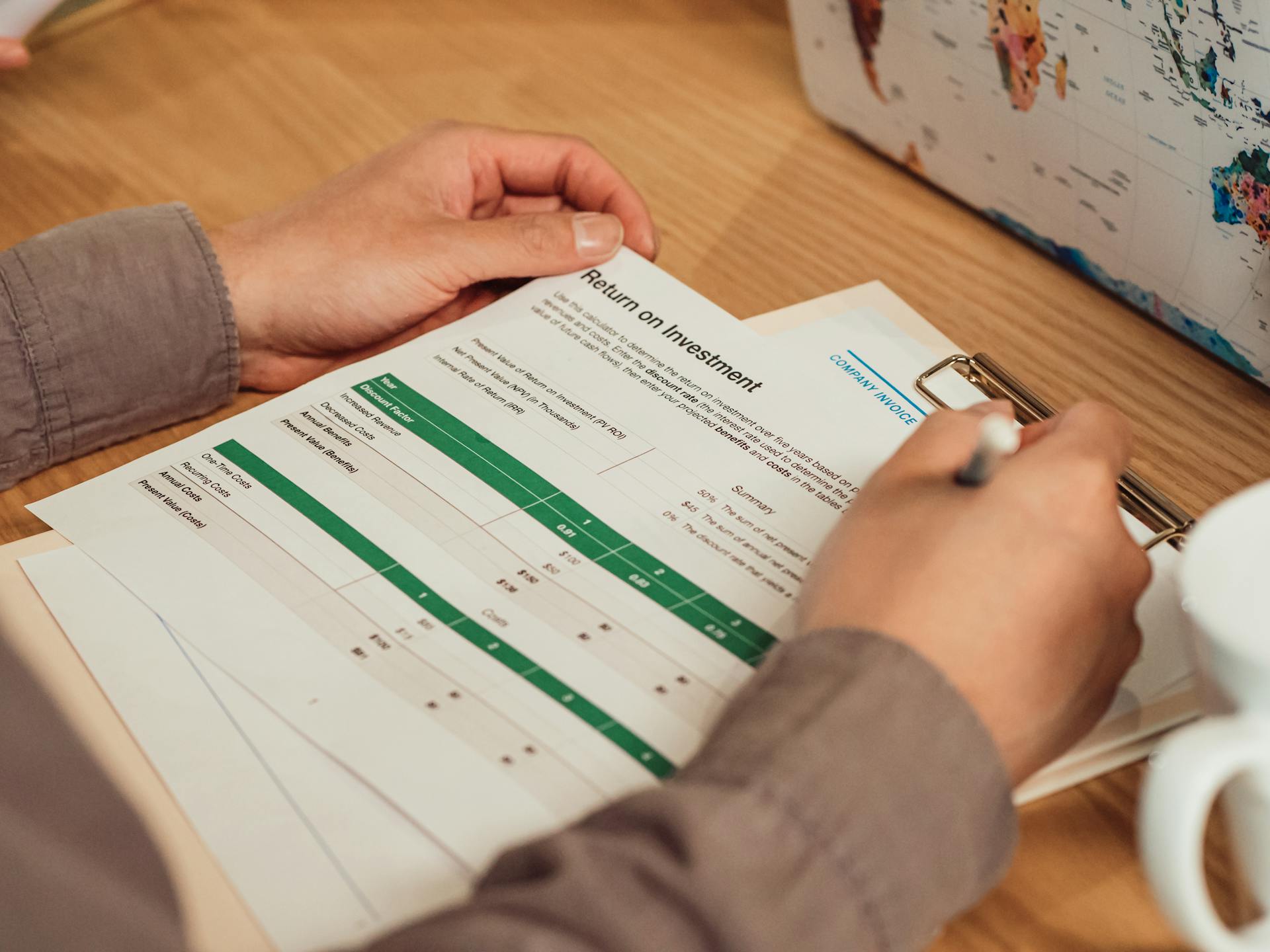
The Brinson-Fachler Model calculates sector allocation effects as (w_i – W_i)(B_i – B), where A_i represents the sector allocation effect, w_i is the weight of the sector, W_i is the weight of the sector in the benchmark, B_i is the return of the sector, and B is the total benchmark return.
This is in contrast to the traditional Brinson Model, which calculates the sector allocation effect as (w_i – W_i)B_i, scaling the difference by the return of the benchmark sector.
This different calculation method allows for proper allocation effect calculation, regardless of whether the sector return is less than the overall benchmark return.
Institutional Portfolio Analysis
Institutional portfolio performance attribution analysis is a crucial aspect of understanding how your portfolio is performing. This analysis helps identify the drivers of your portfolio's return, which is essential for making informed investment decisions.
The basic construct of Brinson analysis is straightforward and can be reflected in a simple formula. The formula breaks down the total return of a portfolio into four components: selection effect, allocation effect, interaction effect, and the actual portfolio return.
Intriguing read: Attribution Analysis
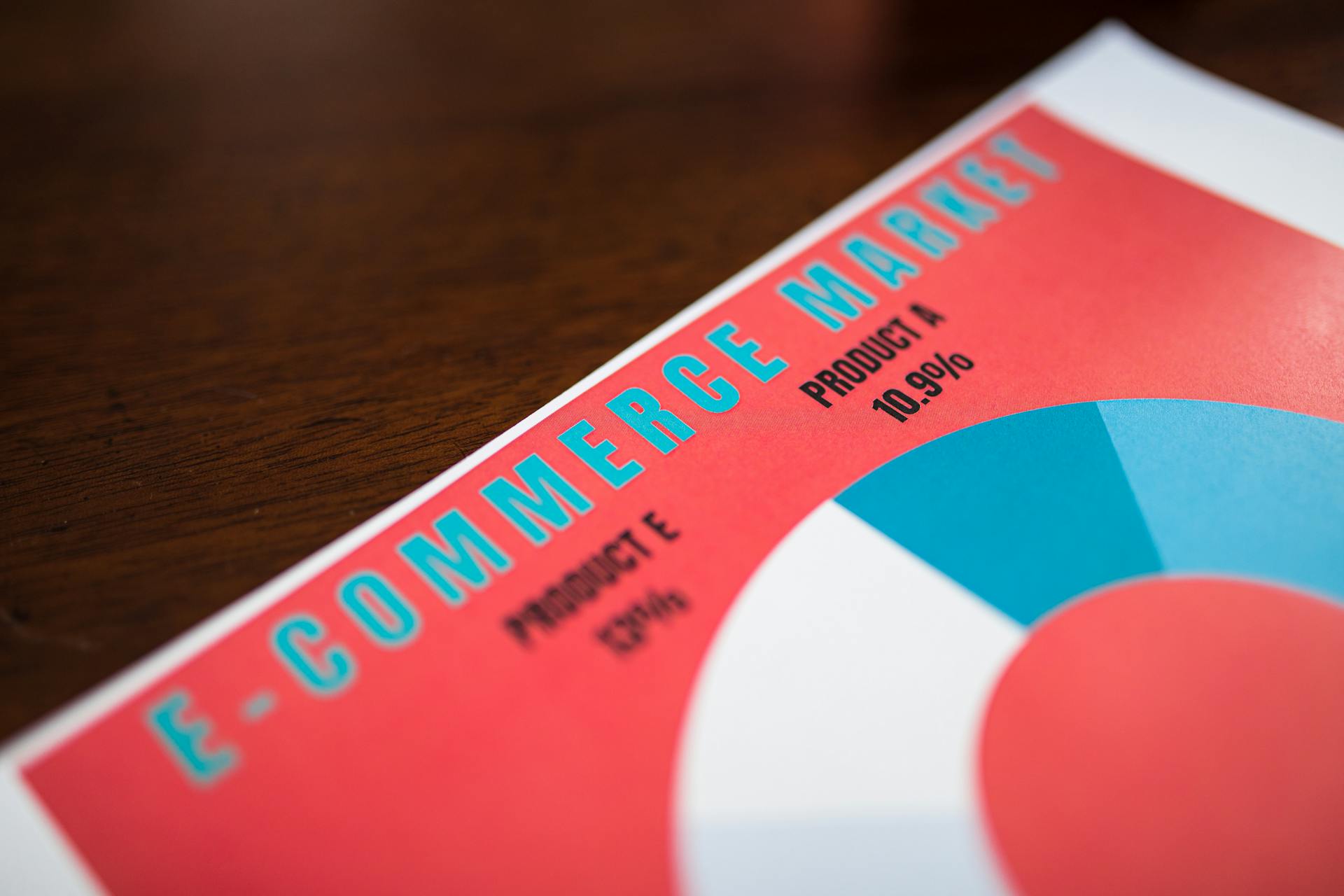
Institutional investment portfolios are typically constructed with a strategic asset allocation, which prescribes asset classes for investment, policy weights for each asset class, and policy benchmarks for each asset class. The portfolio benchmark is simply the sum of the policy weights times the policy benchmark returns.
The selection effect is the variation in return due to asset class returns in excess of benchmark returns. This effect is a critical component of Brinson analysis, as it helps investors understand how their asset class choices are impacting their portfolio's performance.
The allocation effect is the variation in return due to assets held in weights different from policy. This effect can have a significant impact on a portfolio's performance, as even small deviations from policy weights can result in substantial differences in returns.
The interaction effect is a blended effect of the selection and allocation effects. This effect is important to consider, as it can help investors understand how their asset class choices and weight deviations are impacting their portfolio's performance.
By breaking down the total return of a portfolio into these four components, Brinson analysis provides a clear and actionable understanding of a portfolio's return drivers. This information can be invaluable for investors seeking to optimize their portfolios and achieve their investment goals.
Consider reading: Balyasny Asset Management Performance
Case Studies
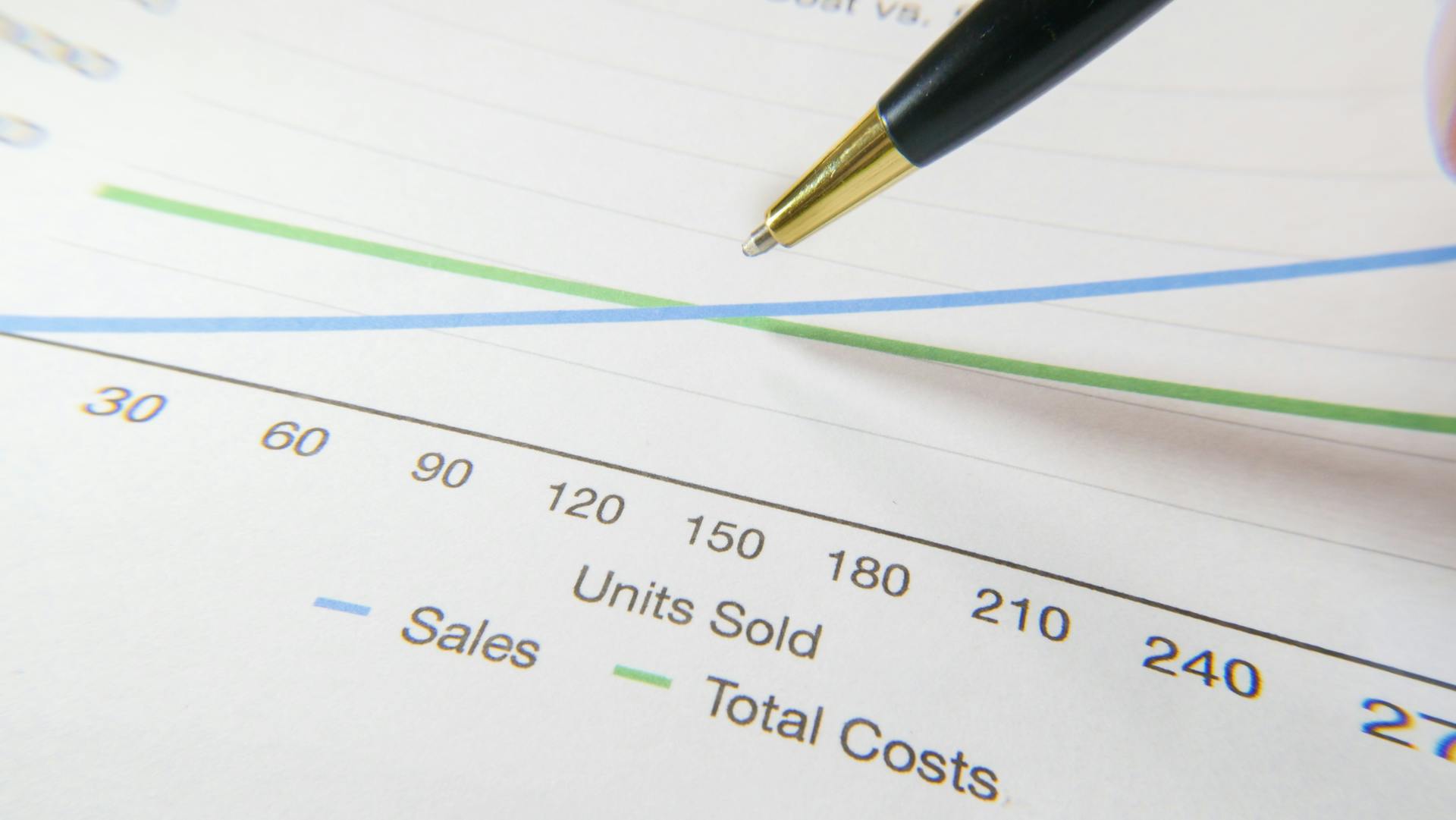
Let's take a closer look at a case study that compares Brinson and risk-based analysis on a popular dividend-focused ETF for the calendar year 2021.
The Brinson attribution method arrived at an active return of 0.03% for the ETF, but provided a limited explanation, attributing the return to sector allocation (-2.40%) and stock selection within each sector (2.43%).
This method didn't provide any insights into risk or additional exposures that drove investment decisions and the strategy's return.
In contrast, risk-based performance attribution, using the Axioma US Equity Factor Risk Model (US4), broke down the active risk and corresponding return from various factors, providing a more thorough view of return and risk.
The risk-based analysis revealed that factor returns contributed a positive 3.26% to return, while style factor exposures contributed 6.00% to return, with stock specific exposures detracting -3.24%.
The ETF's exposure to the Dividend Yield factor was 0.90, but given that it was a bad year for that factor (-1.10%), the exposure hurt performance, contributing -1.41% to the return.
Market sensitivity, or beta, was the main positive driver of performance, with a contribution of 5.73%, as low beta stocks fared better in 2021.
On a similar theme: Stock Splits Performance Post-announcement
Alternative Models
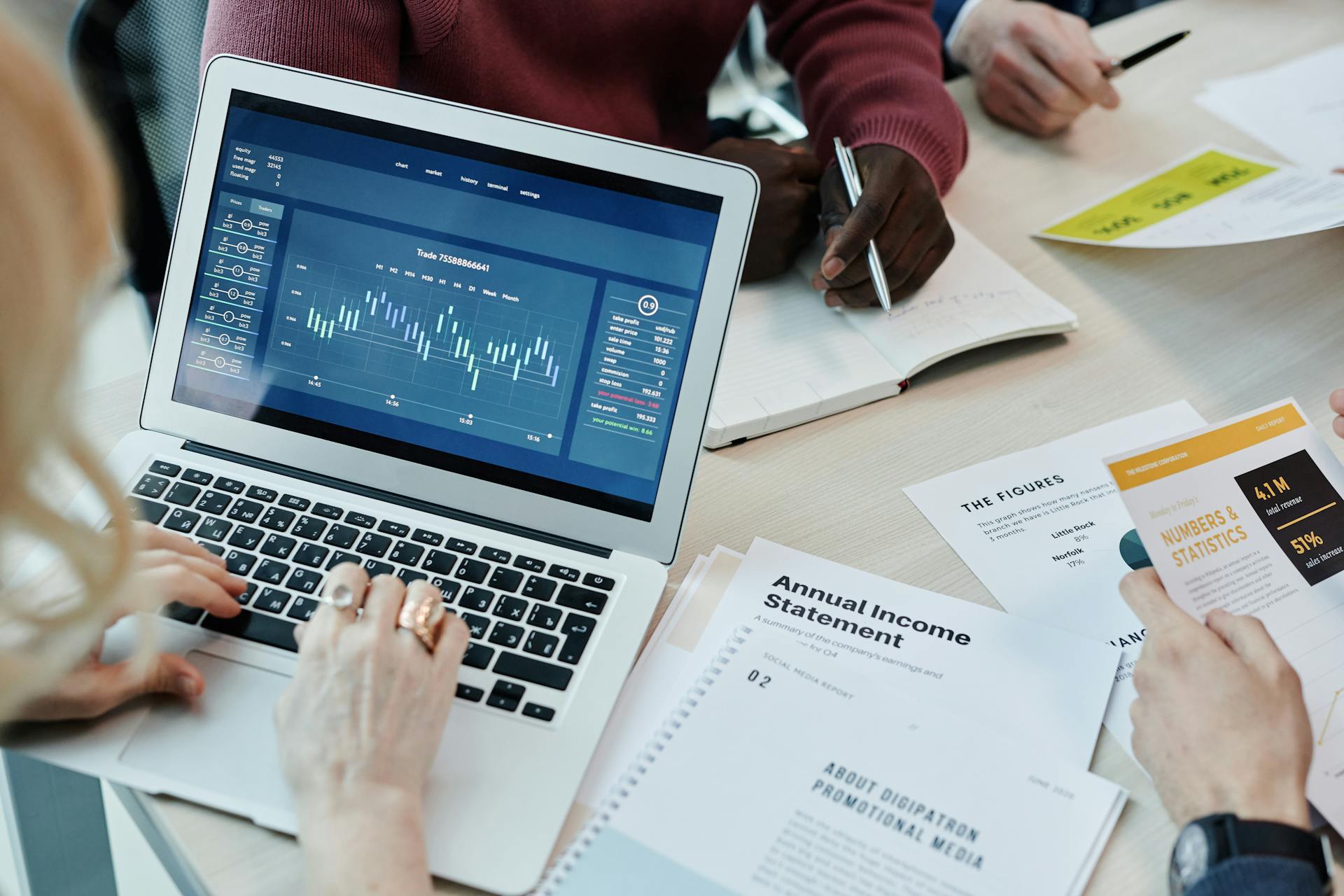
In the realm of Brinson performance attribution, alternative models have emerged to provide a more comprehensive understanding of investment performance.
One such alternative model is the Brinson-Fachler model, which extends the original Brinson model by incorporating additional factors such as cash flows and security selection.
This model is particularly useful for investors who want to gain a deeper understanding of the drivers of their portfolio's performance. The Brinson-Fachler model can help identify areas where an investor's portfolio is underperforming compared to a benchmark.
3 Multi-Factor Model
A multi-factor model is a more realistic way to apply performance attribution analysis, as it assumes multiple factors affect portfolio returns, such as size, value, momentum, and profitability.
These factors can be used to calculate the expected return of the portfolio and the benchmark based on their factor loadings, which measure their exposure to each factor.
A robust quantitative model that incorporates multiple return factors is vital to avoid misleading attestations.
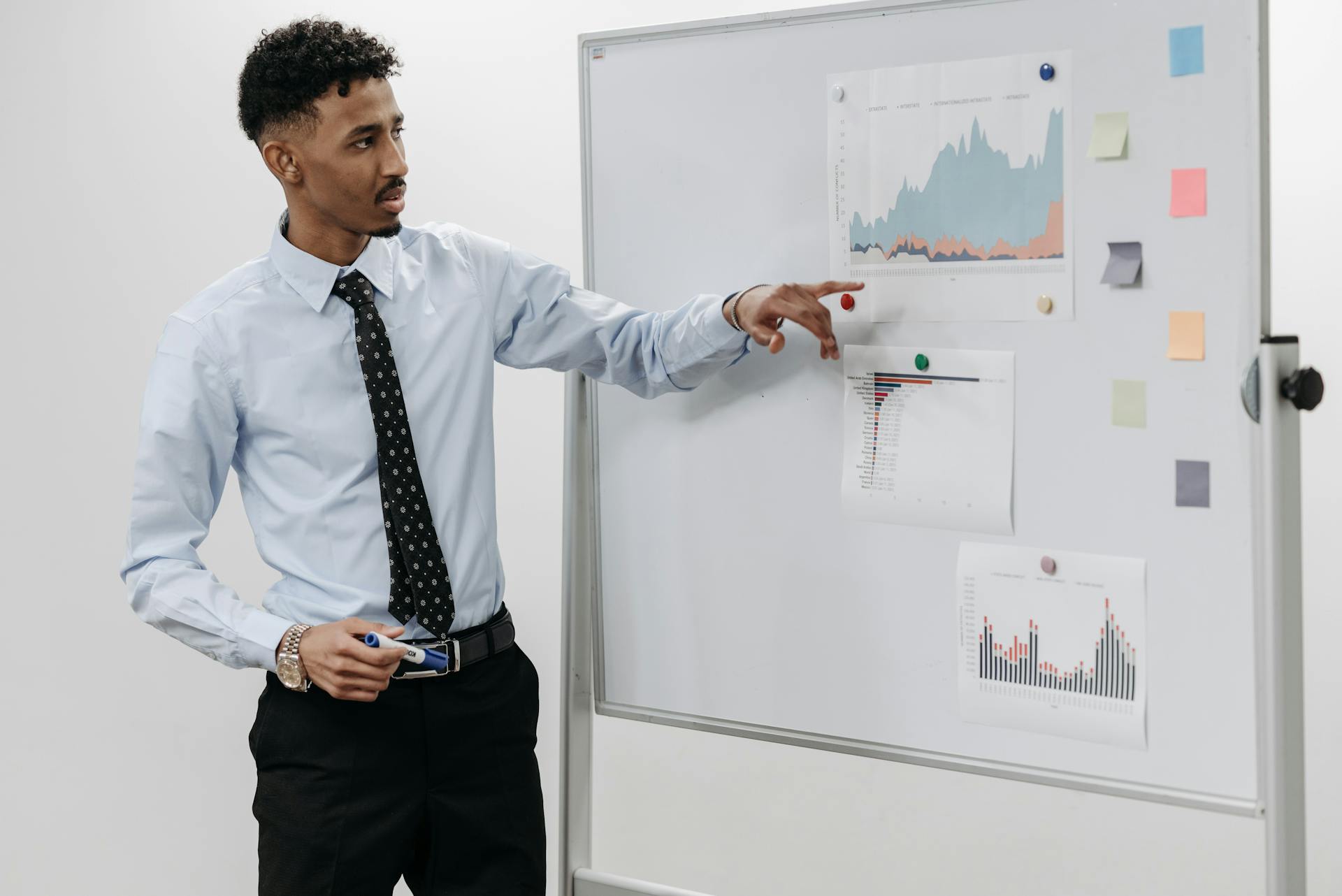
By analyzing momentum factor exposure, you can see if excess gains stem from riding hot streaks in certain securities.
The Fama-French three-factor model and the Carhart four-factor model are examples of multi-factor models that can be used for performance attribution analysis.
Here are some key characteristics of multi-factor models:
- They assume multiple factors affect portfolio returns.
- They use factor loadings to measure exposure to each factor.
- They can help identify the source of portfolio return through performance attribution.
Multi-factor models provide a fuller, more accurate picture of what is truly influencing portfolio performance, rather than relying on assumptions.
Nilay Parikh emphasizes the importance of multi-factor models in his LinkedIn post, stating that they enable data-driven insights into the specific dynamics actually moving the needle.
4 Model
The Brinson model is a popular way to apply performance attribution analysis, based on the sector or asset class level.
It assumes that the portfolio and the benchmark have the same set of sectors or asset classes, and that each sector or asset class has a representative index.
The Brinson model can be used to calculate the contribution of each sector or asset class to the portfolio and the benchmark returns based on their weights and returns.
The difference between the portfolio and the benchmark returns can then be attributed to the allocation, selection, and interaction effects across sectors or asset classes.
This model is useful for analyzing the performance of a portfolio and identifying areas where improvement is needed.
5 Advantages and Limitations
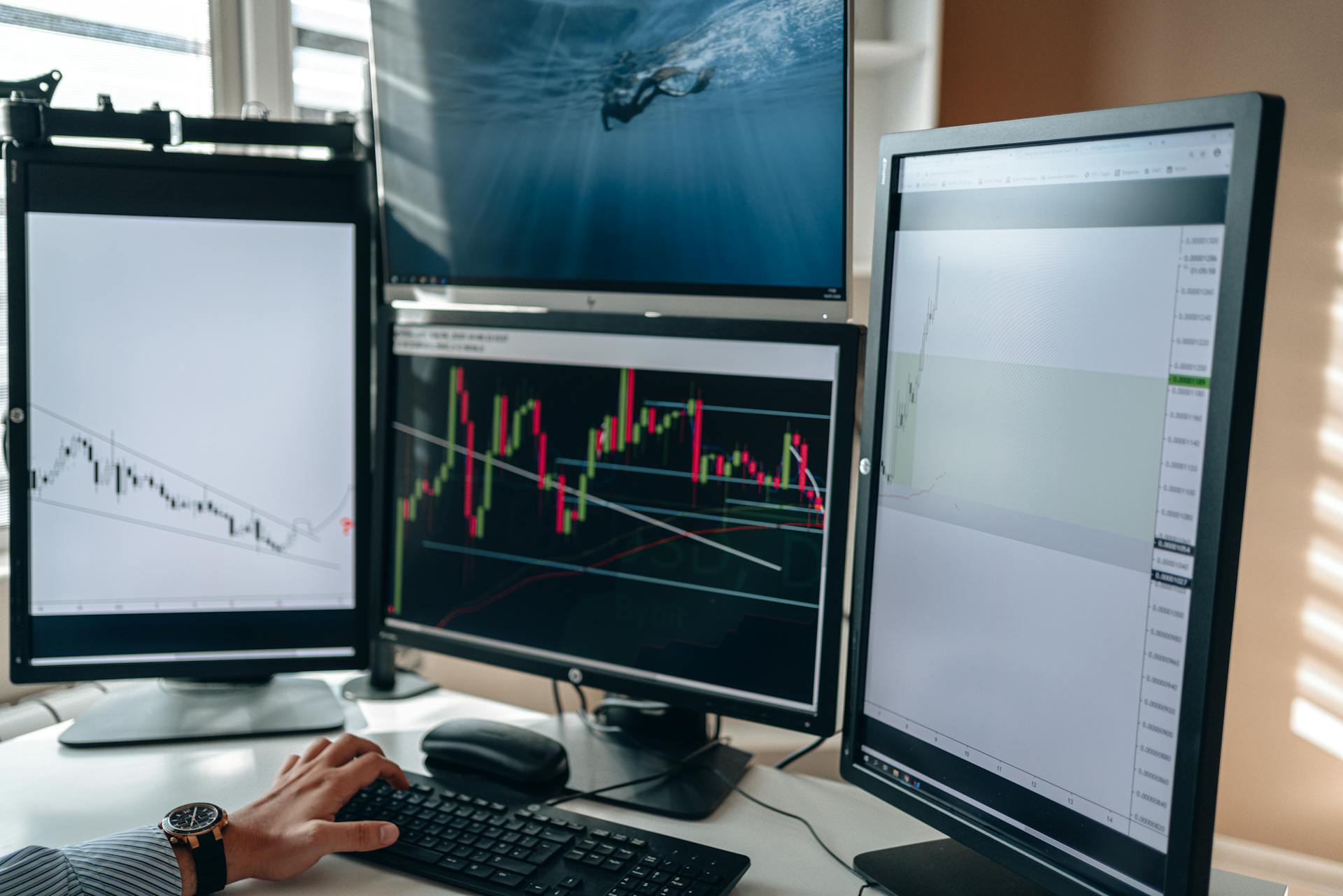
Alternative models offer a fresh perspective on traditional portfolio management, but like any tool, they have their advantages and limitations. Performance attribution analysis, a key aspect of alternative models, can provide valuable insights into the sources of portfolio returns.
It can help you identify your strengths and weaknesses, compare your performance with your peers, and adjust your portfolio allocation and selection accordingly. Performance attribution analysis can also be used to evaluate your portfolio strategy.
However, performance attribution analysis has some limitations. Data availability and quality are major concerns, as inaccurate or incomplete data can lead to flawed conclusions. Model specification and assumptions are also crucial, as they can significantly impact the accuracy of the analysis.
Interpretation and communication challenges are another limitation, as the results of performance attribution analysis can be complex and difficult to understand. This can make it hard to share your findings with others or make informed decisions based on the analysis.
A fresh viewpoint: Marketing Attribution Analysis
Quickly Understand Portfolio Returns with Simple Python
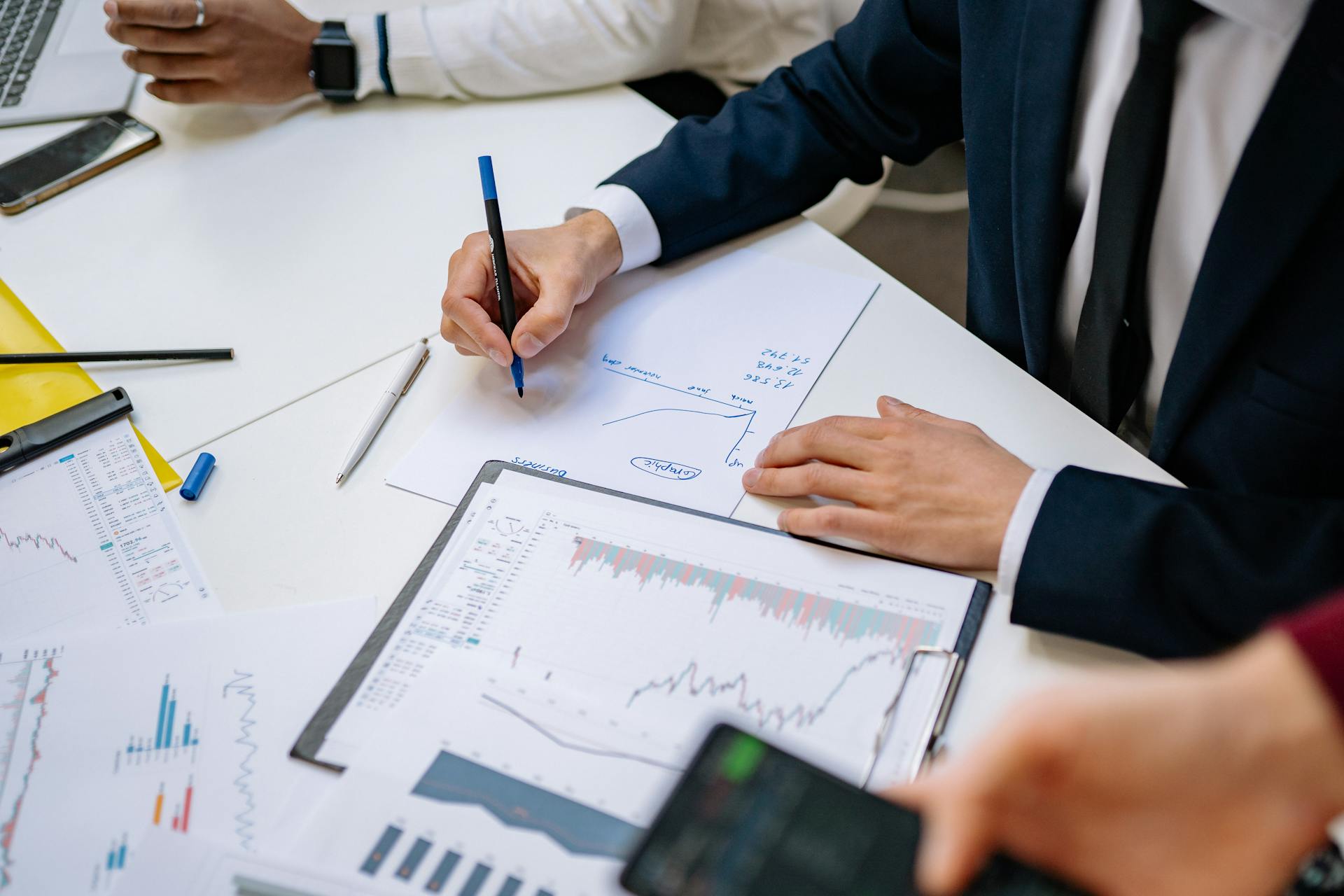
Understanding portfolio returns can be a daunting task, but with the right tools, it doesn't have to be. This simple Python implementation of Brinson Attribution helps quickly identify the drivers of your portfolio's return.
By using Brinson Attribution, you can break down your portfolio's return into its individual components, such as asset allocation and manager selection. This allows for a more nuanced understanding of your portfolio's performance.
With this Python implementation, you can easily analyze your portfolio's return drivers and make data-driven decisions. The code is straightforward and easy to follow, making it accessible to investors of all levels.
The Brinson Attribution model is a widely used method for performance attribution, and this Python implementation makes it easy to apply in practice. By leveraging this tool, you can gain valuable insights into your portfolio's return drivers and optimize your investment strategy.
Frequently Asked Questions
What is the difference between Brinson Fachler and Brinson Hood Beebower attribution models?
The Brinson-Hood-Beebower model extends the Brinson-Fachler model by adding an interaction effect, allowing for a more detailed decomposition of a portfolio's excess return. The main difference between the two models lies in their ability to account for the interaction between allocation and selection effects.
Sources
- https://www.simcorp.com/resources/insights/industry-articles/2024/Risk-based-or-Brinson-attribution
- https://insight.factset.com/how-a-multi-factor-attribution-framework-can-provide-a-deeper-insight-into-the-sources-of-relative-performance
- https://analystprep.com/study-notes/cfa-level-iii/sources-of-portfolio-returns/
- https://github.com/karlpolen/Brinson-attribution
- https://medium.com/off-script-systems/brinson-attribution-in-python-5e5a6265e708
- https://www.linkedin.com/advice/3/how-can-you-use-performance-attribution-analysis-ggwge
Featured Images: pexels.com