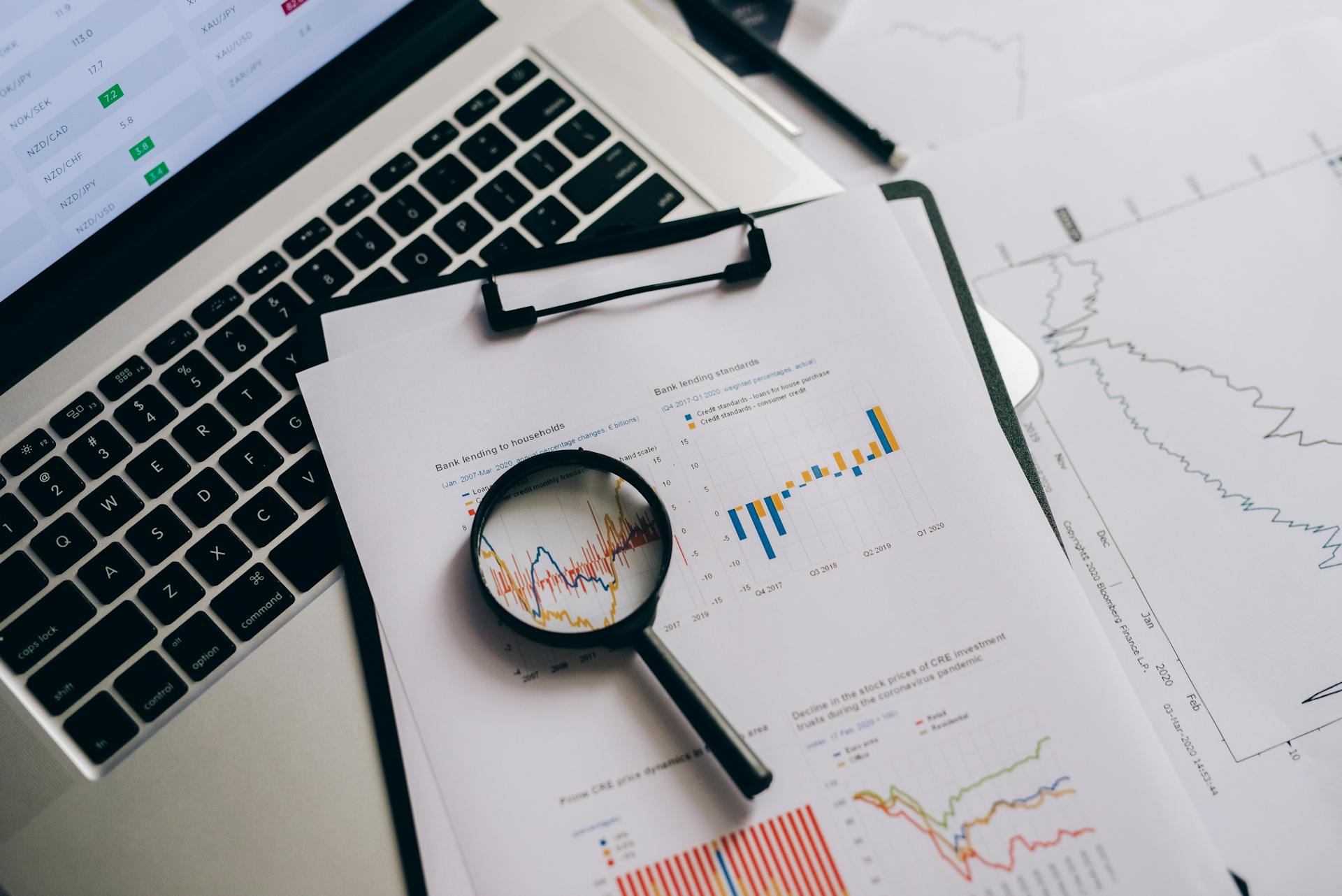
Sampling risk is a crucial aspect of audits and analytics that can have significant implications for businesses and organizations.
Sampling risk is the risk that the sample selected is not representative of the population, leading to inaccurate conclusions. This can occur when the sample size is too small or the sample selection method is flawed.
A key factor in sampling risk is the margin of error, which is the maximum amount by which the sample estimate may differ from the true population parameter. For example, if a sample of 100 items has a margin of error of 5%, the true population parameter may be up to 5% higher or lower than the sample estimate.
The margin of error is influenced by the sample size, with larger samples generally resulting in smaller margins of error.
What Is Sampling Risk?
Sampling risk is the possibility that the conclusions drawn from a sample may not accurately represent the entire population.
This risk is a concern because it can lead to inaccurate or misleading results, which can have serious consequences in various fields such as business, healthcare, and social sciences.
Sampling risk can occur when a sample is not representative of the population, meaning it doesn't accurately reflect the characteristics of the population being studied.
The potential for a sample to deviate from the true characteristics of the population being studied is a key aspect of sampling risk.
In other words, sampling risk is the risk that the sample doesn't give us a true picture of the population, which can lead to poor decision-making and incorrect conclusions.
This risk is often present in statistical analysis and data interpretation, where small samples can be misleading and not representative of the larger population.
Types of Sampling Risk
Sampling risk encompasses two primary types: Type I, which relates to the risk of incorrect rejection of a true hypothesis, and Type II, which involves the risk of failing to reject a false hypothesis.
Type I sampling risk, also known as alpha risk, occurs when a researcher erroneously concludes that there is a significant effect or difference when, in fact, none exists. This type of risk is an essential concept in hypothesis testing, as it relates to the probability of making a false rejection of the null hypothesis.
Type II sampling risk, known as beta risk, arises when a false hypothesis is not rejected, leading to a failure to identify an existing error and impacting the confidence level of the analysis. This risk is closely associated with hypothesis testing as it relates to the potential for accepting a null hypothesis that is actually false.
Type I sampling risk is an essential concept in hypothesis testing, as it relates to the probability of making a false rejection of the null hypothesis. Understanding and managing Type I sampling risk is vital for maintaining the integrity and accuracy of statistical analyses.
Type II sampling risk affects the confidence level by increasing the likelihood of not detecting a significant difference or effect when one truly exists. It emphasizes the potential for errors in decision-making, making it a critical concern in statistical analysis.
Factors Affecting Sampling Risk
Sampling risk is affected by several key factors, including the sample size. A larger sample size generally reduces sampling risk.
High population variability can increase the risk of obtaining unrepresentative samples, affecting the generalizability of the findings. This can be a major concern in research where accuracy is crucial.
The sampling method employed also plays a crucial role in determining sampling risk. Convenience sampling, for instance, may introduce bias, whereas random sampling aims to minimize such errors.
A larger sample size generally yields higher confidence levels and greater accuracy in representing the overall population. This is due to the reduced margin of error resulting from a more comprehensive data set.
It's essential to strike a balance when determining the sample size, as an excessively large sample size can lead to unnecessary costs and time consumption.
Mitigating Sampling Risk
Increasing the sample size is a fundamental strategy for reducing sampling risk. It enhances the confidence level and statistical significance of the analysis, mitigating potential errors and uncertainty.
A larger sample size generally yields higher confidence levels and greater accuracy in representing the overall population. This is due to the reduced margin of error resulting from a more comprehensive data set.
By prioritizing high-risk areas, auditors can allocate their resources more efficiently and effectively, thereby reducing overall sampling risk. This risk-based approach is a crucial aspect of mitigating sampling risk.
A larger sample size helps mitigate the impact of outliers and variability, leading to more reliable findings. It is essential to strike a balance as an excessively large sample size can lead to unnecessary costs and time consumption, without significantly improving the confidence level or accuracy.
Continuous monitoring and reassessment of the sampling plan throughout the audit process are also essential. This dynamic approach allows auditors to adjust their strategies in response to new information or emerging risks, ensuring that the audit remains robust and comprehensive.
Larger sample sizes provide greater statistical power, enabling researchers to detect smaller effects or differences. With a larger sample size, the likelihood of observing a significant effect is amplified, making the findings more robust and dependable.
Measuring and Minimizing Sampling Risk
Sampling risk is calculated through the assessment of statistical significance, variance, mean values, and standard deviation, which collectively contribute to determining the level of uncertainty and potential errors in the analysis.
A larger sample size generally yields higher confidence levels and greater accuracy in representing the overall population.
Sampling risk is measured by the margin of error or confidence interval, which is the range of values within which the true population parameter is estimated to fall, with a certain level of confidence.
Increasing the sample size is a fundamental strategy for reducing sampling risk, as it enhances the confidence level and statistical significance of the analysis, mitigating potential errors and uncertainty.
To minimize sampling risk, it is essential to ensure that the sample is truly representative of the population, which can be achieved by using random sampling methods and increasing the sample size.
Validating results through the use of multiple samples enhances the accuracy and reliability of the analysis, reducing uncertainty and potential errors associated with sampling risk.
Analyzing different subgroups within the sample aids in understanding variability and potential biases, contributing to a more comprehensive assessment that reduces sampling risk in hypothesis testing and data analysis.
Sampling risk holds significant importance in analytics as it influences risk assessment, introduces uncertainty and potential errors, and impacts decision-making processes based on sampled data.
Importance and Considerations
Sampling risk is a crucial factor in assessing the validity of conclusions drawn from sample analysis.
It's influenced by factors such as sample size, population variability, and the sampling method employed. The sample size is a critical factor, as a small sample may not accurately represent the entire population.
Sampling risk can lead to incorrect conclusions and decisions, emphasizing the importance of careful consideration and mitigation measures to reduce its impact on the overall analytical process.
Decision makers can have more confidence in the reliability of their data and make more informed choices for their business by understanding and managing sampling risk.
The presence of sampling risk can introduce uncertainty and potential errors, which can have significant impacts on the accuracy of insights and decisions made based on those insights.
Real-World Applications
Sampling risk can be a major concern in various industries.
In finance, sampling risk can lead to inaccurate estimates of expected returns, which can result in poor investment decisions.
For example, a hedge fund manager may use a sample of stock prices to estimate the average return of a portfolio, but if the sample is not representative of the entire population, the estimate may be skewed.
In quality control, sampling risk can affect the reliability of inspection results, leading to defective products being released to the market.
For instance, a manufacturer may use a sample of products to determine if they meet quality standards, but if the sample is too small or not representative of the entire production run, the results may be inaccurate.
Sampling risk can be mitigated by using larger and more representative samples, as well as by using statistical methods to account for sampling error.
What Is an Example in Analytics?
In A/B testing and market research surveys, sampling risk is a real concern that can lead to errors and uncertainty in analysis. A larger sample size can help reduce sampling risk, providing a more accurate representation of the population.
Using a smaller sample size can increase sampling risk due to higher variability and potential for bias. This is especially true in A/B testing scenarios.
The choice of sampling method, such as random, stratified, or cluster sampling, can greatly impact sampling risk in market research surveys. Selecting the wrong method can introduce bias and decrease the generalizability of results.
A larger sample size can help reduce sampling risk in A/B testing, but it's not the only factor at play. The type of sampling method used can also have a significant impact on the accuracy of the results.
Real-World Audits
In real-world audits, the quality of an audit hinges significantly on the effectiveness of the sampling methods employed.
Statistical and non-statistical sampling methods play a pivotal role in shaping the audit's outcome. The choice between the two can be a game-changer.
The auditor's judgment is a critical factor in both types of sampling, and even with sophisticated statistical tools, experience and understanding of the business context are indispensable.
An auditor's ability to identify high-risk areas based on past audits or industry knowledge can significantly enhance the effectiveness of the sampling process.
The reliability of audit findings can be compromised if sampling risk is not adequately managed, leading to potential misstatements in financial reports.
Compliance
Compliance is a top priority for auditors, and managing sampling risk is a crucial aspect of this process. Technological tools have revolutionized sampling, offering auditors sophisticated methods to enhance precision and reliability.
Automated random sampling tools like Audit Command Language (ACL) and IDEA generate random samples from large datasets, ensuring that the selection is free from human bias and representative of the entire population.
The use of data analytics software allows auditors to sift through vast amounts of data, identifying transactions that deviate from the norm and warrant a closer look. This can lead to the detection of material misstatements and other potential issues.
Computer-Assisted Audit Techniques (CAATs) automate the testing of controls and transactions, making them especially useful in repetitive tasks and handling large volumes of data. CAATs can help flag transactions that exceed a certain threshold or occur with unusual frequency, indicating potential fraudulent activity.
Stratified sampling enables auditors to divide a population into smaller groups based on specific characteristics, ensuring that samples are collected from each segment and leading to more accurate results. This is particularly useful when dealing with diverse populations or complex data sets.
By leveraging these technological tools and techniques, auditors can significantly reduce the margin of error and ensure a more robust audit outcome.
Sources
- https://en.wikipedia.org/wiki/Sampling_risk
- https://www.aphis.usda.gov/plant-imports/risk-based-sampling
- https://accountinginsights.org/managing-sampling-risk-in-audits-techniques-and-technology/
- https://www.bizmanualz.com/library/what-does-sampling-risk-mean
- https://www.fastercapital.com/content/Sampling-Risk--Calculating-Caution--Understanding-Sampling-Risk-in-Audit-Procedures.html
Featured Images: pexels.com