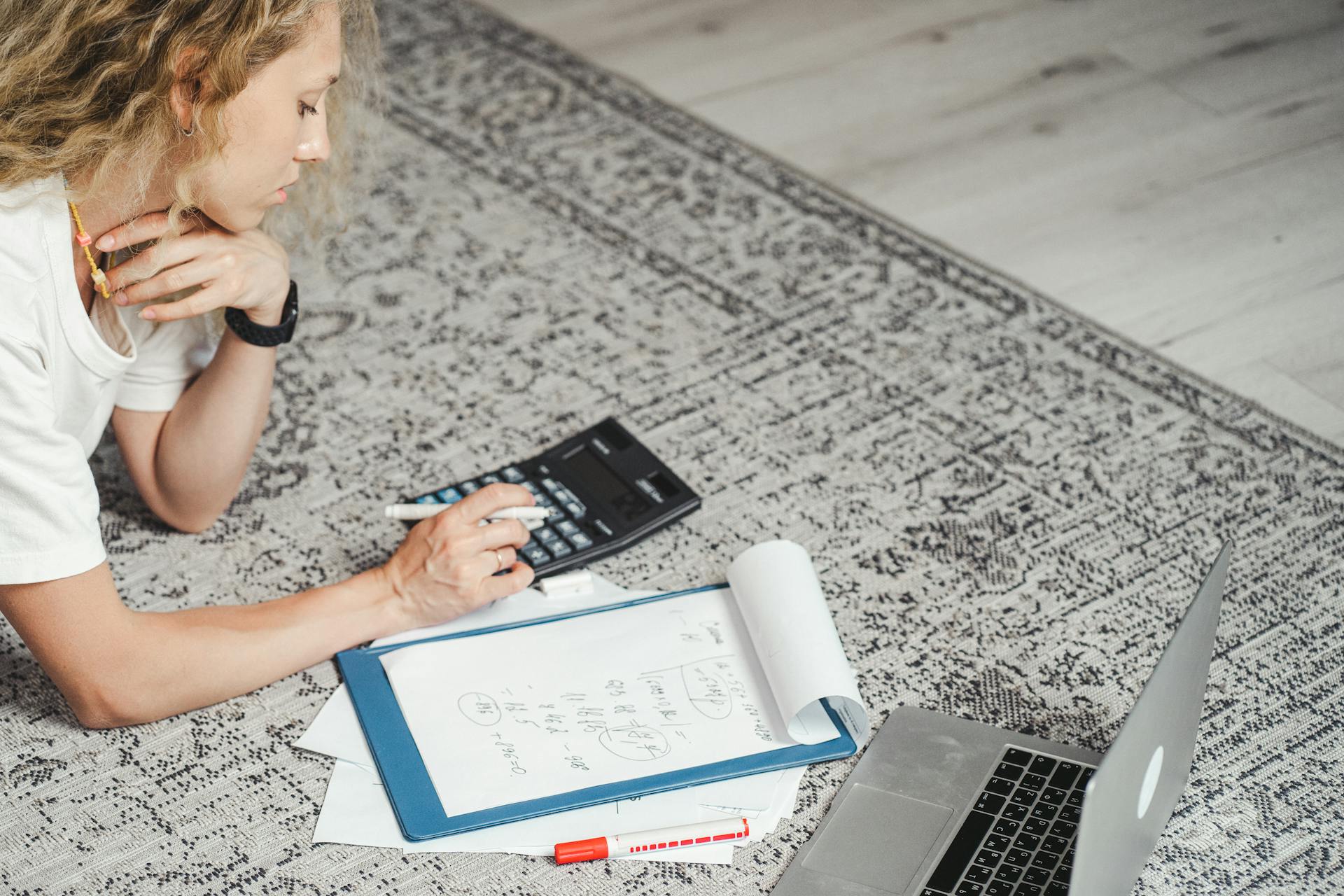
Mortgage analytics can help lenders and investors make informed decisions by providing a detailed understanding of loan performance.
With the right tools and data, lenders can identify trends and patterns in loan behavior, such as the average loan-to-value ratio of 80% mentioned in the article.
This information can be used to adjust lending strategies and improve loan quality.
By analyzing loan performance data, lenders can also identify areas for improvement, such as reducing delinquency rates.
For example, a lender may find that loans with higher credit scores have significantly lower delinquency rates, as seen in the article's discussion of credit score thresholds.
This insight can be used to adjust underwriting guidelines and improve loan quality.
A different take: Which Credit Score Is Used for Mortgage Loans
What Is Yield Book Advanced?
Yield Book Advanced is a comprehensive mortgage analytics platform that helps lenders make informed decisions. It provides a single view of the entire mortgage pipeline, from origination to servicing.
Mortgage lenders can use Yield Book Advanced to analyze loan performance, identify trends, and optimize their portfolios. This platform offers advanced analytics and reporting capabilities.
Broaden your view: Currency Analytics
The platform's data analytics capabilities enable lenders to segment their portfolios by risk, geography, and other factors. This allows them to make data-driven decisions and mitigate potential risks.
Yield Book Advanced also provides real-time reporting and dashboards to help lenders stay up-to-date on their mortgage portfolios. This enables them to quickly identify areas of improvement and make adjustments as needed.
By leveraging Yield Book Advanced, mortgage lenders can gain a deeper understanding of their mortgage portfolios and make more informed decisions.
Check this out: Mortgage Yield
Mortgage Analytics Solutions
Mortgage analytics solutions are all about combining robust data with smart analytics to minimize mortgage fraud and reduce regulatory compliance risk. This can be achieved by spanning the entire loan life cycle with mortgage analytic solutions.
The key to implementing operational mortgage analytics is to understand what questions you want to answer and define what data you'll need to create the charts and visualizations that will give you the answers. This involves breaking down the process into several steps, including defining what charts and visualizations will answer the questions.
Here's a quick rundown of the tech used in operational analytics:
- Database for the entities (e.g., MongoDB, PostgreSQL, etc.)
- Database for the events (e.g., ClickHouse, Swnoflake, etc.)
- Event streaming technology (e.g., Kafka)
- Event processing technology (e.g., Decodable)
- BI/Reporting software (e.g., Streamlit, Mode, etc.)
Loan-Level Includes
Our mortgage analytics solutions have access to a vast amount of data, including over 30 million loan applications.
This extensive dataset allows us to provide detailed insights into the mortgage market. We can analyze trends and patterns that might otherwise go unnoticed.
One of the key benefits of our loan-level data is its comprehensiveness. It covers about 750,000 reviewed mortgage loans, giving us a thorough understanding of the market.
Here's a breakdown of the loan-level data we have access to:
- Over 30 million loan applications
- About 750,000 reviewed mortgage loans
- 99% of total deeds, mortgages and foreclosures covering more than 3,000 counties
- PACE Lien Data
- Fraud indices dating back to 2003
Property Include:
Our property datasets are comprehensive and cover a significant portion of the US market. We have assessor/property ownership data for 100% of the US market, giving you a complete picture of property ownership.
Tax data is also available for 100% of the US market, providing you with a thorough understanding of tax-related information. Homeowner associations are covered in our dataset, with 99% of HOAs and COAs included.
We have access to 1,500+ title plants, which is a significant resource for title-related information. Assignments and releases are also included in our dataset, with 96% of the US market covered.
Real estate listings are available for 85% of the market, giving you a robust source of listing data.
A unique perspective: What Is Equity in Home Ownership
Credit Analysis Matters
As the U.S. economy reaches the later stages of an expansion phase, it's essential to study credit trends and identify opportunities.
The GSE patch is set to expire in 2021, which means certain credit profiles will no longer be eligible for agency securitisation. This change will impact the mortgage industry significantly.
The GSE Non-QM Patch has a DTI boundary line universe, indicating that credit profiles with high debt-to-income ratios may not be eligible for agency securitisation.
This shift in the GSE patch's expiration date creates a window of opportunity for lenders and investors to adapt and find new ways to securitize mortgages.
Curious to learn more? Check out: Will Lender Accept If a Friend Gift Money Conventional Loan
Available in U.S. Secondary Market
Mortgage analytics solutions are making a huge difference in the industry, and one of the key areas where they're having an impact is in the U.S. secondary market. With access to indicative transactional mortgage data, mortgage capital markets professionals can make more informed decisions.
We can quickly and efficiently identify fraud risk and errors in mortgage applications early in the application review process, which is a huge time-saver. This is especially important in today's fast-paced mortgage market.
A unique perspective: How to Market to Realtors as a Loan Officer
MCT aggregates mortgage analytics and provides access to a vast amount of mortgage data, making it easy to stay on top of market trends. By monitoring liquidity in the servicing market, mortgage professionals can gain a clearer view of the market and make more informed decisions.
Buyers of non-banking servicing can quickly get the information they need with our easy-to-use platform, which provides data by transfer type, program, and state. This level of detail is invaluable for making informed investment decisions.
With our mortgage analytics solutions, you can quickly perform research on any element of a property or properties in a loan application and create a single view report. This saves time and reduces the risk of errors.
Key Benefits and Features
Mortgage analytics has revolutionized the way lenders operate, making the process more efficient and accurate.
Advanced analytics in mortgage lending reduces the time required to extract meaningful data and enables real-time decision making.
Curious to learn more? Check out: Usda Loan First Time Home Buyer
Mortgage lenders can automate their processes and make informed decisions faster, thanks to advanced analytics.
Our web-based application simplifies the complex data transformation process, making it effortless to access trusted big data analytics.
Mortgage data is big, dirty, and difficult to analyze, but our application makes it easy to transform and interrogate.
With advanced analytics, mortgage capital markets professionals can protect market share, identify opportunities, and better understand risk.
Large volumes of data are disseminated inconsistently at varying frequencies, but our application helps to transform and analyze this data.
We combine our expertise in property data with mortgage analytic solutions to minimize mortgage fraud and reduce regulatory compliance risk.
Our solutions span the entire loan life cycle, from origination to due diligence and market condition detection.
Quickly identifying fraud risk and errors in mortgage applications is crucial, and our application helps to do just that.
We also provide loan-level compliance reviews, background checks, and 100% nationwide property tax records with the most current tax status.
Calculating the value of a home is now easier and more accurate, thanks to our low-cost, consistent, and accurate lender-grade AVM.
Data and Analysis
To analyze mortgage performance, you'll want to dig into detailed reporting compared to your peer group. This includes looking at metrics like landing page engagement, loan locks, and sales.
To get started, you'll need to understand what questions you want to answer, such as what's driving loan locks or how your sales compare to the competition. Define the charts and visualizations that will help you answer these questions.
Key data sources include databases for entities like customers and events like loan applications. You'll also want to collect data on loan performance, such as interest rates and loan terms.
Here's a rundown of the types of data you'll need to collect:
- Landing page engagement metrics
- Loan lock and sale data
- Interest rates and loan terms
- Peer group benchmark data
Pinpoint Hidden Prepayment Stories
Historically low interest rates have changed the way borrowers prepay their mortgages. This shift is making it harder for econometric models to predict prepayment behavior accurately.
The rise of non-banks and paperless mortgage processes is also contributing to the unpredictability of prepayments. This is where data-driven forecasts can help fill the gap and provide more accurate predictions.
Our data is machine-learning ready, allowing you to easily export it in a tabular format and apply algorithms to isolate duration profiles. This helps ensure the recovery of premium paid to own the bond.
In traditional Mortgage Analytics, historical data is used to identify trends and make predictions. However, this approach can be too retrospective and focused on long-term patterns rather than immediate operational improvements.
Representative Sample
MCT represents a significant portion of the US secondary mortgage market, with investor partners estimating its volume to be between 22% and 30%.
This substantial market share gives MCT a unique position in the industry, allowing it to influence trends and set standards.
Unique Types & Fields
Most data sources are limited to originations, but MCT goes deep into the secondary market with commitment, purchase, and securitizations data.
What sets MCT apart is its ability to provide data on commitment, purchase, and securitizations, which are often overlooked in traditional data sources.
Having access to this type of data can be incredibly valuable for making informed business decisions, as it provides a more comprehensive view of the market.
MCT's focus on the secondary market allows users to gain insights into the entire lending process, from commitment to securitization.
Take a look at this: Secondary Home Mortgage Rates
Scope and Detail Level
Operational Mortgage Analytics focuses on granular, process-specific data, including detailed information on loan processing times, underwriting decisions, and staff productivity metrics.
This level of detail allows for a fine-grained analysis of the mortgage process, enabling lenders to identify areas for improvement and optimize their workflows.
Operational Mortgage Analytics looks at the inner workings of the mortgage process, examining every step from application to closing.
In contrast, Traditional Mortgage Analytics takes a broader view, considering market and customer data such as borrower credit profiles, loan-to-value ratios, and historical default rates.
This aggregated view of data over time helps lenders understand long-term trends and patterns, but may not provide the same level of detail as Operational Mortgage Analytics.
By understanding the scope and level of detail required for each type of analysis, lenders can choose the right approach for their needs and make more informed decisions.
Take a look at this: Mortgage Broker Process
Zip Codes
Zip codes are a crucial part of our daily lives, helping us navigate and track packages, bills, and important documents.
In the United States, zip codes are typically 5 digits long and are used to group mail and packages by geographic area.
The first digit of a zip code represents a group of states, with the first digit "0" indicating a group of states in the Northeast.
Zip codes can also be used to identify specific neighborhoods or areas within a city.
Did you know that the United States Postal Service (USPS) uses zip codes to sort and deliver over 180 billion pieces of mail every year?
The USPS also uses zip codes to determine the cost of shipping packages, with longer distances requiring more expensive shipping rates.
Operational Efficiency
Operational mortgage analytics focuses on the efficiency of operational processes involved in mortgage lending, including loan processing, underwriting, servicing, and default management.
Its primary goal is to optimize operational performance, reduce costs, and enhance the customer experience through data-driven insights.
Analyzing data related to workflow efficiency, process bottlenecks, and staff productivity is key to achieving operational efficiency.
Operational mortgage analytics can help identify steps in the loan processing chain that consistently experience delays, allowing for targeted improvements.
By pinpointing inefficiencies or errors, you can create a roadmap for process optimization and automation.
Automating repetitive tasks and events can significantly reduce processing times and improve accuracy.
Analyzing operational data can also help create reports that give a quick overview of where each loan stands in the pipeline and if any action is required.
Implementing change into processes and using analytics to assess effectiveness is crucial for continuous improvement.
Monthly performance reporting is essential to ensure ongoing success based on factors important to your business, such as loan sale best execution performance and investor report cards.
A detailed assessment of business workflows compared to peers and reviewing mortgage technology platforms can help mortgage lenders achieve maximum return on investment.
Related reading: Assumable Mortgages Can Help Buyers Get Sub-4 Mortgage Rates
Use Cases and Applications
Mortgage analytics is a powerful tool for making informed decisions in the mortgage industry. It helps professionals assess risk, identify opportunities, and better understand market trends.
Risk management is a critical aspect of mortgage analytics. By analyzing interest rate fluctuations, prepayment risks, and credit risks, lenders can make more informed decisions and mitigate potential losses.
Identifying prepayment risks is especially important, as it can help lenders avoid costly surprises down the line. By understanding the likelihood of prepayments, lenders can adjust their strategies and protect their investments.
Mortgage analytics also helps lenders determine the market value of mortgage-backed securities (MBS) and inform their investment decisions. Accurate pricing information is crucial for making informed decisions, and mortgage analytics provides the insights needed to make smart choices.
Advanced analytics can also be used to develop predictive models based on historical mortgage market data. These models can help lenders forecast future market trends and guide their decision-making.
Operational mortgage analytics focuses on improving processes like loan origination, underwriting, servicing, and default management. By analyzing and optimizing these processes, lenders can reduce costs and improve efficiency.
Traditional mortgage analytics, on the other hand, focuses on broader aspects like market trends, borrower behavior, loan performance, risk assessment, and portfolio analysis. This approach helps lenders understand market dynamics, assess credit risk, and make strategic decisions based on long-term trends and borrower demographics.
For another approach, see: Rocket Mortgage Cash Out Refinance Prepayment
Here are some specific use cases for advanced analytics in mortgage originations:
- Assessing and managing interest rate fluctuations
- Identifying prepayment risks and credit risks
- Determining market value of MBS
- Informing investment decisions based on insight to accurate pricing information
- Developing predictive models based on historical mortgage market data
- Forecasting future market trends and guiding decision-making
By leveraging mortgage analytics, lenders can gain a competitive edge, protect their investments, and make more informed decisions.
Technical Details
Mortgage analytics involves the use of data and statistical models to analyze and predict mortgage performance.
To begin, let's talk about the types of data used in mortgage analytics. This includes credit data, property data, and loan data. Credit data includes information about the borrower's credit history, income, and employment status. Property data includes information about the property's value, location, and condition. Loan data includes information about the loan terms, interest rate, and repayment schedule.
Mortgage analytics often uses machine learning algorithms to analyze this data and identify patterns and trends. These algorithms can be trained on large datasets to make accurate predictions about mortgage performance. For example, a model might be trained to predict the likelihood of a borrower defaulting on a loan based on their credit history and income.
Take a look at this: History of Mortgage Banking
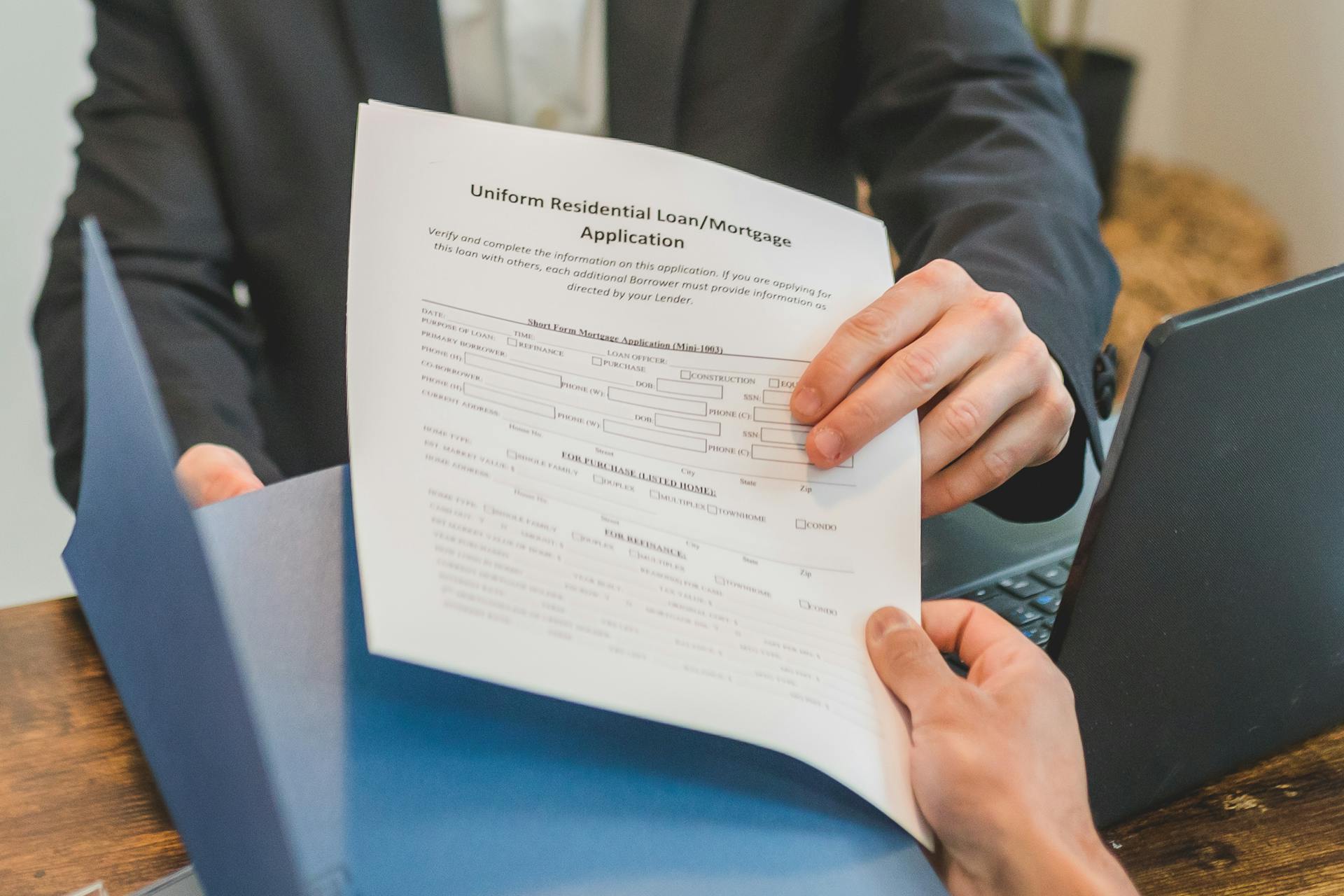
In addition to machine learning algorithms, mortgage analytics also uses traditional statistical methods such as regression analysis to analyze the data. Regression analysis can be used to identify the relationships between different variables and make predictions about mortgage performance. For instance, a regression analysis might show that a borrower's credit score is strongly correlated with their likelihood of defaulting on a loan.
The frequency and depth of data collection also play a crucial role in mortgage analytics. In some cases, data is collected daily, while in others, it may be collected monthly or quarterly. The depth of data collection can also vary, with some lenders collecting detailed information about a borrower's credit history, while others may only collect basic information.
Mortgage analytics can be used to identify high-risk borrowers and prevent defaults. By analyzing large datasets, lenders can identify patterns and trends that indicate a borrower is likely to default on a loan. This information can be used to adjust lending policies and prevent defaults. For example, a lender might raise the interest rate on a loan for a borrower with a poor credit history to reduce the risk of default.
Business Goals and Objectives
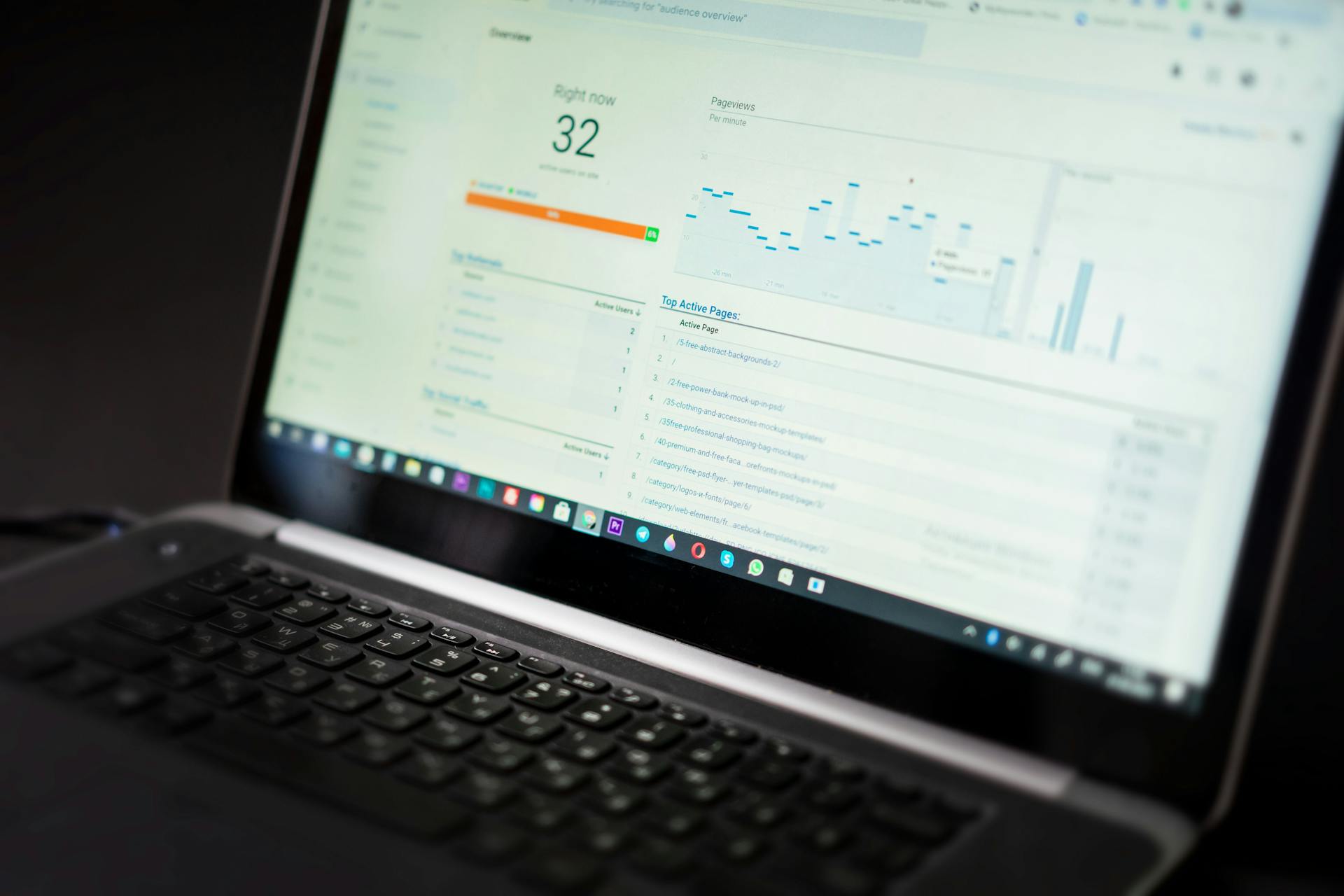
Mortgage analytics can help streamline operations, reduce costs, and improve customer experience.
Operational Mortgage Analytics is primarily used to make tactical improvements in mortgage operations.
It can also enhance compliance within daily operational processes.
Traditional Mortgage Analytics focuses on strategic decision-making and risk management.
This type of analytics is more about guiding long-term business strategies.
It helps mortgage companies understand broader market risks and opportunities.
By using technology, mortgage companies can reach their strategic goals.
A software consultant with a background in software engineering can help build custom tools and automation solutions for mortgage lenders.
A unique perspective: Mortgage Servicing Companies in Texas
Competitive Analysis
Competitive Analysis is a crucial aspect of mortgage analytics. It helps you understand how your mortgage performance stacks up against your peers.
To conduct a competitive analysis, you can use detailed performance reporting. This includes metrics such as landing page dashboard, locked loan reporting, and sold loan reporting.
These metrics provide a clear picture of your mortgage business. For instance, the landing page dashboard shows how your website is performing in terms of mortgage inquiries.
Locked loan reporting and sold loan reporting are equally important. They give you insights into your loan origination and sales processes.
Capital markets reporting is another essential aspect of competitive analysis. It helps you understand the market trends and how they impact your mortgage business.
Finally, peer group benchmark reporting is a must-have. It allows you to compare your mortgage performance with that of your peers, helping you identify areas for improvement.
Here are some key metrics to track in your competitive analysis:
- Landing Page Dashboard
- Locked Loan Reporting
- Sold Loan Reporting
- Capital Markets Reporting
- Peer Group Benchmark Reporting
Lender and Servicing
Monitoring liquidity in the servicing market is a mission-critical task for mortgage capital markets professionals and prepayment researchers. Knowing who is buying and selling servicing requires persistent oversight of lender and servicer naming taxonomies across the agencies.
Buyers of Non-Banking Servicing by Transfer Type, Program, and State are a crucial aspect of this oversight. This information helps lenders and servicers stay on top of market trends and make informed decisions.
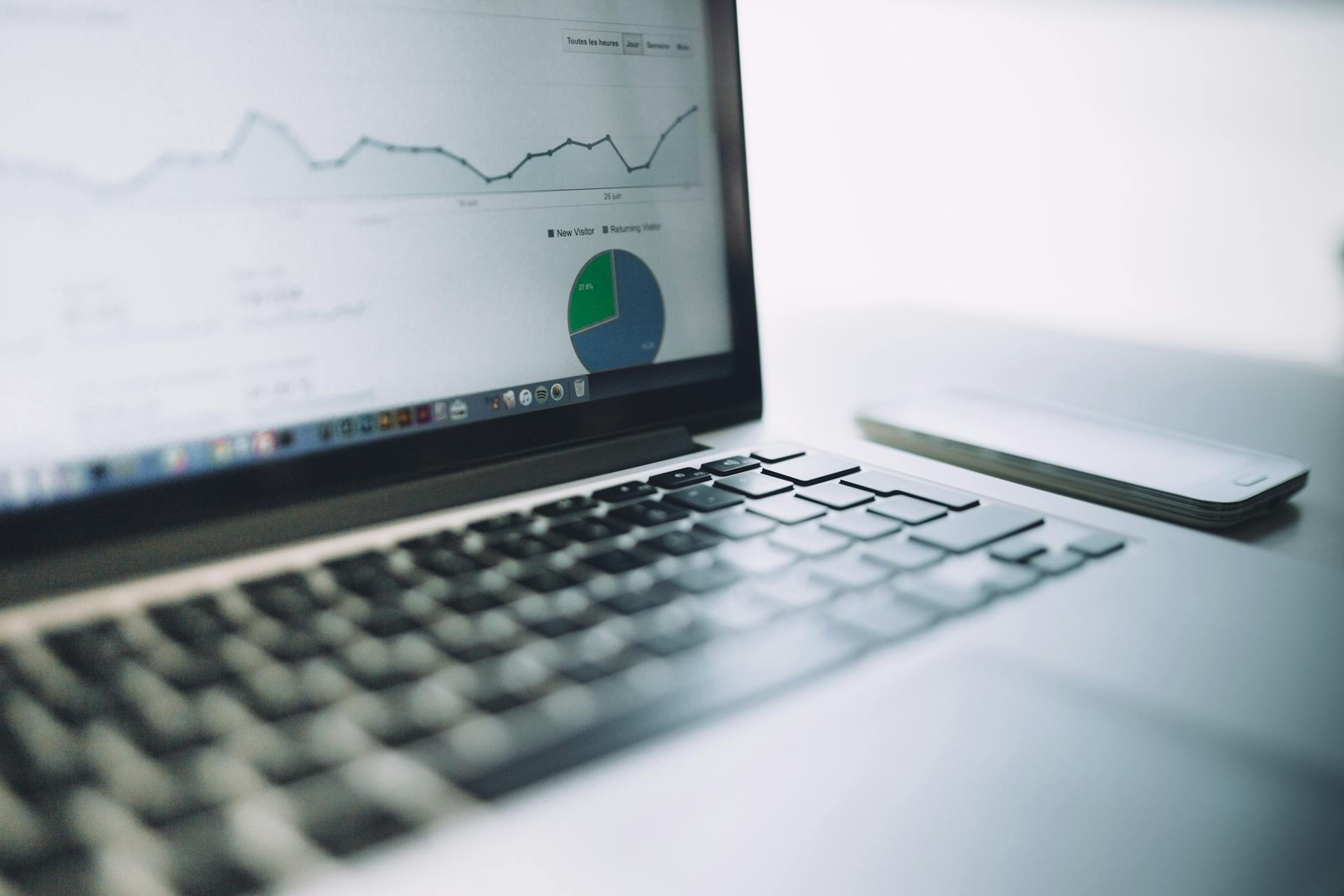
Lender Analytics is essential for determining hedgeable product and managing risk. By analyzing data, lenders can be prepared to react quickly to changes in the market.
To evaluate mortgage pipeline performance, lenders should complete a detailed assessment of business workflows compared to their peers. This includes reviewing mortgage technology platforms to achieve maximum return on investment.
Monthly performance reporting should be implemented to ensure continuing success based on factors important to the business. This could include a review of loan sale best execution performance and investor report cards.
Frequently Asked Questions
What is loan analytics?
LoanAnalytics provides customizable solutions to help lending institutions manage loan portfolios and reduce risk, while increasing returns. Our Quantitative Loan Portfolio Management Process is at the heart of our mission to empower lenders with data-driven insights.
Is mortgage data public?
Yes, mortgage data is publicly available, with the HMDA data and reports providing comprehensive information on mortgage market activity.
Sources
- https://dna.firstam.com/mortgage-analytics-data
- https://www.lseg.com/en/data-analytics/products/yield-book/advanced-mortgage-analytics
- https://mct-trading.com/mortgage-data-analytics/
- https://www.infosysbpm.com/blogs/bpm-analytics/deploying-advanced-analytics-in-mortgage-originations.html
- https://www.opsflowhq.com/newsletter-issues/whats-operational-mortgage-analytics
Featured Images: pexels.com