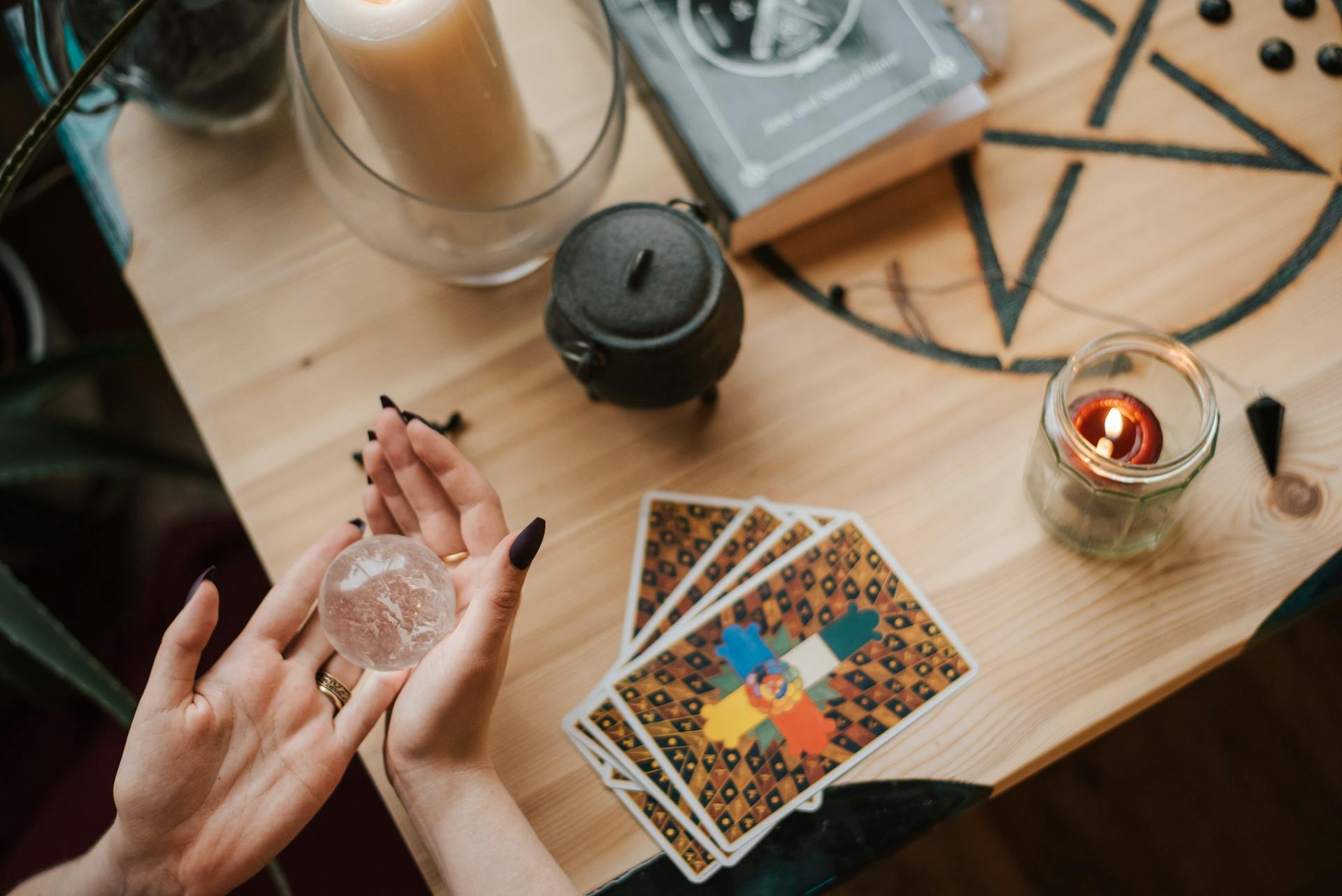
Predictive risk modeling is a powerful tool for financial and operational management, allowing businesses to anticipate and mitigate potential risks.
By leveraging advanced analytics and machine learning algorithms, companies can identify patterns and correlations that may indicate a risk, such as a sudden increase in customer complaints or a spike in inventory levels.
This proactive approach enables businesses to take preventive measures, reducing the likelihood and impact of a risk event.
Businesses can also use predictive risk modeling to optimize resource allocation and improve operational efficiency, by identifying areas where costs can be reduced or processes can be streamlined.
You might enjoy: Compliance and Operational Risk Analyst
What is Predictive Risk Modeling?
Predictive risk modeling is a powerful tool that uses complex algorithms to analyze data and make predictions about potential risks. These algorithms can range from simple linear regressions to sophisticated neural networks.
The accuracy of predictive models depends on the quality of input data, which must be comprehensive, clean, and relevant. In other words, the data needs to be gathered from a wide array of sources within the organization.
Readers also liked: Compas Risk Scales: Demonstrating Accuracy Equity and Predictive Parity
To ensure trustworthy predictions, predictive models must be both calibrated and validated. Calibration involves fine-tuning the model parameters to align predictions with known outcomes.
High-quality data is the foundation upon which all predictive modeling rests. This means that data must be accurate and reliable in order to make informed decisions.
Predictive risk modeling is a key element of an enterprise risk management (ERM) framework, which underpins the credibility of the model's risk predictions and the subsequent decisions made by the business.
Additional reading: Altcoin Predictions
Best Practices for Business Modeling
To build effective predictive models, you need to start with a solid foundation of quality data. This involves collecting and aggregating a broad range of data points that are critical for accurate enterprise risk management.
Collaboration between business leaders and data scientists is crucial for the success of predictive modeling. Data scientists bring the analytical expertise needed to construct complex models that can filter through vast quantities of data.
Worth a look: Community Banking Models
The precision in gathering and processing data is what dictates the efficacy of the predictive models. Organizations need to establish robust data management systems to ensure the accuracy of their models.
Continuous review and adjustment of predictive models is necessary due to the dynamic nature of business risks. As new data becomes available and the business environment evolves, models need to be recalibrated to maintain their accuracy.
Broaden your view: Characteristic Nonrational Models
Navigating Challenges
Predictive risk modeling is a powerful tool, but it's not without its challenges. One of the biggest hurdles is data privacy and ethics.
Adhering to data protection regulations, such as GDPR, and ethical guidelines is paramount to maintain trust and integrity in the predictive modeling process. Businesses must establish strict data governance policies to protect individual privacy rights while leveraging data for risk assessment.
Predictive models are tools to aid decision-making, not replace it. Human oversight is crucial to interpreting model outputs correctly and applying them within the broader context of organizational risk methodology.
Suggestion: What Does Ibnr Stand for
The technology underpinning predictive modeling is advancing at a rapid pace, requiring businesses to stay updated with the latest developments in AI and machine learning. This ensures the business risk strategy harnesses the full potential of predictive modeling.
Continuous learning and adaptation are required to keep the corporate risk framework aligned with the latest technological capabilities and ERM guidelines.
Financial Management
Financial management is a crucial aspect of any business, and predictive risk modeling can help you stay on top of potential financial risks. Predictive analytics for financial risk management can identify issues such as extended credit, interest rate fluctuations, or debt load, which can lead to cash flow harm or unexpected losses.
The United States Treasury Department has recently issued funds for financial risk assessment for the DeFi sector, highlighting the importance of financial risk management. This type of risk assessment is valuable for any industry, including retail, software development, supply chain, manufacturing, insurance, and more.
Intriguing read: Risk Assessment Process
To implement predictive risk analytics in your business, start by listing all potential risks in a library before getting started with analysis. Then, check and test data sources to identify and validate key risk indicators.
Here are the easy steps to implement predictive risk analytics in your business:
- List all potential risks in a library before getting started with analysis.
- Check and test data sources to identify & validate key risk indicators.
- Automate testing to raise issues directly to the management.
- Use visualizations to check and evaluate insights against risks.
- Keep analyzing and reporting insights regularly.
By following these steps, you can stay ahead of potential financial risks and make informed decisions to protect your business.
Operational Management
Operational risk can be a major concern for businesses, and it's essential to have a solid plan in place to mitigate it. A recent survey revealed that cyberattacks are the primary influential factors behind rising operational risks among mid-level enterprises and big firms.
Unexpected occurrences such as natural disasters, damage to your building, a server outage, or even an employee blunder can all be classified as operational risk. It can also be considered a sub-set of financial risk.
Big data management and predictive analytics can help you deal with operational risk together. This approach can help you identify potential risks and take proactive measures to prevent them.
For more insights, see: Operational Risk Management Process
A business plan failing to yield the desired results refers to a strategic risk, which can also be managed using predictive analytics. This can help you catch risks with any strategy or plan which is not working as expected.
Integrating risk management solutions through predictive analytics helps organizations learn from past adverse incidents. This can enable you to develop a more effective risk management strategy.
For more insights, see: Currency Analytics
Frequently Asked Questions
What are three 3 examples of predictive models?
Here are three examples of predictive models: Regression, Neural Network, and Decision Tree, which are used to forecast continuous values, complex patterns, and categorical outcomes respectively. Each model has its unique strengths and applications, making them essential tools in data analysis and machine learning.
What are the three risk modelling methods?
Risk modeling methods include quantitative, qualitative, and hybrid approaches, each using different techniques to predict potential risks. These methods help organizations make informed decisions by analyzing and mitigating potential risks.
Sources
- https://www.certa.ai/blogs/predictive-modeling-the-future-of-enterprise-risk-assessment
- https://www.bluent.com/blog/predictive-analytics-for-risk-management/
- https://blog.famcare.net/predictive-risk-modeling
- https://www.ucviden.dk/en/publications/predictive-risk-modelling-as-a-tool-for-supporting-decision-makin
- https://www2.deloitte.com/us/en/pages/regulatory/articles/using-predictive-operational-risk-analytics-data.html
Featured Images: pexels.com