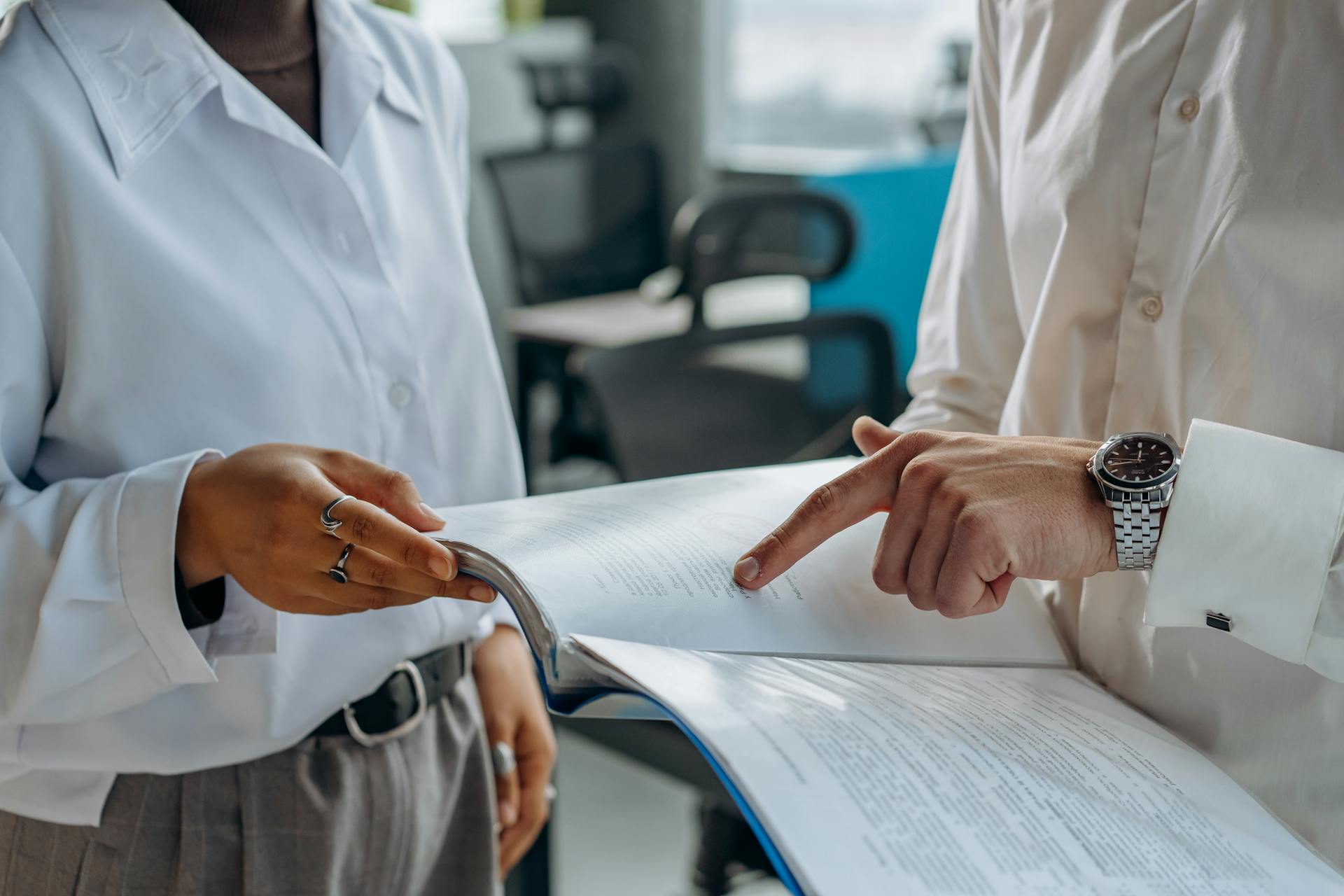
Anti money laundering machine learning is a powerful tool that helps financial institutions detect and prevent illicit activities. It's a crucial aspect of AML compliance.
Machine learning algorithms can analyze vast amounts of data to identify suspicious patterns and anomalies. This enables financial institutions to stay ahead of money launderers.
Effective AML compliance requires a multi-layered approach, including customer due diligence, transaction monitoring, and reporting suspicious activities. Machine learning plays a significant role in this process.
Financial institutions can use machine learning to identify high-risk customers, monitor transactions in real-time, and flag suspicious activities for further review.
Take a look at this: Machine Learning Algo Trading
The Role of Machine Learning
Machine learning plays a crucial role in anti-money laundering efforts. According to the United Nations Office on Drugs and Crime, money laundering amounts to 2-5% of global GDP, equivalent to $800 billion to $2 trillion in current US dollars.
This staggering amount of money laundered globally presents a significant challenge that machine learning technologies can help address. Machine learning enables the automation and analysis of vast volumes of data.
By detecting patterns, anomalies, and suspicious activities, machine learning empowers AML systems to identify potential money laundering. This automation reduces reliance on human operators to perform routine tasks.
Machine learning decreases the total triage time for alerts, allowing personnel to focus on more valuable and complex activities.
On a similar theme: Toronto Dominion Bank Money Laundering
Benefits and Advantages
Machine learning is revolutionizing the way we combat money laundering, and the benefits are numerous. Improved detection accuracy is one of the key advantages, with machine learning algorithms able to identify complex patterns and anomalies within large datasets.
Machine learning can improve detection accuracy by up to 40% and efficiency by up to 30%, as seen in leading financial institutions. This is because machine learning algorithms can continuously analyze data from multiple sources, including new and previously unidentified scenarios, and improve their accuracy over time.
One of the biggest challenges in AML compliance is the generation of numerous false positive alerts. Machine learning algorithms can help reduce false positives by analyzing and classifying data more accurately, resulting in a more efficient and focused investigation process.
Machine learning also allows for continuous, real-time analysis of transactions, automating the process of monitoring and detecting suspicious activities. This enables financial institutions to streamline operations, identify potential risks promptly, and allocate resources more effectively.
Here are some key benefits of machine learning in AML:
- Improved Detection Accuracy
- Enhanced Efficiency
- Reduced False Positives
- Adaptability to Evolving Tactics
Machine Learning Techniques
Machine learning techniques have proven invaluable in the field of Anti-Money Laundering (AML). They enable the automation and analysis of vast volumes of data, empowering AML systems to detect patterns, anomalies, and suspicious activities that may indicate potential money laundering.
Machine learning algorithms can analyze large datasets to identify patterns and detect suspicious activities, improving accuracy over time. Supervised learning algorithms, for example, can be used to classify subtypes of AML in large genomic data sets, revealing potential targets for therapy.
Unsupervised learning algorithms, on the other hand, analyze large volumes of transactional data to identify anomalies and detect potentially suspicious activities that may not have been previously labeled. They use clustering techniques to group similar transactions together and identify outliers that deviate from normal patterns.
For your interest: Types of Money Laundering Schemes
The Role of Technology in Various Industries
Machine learning techniques have far-reaching applications across various industries. In finance, AI and ML are used for fraud detection, credit scoring, algorithmic trading, and risk assessment.
The healthcare industry is also leveraging AI and ML to diagnose diseases, analyze medical images, predict patient outcomes, and discover new drug candidates. This has the potential to revolutionize the way we approach healthcare.
In transportation, AI and ML algorithms are being deployed in autonomous vehicles for perception, decision-making, and navigation tasks. This is a significant shift towards making transportation safer and more efficient.
E-commerce is another industry that is benefiting from AI, with personalized recommendations, chatbots for customer support, demand forecasting, and supply chain optimization being just a few examples. This enables businesses to better understand their customers and improve their overall shopping experience.
Here are some examples of the various industries that are using AI and ML:
- Healthcare: Diagnosing diseases, analyzing medical images, predicting patient outcomes, and discovering new drug candidates.
- Finance: Fraud detection, credit scoring, algorithmic trading, and risk assessment.
- Transportation: Autonomous vehicles for perception, decision-making, and navigation tasks.
- E-commerce: Personalized recommendations, chatbots for customer support, demand forecasting, and supply chain optimization.
- Marketing: Targeted advertising, customer segmentation, sentiment analysis, and predictive analytics for marketing campaigns.
These are just a few examples of the many ways in which AI and ML are being used across different industries. As technology continues to evolve, we can expect to see even more innovative applications in the future.
Deep
Deep learning is a subset of machine learning that involves the use of artificial neural networks with multiple layers to analyze and extract features from complex datasets.
Deep learning models can be trained to recognize patterns and anomalies in financial transactions, enabling more accurate identification of suspicious activity. This is particularly useful in AML, where intricate patterns may go unnoticed by traditional rule-based approaches.
By leveraging deep learning techniques, AML systems can analyze vast amounts of data and identify complex patterns that might go unnoticed by traditional rule-based approaches. This can significantly enhance the effectiveness of AML programs by reducing false positives and improving detection rates.
The adoption of machine learning techniques, including network modeling, clustering, and deep learning, is transforming the landscape of AML. These techniques enable financial institutions to better detect and prevent money laundering activities, enhancing their ability to safeguard against illicit financial transactions.
Deep learning models have the potential to significantly enhance the effectiveness of AML programs by reducing false positives and improving detection rates.
Readers also liked: Machine Learning
Enhancing Anti-Money Laundering Programs
Machine learning plays a significant role in enhancing Anti-Money Laundering (AML) programs by reducing false positives and enabling continuous monitoring and detection.
Traditional parameter-based transaction monitoring systems generate a high number of false positives, which can be time-consuming to investigate and divert resources from more critical tasks.
Machine learning algorithms can learn from historical data, identify patterns, and automatically refine detection rules, reducing the number of false positives while maintaining regulatory compliance.
By leveraging machine learning, financial institutions can identify suspicious activities more accurately and reduce unnecessary alerts, allowing compliance teams to focus on investigating genuine cases of money laundering.
Continuous monitoring and detection with machine learning enable financial institutions to stay ahead of sophisticated criminals and strengthen their AML defenses.
Machine learning algorithms can analyze vast volumes of data, effortlessly detect anomalies and suspicious behaviors as they occur, and adapt to new scenarios, making them more effective than traditional rule-based systems.
Curious to learn more? Check out: Types of anti Money Laundering
The integration of AI and ML technologies in AML compliance has revolutionized the way financial institutions combat money laundering, enabling advanced transaction monitoring, enhanced risk assessment, and automation of compliance processes.
Financial institutions can leverage machine learning to identify high-risk customers, entities, or transactions more effectively, allowing them to allocate resources based on the level of risk posed.
By continuously learning and adapting, machine learning models can stay ahead of evolving money laundering techniques and improve the accuracy of suspicious activity detection.
Consider reading: Bank Transaction Categorization Machine Learning
Real-World Applications and Case Studies
Machine learning has proven to be a game-changer in the fight against money laundering, with applications in transaction monitoring, customer due diligence, and fraud detection.
Several case studies showcase the successful implementation of machine learning in anti-money laundering (AML) programs. One leading financial institution demonstrated that implementing machine learning models in transaction monitoring improved suspicious activity identification by up to 40% and efficiency by up to 30%.
Machine learning algorithms can scrutinize vast amounts of financial transactions in real-time, rapidly analyzing patterns indicative of money laundering. This is critical in promptly identifying suspicious activities.
Financial institutions worldwide are harnessing machine learning technologies to combat money laundering more effectively. Machine learning models assess risk levels more accurately by analyzing multiple data sources, helping institutions better understand and manage customer risks.
United Overseas Bank (UOB) is a prime example of a successful implementation of machine learning in AML. They partnered with Tookitaki to integrate machine learning into their anti-money laundering program, aiming to establish a future-ready "Community-driven compliance model."
Machine learning also enhances customer due diligence processes by analyzing multiple data sources, revealing obscure patterns that manual methods would likely overlook. This is a significant improvement over traditional methods.
The adaptability and efficiency of machine learning models have proven indispensable in responding to emerging threats in financial crime. Financial entities can act swiftly to freeze accounts or flag suspicious transactions using machine learning algorithms that spot unusual activities faster than traditional methods.
Regulatory Support
Regulatory bodies in the United States are actively supporting the adoption of machine learning to combat financial crimes. Acts like the Anti-Money Laundering Act of 2020 and the National Illicit Finance Strategy are reducing obstacles and encouraging banks to embrace advanced technologies in their AML efforts.
Financial institutions and regulatory bodies are recognizing the potential of machine learning to revolutionize AML practices. By leveraging the power of machine learning algorithms, institutions can enhance their ability to detect and prevent money laundering.
The Anti-Money Laundering Act of 2020 is reducing obstacles for banks to adopt machine learning in their AML efforts. This act is fostering an environment that promotes the implementation of machine learning algorithms in AML.
Machine learning, combined with other advanced algorithms, can provide immediate and significant benefits in the fight against money laundering. These techniques, when applied to transaction monitoring, enhance the detection of suspicious activities and improve the efficiency of AML operations.
For more insights, see: Anti Selection
Challenges and Limitations
Traditional AML systems rely heavily on predefined rules, which can be rigid and slow to adapt, allowing criminals to exploit these limitations.
These systems also generate a high volume of false positives, overwhelming compliance teams with an excessive number of alerts, resulting in increased workloads and missed critical threats.
Static systems lack the ability to learn and evolve, restricting their effectiveness as money laundering tactics advance.
Criminals frequently exploit the limitations of traditional AML systems, making it crucial to adopt more advanced technologies.
Machine learning integration into AML frameworks presents challenges, particularly aligning machine learning capabilities with legacy systems that may lack flexibility.
Adapting to Evolving Tactics
Money launderers are constantly evolving their tactics to evade detection. This means that AML programs need to be adaptive and agile to keep up.
Machine learning algorithms excel in adapting to evolving threats because they can continuously analyze data from various sources, including previously unidentified scenarios.
Organizations can identify emerging patterns and behaviors that may indicate money laundering activities by leveraging machine learning.
Machine learning models can improve their own accuracy over time by learning from new trends and adjusting their detection capabilities accordingly. This ability to adapt is crucial in keeping up with sophisticated criminals.
Challenges and Limitations
Traditional AML systems are often rigid and slow to adapt, making them vulnerable to exploitation by criminals.
High volumes of false positives generated by these systems can overwhelm compliance teams, resulting in increased workloads and missed critical threats.
Static systems lack the ability to learn and evolve, restricting their effectiveness as money laundering tactics advance.
AI and Machine Learning technologies have significant potential to transform Anti-Money Laundering compliance, but there are several challenges and limitations to consider.
Integrating machine learning into AML frameworks can be challenging, particularly when aligning it with legacy systems that may lack flexibility.
Data quality and consistency are essential for successful integration, as machine learning models require access to clean, structured, and comprehensive data.
Strategic planning and collaboration can help overcome integration challenges, involving stakeholders from IT, compliance, and finance departments.
Integrating into Existing Frameworks
Integrating machine learning into existing AML frameworks is essential for modern financial institutions. This integration offers a strategic advantage by combining established practices with advanced technology.
Existing frameworks provide a foundation that can be enhanced with machine learning's analytical strength. Machine learning models can be seamlessly incorporated into existing systems to improve data analysis.
These models analyze transaction data and detect suspicious activities more accurately than traditional methods. This integration enhances the overall effectiveness and efficiency of AML operations.
Institutions must invest in robust data management practices to overcome the challenge of data quality and consistency. Machine learning models require access to clean, structured, and comprehensive data to function effectively.
A multidisciplinary approach that engages stakeholders from IT, compliance, and finance departments can help tailor machine learning solutions to fit seamlessly within existing AML systems. This collective effort ultimately enhances their capability to combat financial crimes.
By integrating machine learning with existing AML frameworks, financial institutions are better equipped to combat evolving financial crimes. This evolution ensures compliance with regulatory requirements and remains robust against emerging money-laundering tactics.
Future Outlook and Trends
The future of anti-money laundering (AML) machine learning is looking bright. Over 80% of heads of AML and financial crime from major North American banks have already started adopting machine learning solutions, with many more expected to follow suit in the next two to three years.
Financial institutions are investing heavily in compliance and risk management, with a staggering $214 billion spent on financial-crime compliance in 2020 alone. This significant investment is a testament to the industry's commitment to combating money laundering effectively.
Predictive analytics and AI advancements are at the forefront of AML innovation, enabling financial institutions to proactively combat financial crimes. By leveraging vast amounts of transaction data, they can anticipate suspicious activities before they occur.
AI can learn and adapt to new laundering tactics, continuously improving over time. This is particularly useful in detecting complex money laundering networks and uncovering hidden connections between individuals and entities.
Here are some emerging trends and advancements in AI and machine learning for AML compliance:
- Advancements in natural language processing and text analysis will enable better analysis of unstructured data sources such as news articles, social media posts, and internal memos.
- Leveraging network analysis and graph algorithms will enhance the detection of complex money laundering networks and uncover hidden connections between individuals and entities.
- The potential of blockchain technology in AML compliance is gaining attention, with its decentralized and immutable nature increasing transparency and traceability in financial transactions.
Frequently Asked Questions
Will AI replace AML?
AI is not replacing AML, but rather augmenting it with automated tools that support and complement human analysts. This collaboration enhances the effectiveness of AML processes
Sources
- https://financialcrimeacademy.org/machine-learning-algorithms-for-aml/
- https://www.tookitaki.com/compliance-hub/anti-money-laundering-using-machine-learning
- https://medium.com/@slsarath2/unlocking-the-power-of-machine-learning-in-transaction-monitoring-for-anti-money-laundering-f1c61305cd
- https://cloud.google.com/financial-services/anti-money-laundering/docs/concepts/overview
- https://www.kyrosaml.com/how-ai-and-machine-learning-are-transforming-aml-compliance/
Featured Images: pexels.com