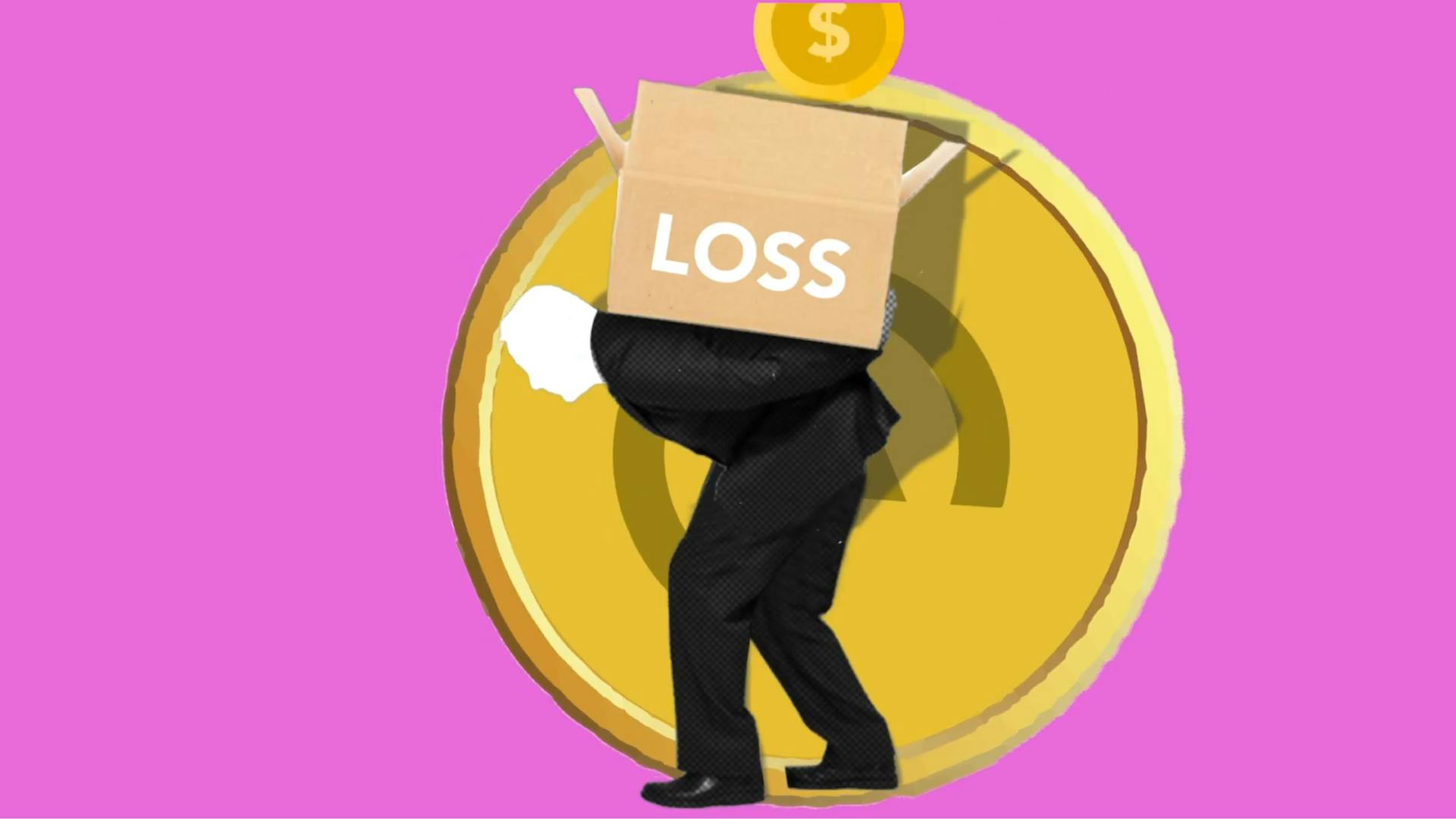
The loss development factor is a crucial concept in insurance that helps companies accurately estimate future claims costs. It's a percentage that reflects how much the cost of a claim will increase over time.
A loss development factor can range from 0 to 100%, with higher percentages indicating a greater increase in claims costs. For instance, a loss development factor of 50% means that the cost of a claim will increase by 50% over time.
As claims age, they tend to increase in cost, which is why loss development factors are typically higher for older claims. This is because the cost of settling a claim can escalate over time due to various factors, such as medical inflation or changes in the law.
Loss development factors can be influenced by a variety of factors, including the type of claim, the severity of the injury, and the jurisdiction in which the claim is being settled.
What Is
Loss development is the difference between the final losses recorded by an insurer and what the insurer originally recorded.
Loss development seeks to account for the fact that some insurance claims take a long time to settle, and that estimates of the total loss an insurer will experience will adjust as claims are finalized.
Loss development factors are used by actuaries, underwriters, and other insurance professionals to "develop" claim amounts to their estimated final value.
A loss development factor (LDF) is a number that is meant to adjust claims to their ultimate projected level, such as an LDF of 2.0, which means that for every $1 in claims, the ultimate payout will be $2.
Loss amounts are key for pricing insurance premiums and determining carried reserves, which requires knowing the ultimate loss amounts of an insurance company's claims.
Calculating LDF
Calculating LDF can be a straightforward process, as it's essentially the ratio of the ultimate loss to the initial loss.
The ultimate loss is the total amount of loss that will be incurred over the lifetime of a policy, which can be estimated using the average annual loss (AAL) and the expected loss ratio (ELR).
A loss development factor (LDF) of 1 indicates that the ultimate loss is equal to the initial loss, while an LDF greater than 1 suggests that the ultimate loss will be higher than the initial loss.
The expected loss ratio (ELR) is a critical component of the LDF calculation, as it represents the proportion of initial losses that are expected to be incurred in the future.
By using historical data and actuarial models, insurers can estimate the ELR and make informed decisions about policy pricing and risk management.
LDF Methods and Techniques
The Loss Development Factor (LDF) is a crucial concept in insurance that helps predict future losses. It's calculated as a ratio of the average loss payment to the average exposure unit.
There are several methods to estimate LDF, including the Chain Ladder method, which is a simple and widely used technique. This method requires a minimum of three years of data to produce reliable results.
The LDF can be influenced by various factors, such as inflation and changes in exposure, which can impact the accuracy of the predictions.
Clark LDF in Chain Ladder
Clark LDF is a type of Loss Development Factor method used in the Chain Ladder technique. It's based on the idea of grouping claims by their development age.
The Clark LDF method is often used in conjunction with the Chain Ladder technique to estimate future losses. This is because it provides a more accurate picture of loss development over time.
Clark LDF is particularly useful for claims that develop over a long period. For example, a claim that takes 5 years to develop might be grouped with other claims that also take 5 years to develop.
The Clark LDF method can be complex to implement, especially for large datasets. However, it's a powerful tool for actuaries and risk managers who need to estimate future losses accurately.
Smoothing Age-to-Age
Smoothing Age-to-Age loss development factors can be a challenge, but actuaries have a technique to help smooth out lumpy patterns. This technique uses the relationship between adjacent age-to-age factors (ATAs) from a benchmark loss development pattern.
The benchmark pattern is used to calculate the ATA factor for a specific age range. For example, the ATA factor for ages 36 to 72 can be calculated as the product of the respective ATAs for ages 36 to 48, 48 to 60, and 60 to 72. This equals 1.172.
To smooth out the lumpy pattern, you calculate the logarithmic relativities corresponding to each period. These are calculated as the ratio of the natural log of the ATA period to the natural log of the entire 36-month period.
The relativities are then applied as exponents to the initial selected ATA factor for the corresponding period. This results in a smoothed ATA factor that mirrors the shape of the benchmark pattern.
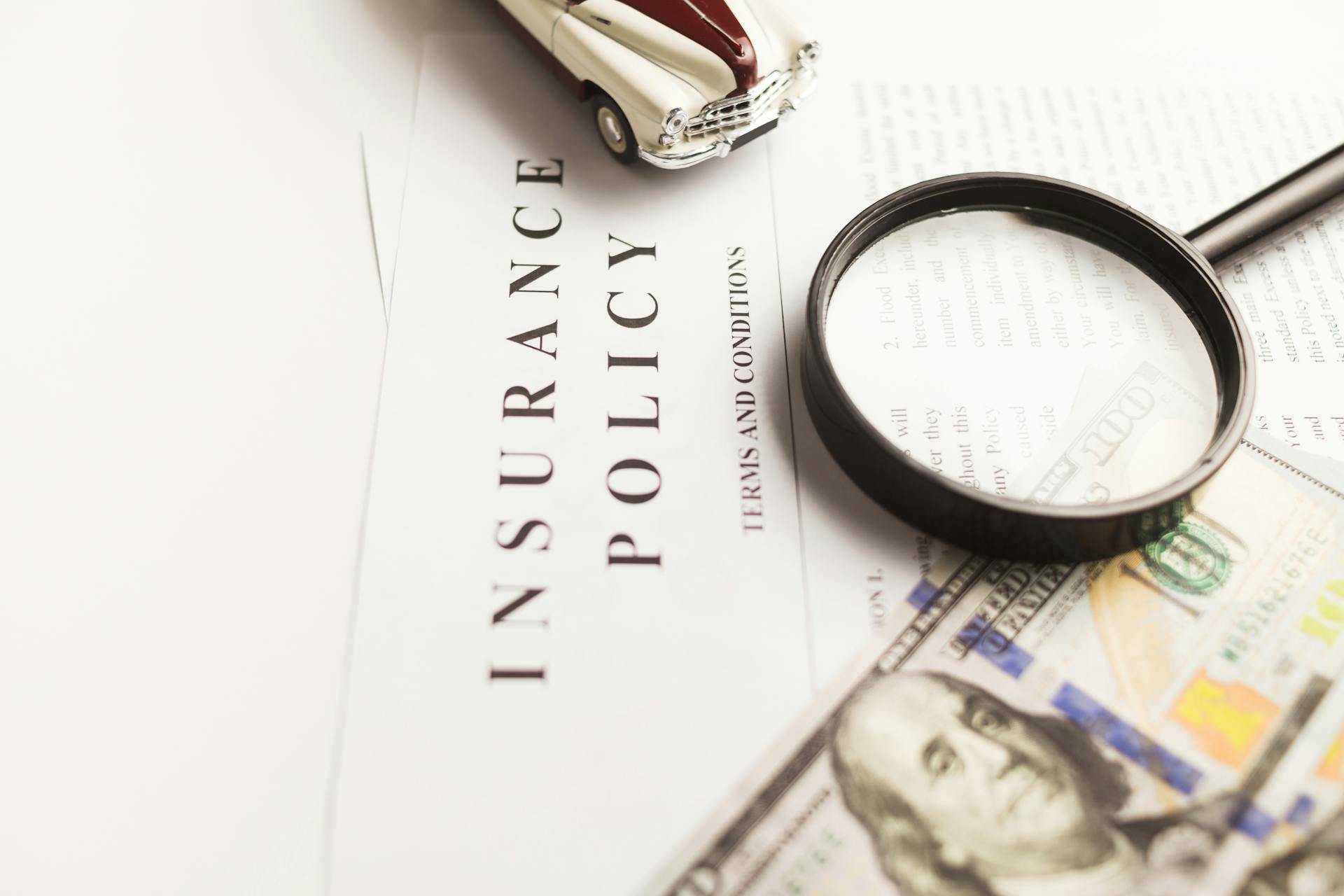
Using this technique, the unsmoothed/smoothed age-to-ultimate factors for earlier ages are equal. For example, in the case of ages 36 and prior, the unsmoothed/smoothed age-to-ultimate factors are equal.
The output of a loss development triangle, such as Figure 2, is loss development factors (LDFs), which include age-to-age factors (ATAFs). These are determined by dividing losses at a later maturity by an earlier maturity.
For instance, dividing the 2020 losses that are 24 months old by the 2020 losses that are 12 months old produces a 12-24-month ATAF for the 2020 year of 3.081.
Double Weighted Averages
Double Weighted Averages are a superior method to loss weighted averages because they help prevent unusual data points from skewing averages.
This method involves using two weights: one for loss volume and another for time, with the most recent data typically receiving the most weight.
The time-based weights can be determined using a simple scheme, where the value of the weight varies based on the recency of the loss data being evaluated.
Losses underlying the 1st prior development evaluation receive a relative weight of α, which can be a value between 0 and 1.00.
The smaller the value of α, the more weight is applied to the loss development for recent periods.
In practice, actuaries commonly use α values between 0.75 and 0.95, and may calculate a series of weighted averages to recognize the fact that older periods may be less representative of current development patterns.
The Double Weighted Average is a great way to contemplate ALL historical data while still reducing the influence of the older periods.
This method can be applied uniformly across all periods when the selected starting weight equals 1.00 (α = 1.00), reducing to a loss weighted average.
LDF Uncertainty Mitigation Techniques
One of the most effective techniques to mitigate uncertainty in LDF is to use a robust design approach, which can be achieved by considering multiple design scenarios and selecting the most optimal one.
A robust design approach can help reduce the impact of uncertainty on LDF by ensuring that the design is not overly sensitive to changes in the design variables.
Another technique is to use a Monte Carlo simulation, which can help quantify the uncertainty in the design variables and provide a range of possible outcomes.
Monte Carlo simulations can be used to analyze the behavior of the LDF under different uncertainty scenarios, allowing designers to make more informed decisions.
By using a combination of robust design and Monte Carlo simulation, designers can develop an LDF that is more resilient to uncertainty and better able to adapt to changing conditions.
LDF Analysis and Tools
Loss development factors (LDFs) are determined by the output of a triangle, as shown in Figure 2. This output is used to analyze and understand the development of losses over time.
Age-to-age factors (ATAFs), or incremental LDFs, are calculated by dividing losses at a later maturity by an earlier maturity. For example, dividing $2,200 by $714 will produce a 12-24-month ATAF for the 2020 year of 3.081.
Understanding how to calculate and analyze LDFs and ATAFs is crucial for accurately forecasting future losses and making informed business decisions.
Technology in LDF Analysis
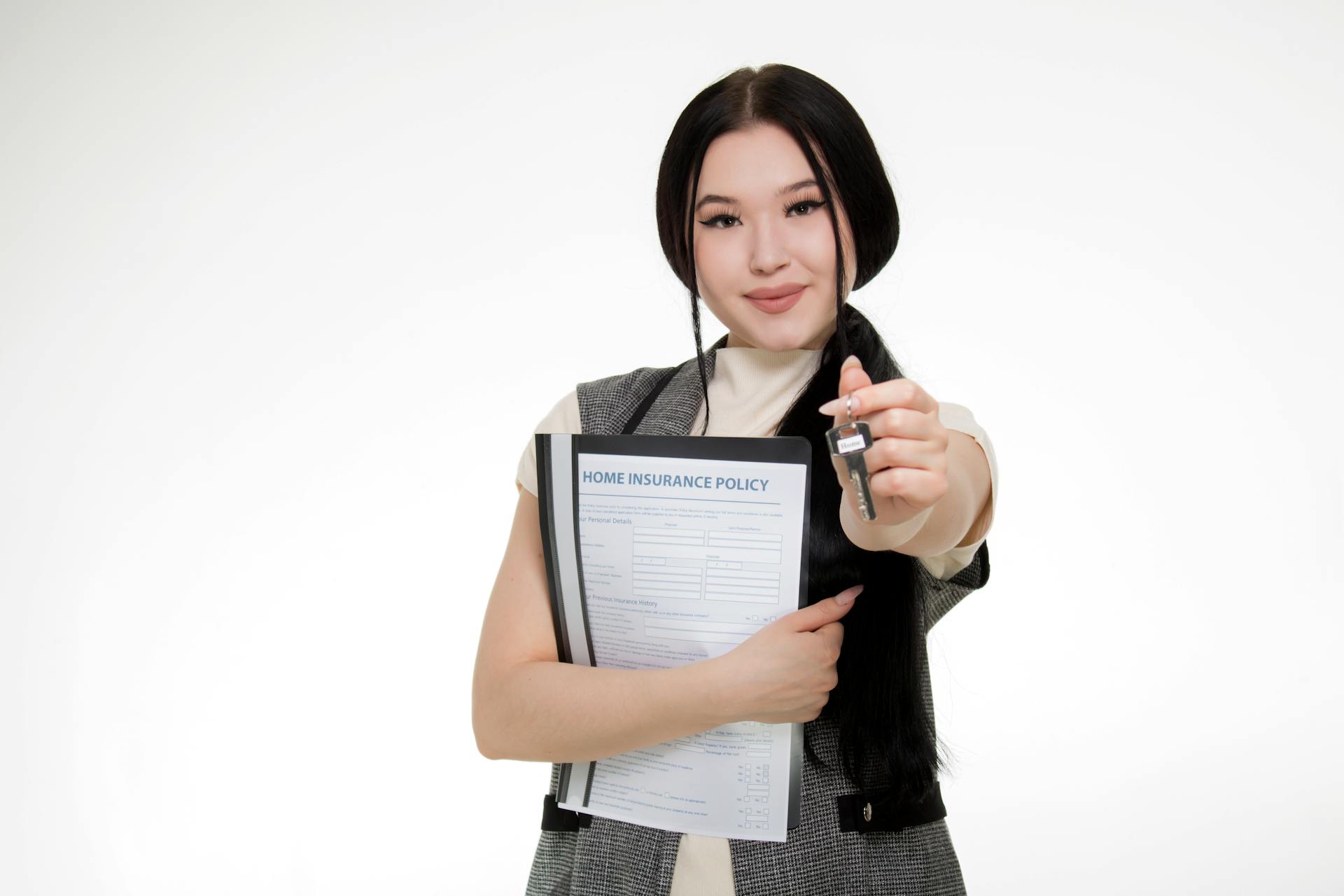
Technology has greatly impacted the field of LDF analysis, making it more efficient and accurate.
Specialized software programs, such as those mentioned in the "Software Tools for LDF Analysis" section, have automated many tasks, reducing the time and effort required for analysis.
These programs can handle large datasets and complex calculations, providing instant results and freeing up analysts to focus on higher-level tasks.
Some popular software options include LDF Expert and LDF Pro, which are discussed in the "Software Options for LDF Analysis" section.
These tools offer advanced features, such as predictive modeling and data visualization, that can help analysts identify patterns and trends in their data.
The use of cloud computing has also become increasingly prevalent in LDF analysis, as it allows for seamless collaboration and data sharing among team members.
Cloud-based platforms, like those mentioned in the "Cloud-Based LDF Analysis Solutions" section, provide secure and scalable storage for large datasets, making it easier to work with complex data.
Selections and Cumulative Paid
The selection of LDFs is largely subjective, but actuaries look for trends and shifts in patterns to make their selections.
Actuaries will often look at a variety of averages, as shown in Figure 3, to select each ATAF.
The selection of the tail factor may be based on an industry benchmark, a curve-fitting exercise, or actuarial judgment, depending on the line of business.
The last maturity of the last ATAF, the number of open claims, or the amount of case reserves are also considered when selecting the tail factor.
The tail factor in Figure 3 is 1.065, which is a key component in determining cumulative LDFs.
Each of the selected ATAFs are multiplied together to determine cumulative LDFs.
For example, the tail factor of 1.065 multiplied by the 108-120-month ATAF of 1.006 equals 1.072, which is called the 108-ultimate factor.
This 108-ultimate factor would be applied to losses that are 108 months from inception.
It's essential to match the characteristics of those losses when applying LDFs, or it may overstate or understate the future development on those losses.
For instance, it's not appropriate to apply unlimited LDFs to losses limited to $250,000 per claim.
Challenges and Uncertainty
As we explore the concept of loss development factor, it's essential to acknowledge the challenges and uncertainty that come with it. The lack of reliable data on loss development patterns is a significant issue, making it difficult to accurately estimate future losses.
The limited availability of data on loss development patterns is a major concern, particularly for insurers who need to make informed decisions about pricing and reserving. This lack of data can lead to significant errors in loss development factor calculations.
The uncertainty surrounding loss development factor is further complicated by the presence of outliers, which can skew the data and lead to inaccurate predictions.
Triple Whammy Effect
The Triple Whammy Effect is a phenomenon that can significantly impact P&C insurance reserve estimates. It occurs when reported loss emergence deviates from expectations, leading to a chain reaction of effects that can result in increased ultimate loss estimates.
Higher-than-expected reported loss emergence can trigger the triple-whammy effect, which consists of three distinct effects. The first effect is a direct increase in ultimate loss estimates, equal to the higher-than-expected reported loss emergence.
For instance, if reported losses emerge higher than expected by $1M, the estimate of ultimate loss will increase by $1M. This is whammy #1. Additional IBNR is also necessary to cover development on the higher-than-expected reported loss emergence, which can add up to $500K.
The magnitude of this effect is determined by the ATU factor, which is used to calculate IBNR. In the example, the ATU factor is 1.5, resulting in a $500K provision for development.
The second effect of the triple-whammy is the re-evaluation of the development pattern, which can lead to a revision of the ATU factor. In the example, the ATU factor is increased from 1.5 to 1.6, resulting in an additional $600K of IBNR.
This additional IBNR is necessary to cover development as a result of revised assumptions about future loss development and its effects on the entire portfolio. This is whammy #3, and it can have a significant impact on reserve estimates.
In practice, actuaries do not rely exclusively on the reported loss development method, so the triple-whammy effect tends to be mitigated. However, this example accurately reflects the upward pressure created on reserve estimates when higher-than-expected reported loss emergence is observed.
Challenges in Estimating
Estimating the future is a tricky business, and it's not just because of the unpredictability of events. Complex systems and interactions can make it difficult to accurately forecast outcomes.
One of the main challenges is accounting for the butterfly effect, where small changes can have significant impacts. This was seen in the example of the drought in California, where a slight variation in the El Niño event led to devastating consequences.
The uncertainty of human behavior is another challenge. As seen in the case of the financial crisis, people's actions can be influenced by emotions and biases, making it hard to predict their decisions.
The complexity of systems is a major obstacle to accurate estimation. The example of the global supply chain showed how a single disruption can have far-reaching effects, making it difficult to anticipate and prepare for potential issues.
In addition, the availability of data can be limited, making it harder to make informed decisions. The article on the limitations of data collection highlighted the challenges of gathering accurate and relevant information.
Estimating the future is a daunting task, but by acknowledging these challenges, we can begin to develop strategies to mitigate their impact.
Data and Exposure
Data is crucial in calculating loss development factors, and one important aspect of data is exposure. Exposure is used to gauge how large a company is and its propensity to loss.
Exposure measures vary by coverage, so it's essential to use the right one. For example, payroll, head count, or employee hours are often used for workers' compensation, while revenue is used for general liability.
The choice of exposure measure can significantly impact the loss development factor calculation. For instance, if an actuary is reviewing auto liability exposure and notes that losses have doubled, it may seem like loss experience is drastically increasing. However, if the actuary is aware that the number of vehicles covered by the policy has also doubled, the increase in losses is anticipated.
Here's a brief overview of common exposure measures for different coverages:
Exposures
Exposures are a crucial piece of information that actuaries use to gauge how large a company is and its propensity to loss. This can vary by coverage, with different exposure measures being used for different types of insurance.
For example, in workers' compensation, the exposure is typically measured by payroll, head count, or employee hours. This is because these factors can impact the likelihood and severity of workplace injuries.
In general liability, revenue is often used as the exposure measure. And for auto liability, auto count or mileage is commonly used.
Here are some examples of exposure measures by coverage:
Understanding exposures is important because it can impact how actuaries interpret loss data. For example, if losses have doubled in a given year, it may seem like loss experience is increasing. But if the exposure has also doubled, that increase in losses is anticipated.
Claims Disposal Ratio
The claim disposal ratio is an important metric for any P&C insurer, measuring the number of claims closed in a calendar period relative to the total inventory of claims available to be closed.
This metric is calculated by adding the open inventory of claims at the beginning of the period to the number of claims reported during the same period.
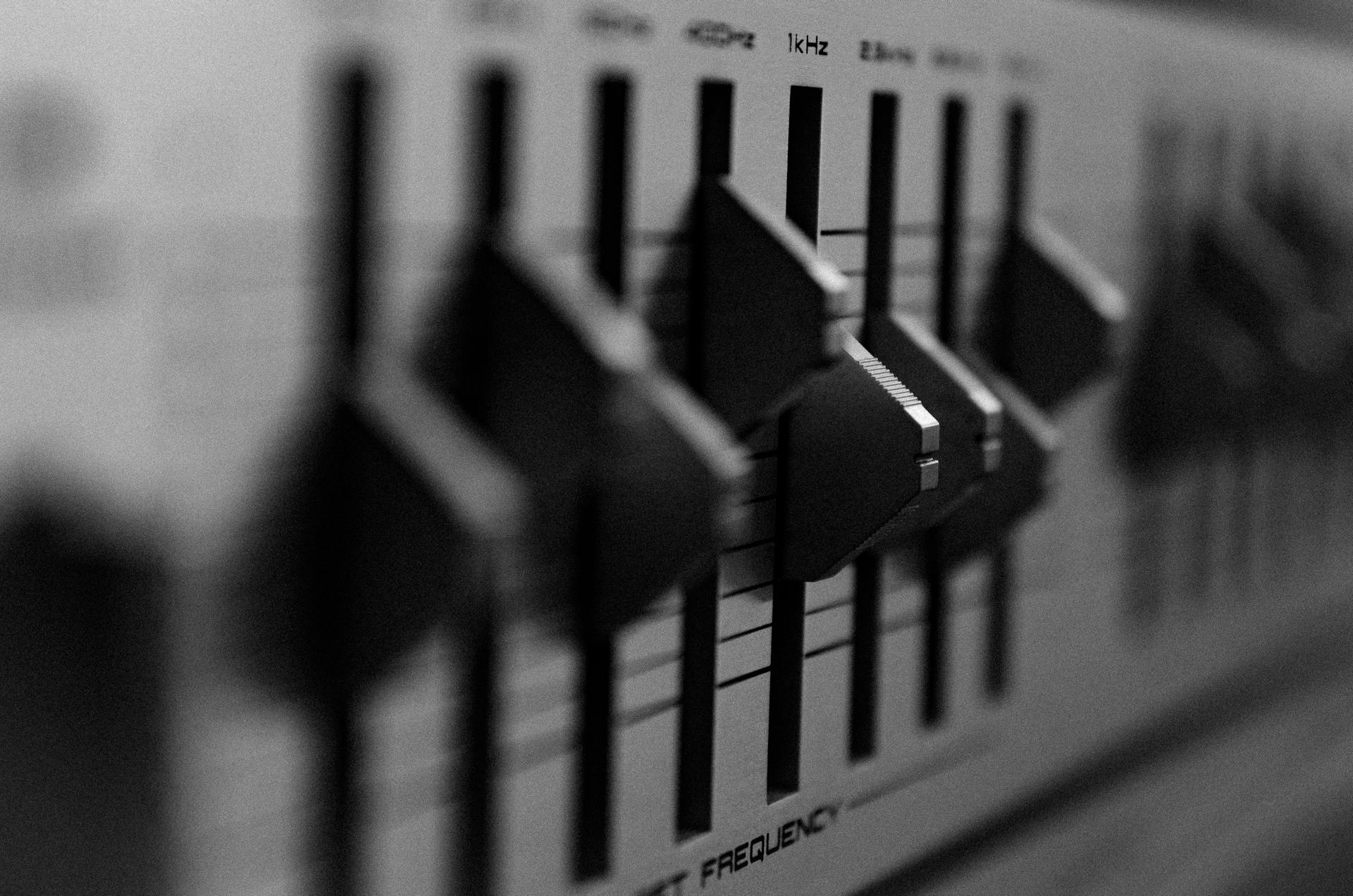
The total inventory of claims available to be closed is a key component of this metric, and it's essential to accurately track and update this number regularly.
A high claim disposal ratio indicates that an insurer is efficiently closing claims, while a low ratio may suggest that there are issues with the claims process or a large backlog of claims.
Monitoring and interpreting claim closing rates are essential to the success of any P&C insurer, and the claim disposal ratio is a valuable tool for achieving this goal.
Calendar Dates
Calendar dates play a crucial role in organizing data related to loss runs. The date of loss, also known as the accident date or incident date, marks the date the accident occurred.
For instance, a workers' compensation claim that occurred in 2019 but wasn't reported until 2022 would have a report lag of three years. Report lag refers to the length of time between the date of loss and the date of report.
Auto liability claims are typically reported very quickly, but hospital professional liability claims may not be known about or reported for many years after they occurred. This distinction is important, particularly for certain lines of business.
Frequently Asked Questions
What is the age-to-age loss development factor?
The age-to-age loss development factor (LDF) is a ratio that shows how loss amounts grow over time. It helps predict where ultimate losses will settle by projecting future loss growth patterns.
What is adverse loss development?
An Adverse Development Cover (ADC) provides protection for future losses from past claims, paying out excess losses above a retention level and up to a specified limit. This type of reinsurance helps manage the financial impact of unexpected claim developments.
Sources
- https://archeractuarial.com/tools/
- https://fastercapital.com/content/Loss-development-factor--Unraveling-the-Dynamics-of-Claims-Reserve-Changes-update.html
- https://rdrr.io/cran/ChainLadder/man/ClarkLDF.html
- https://www.milliman.com/en/insight/a-beginners-guide-to-the-casualty-actuarial-language
- https://www.investopedia.com/terms/l/loss-development.asp
Featured Images: pexels.com