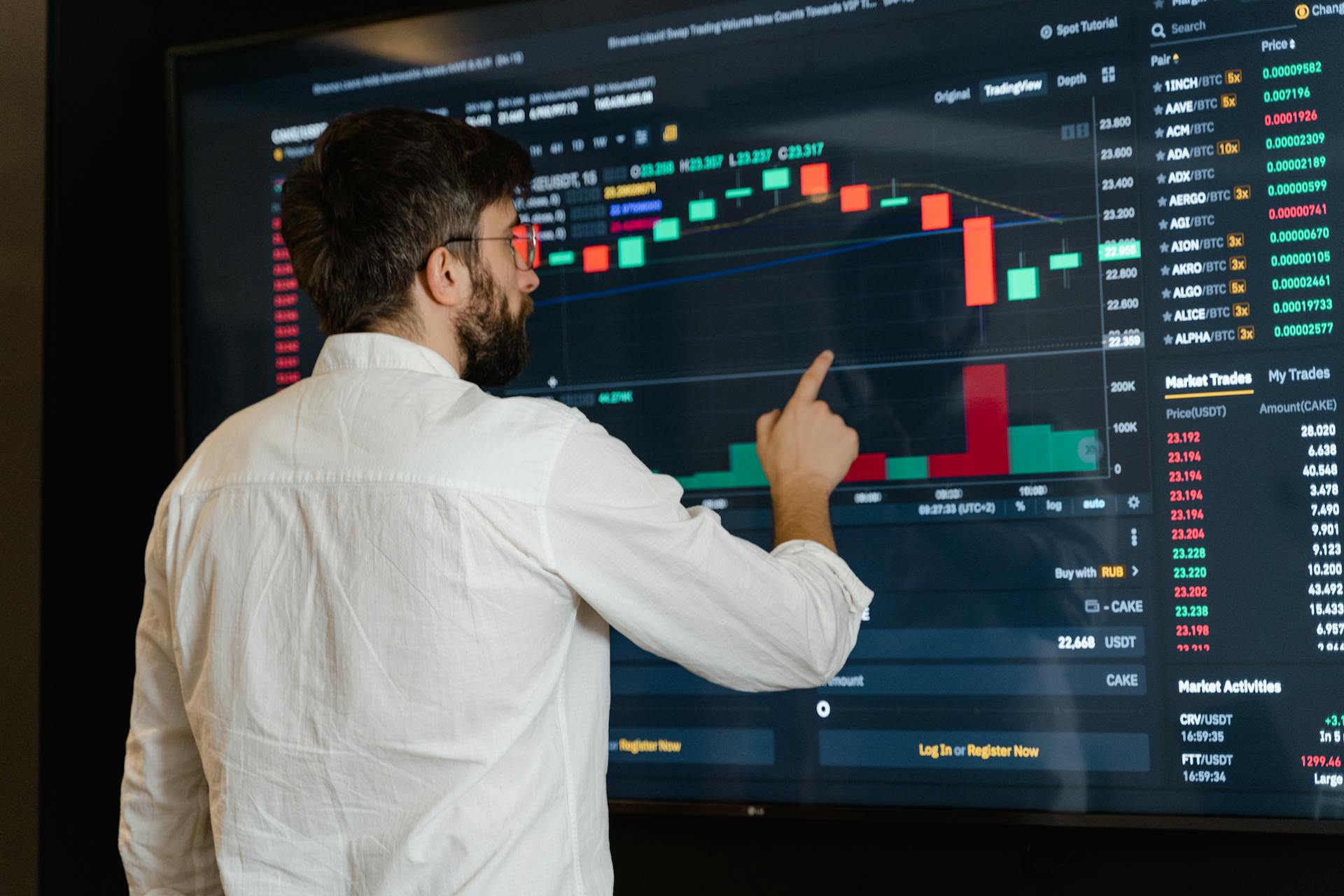
Insurance data analysis is revolutionizing the insurtech landscape by unlocking new insights and opportunities. With the help of advanced analytics, insurers can now make more informed decisions and create personalized policies that better meet their customers' needs.
By leveraging data from various sources, such as claims history and customer behavior, insurers can identify high-risk customers and offer them tailored policies that reduce their premiums. This approach not only benefits the customer but also helps insurers manage their risk exposure more effectively.
According to a recent study, using data analytics can reduce claims costs by up to 20%. This is a significant reduction that can have a major impact on an insurer's bottom line.
Insurers are also using data analysis to create more accurate risk assessments, which enables them to price policies more competitively and attract more customers.
For more insights, see: How Do Insurance Policies Work
Insurance Data Analysis
Insurance data analysis is a crucial step in understanding your client base and claims data. It helps uncover data patterns and trends, such as an insurance firm summarizing its client base and claims data.
There are four categories of analyses: descriptive, diagnostic, predictive, and prescriptive analytics. Descriptive analysis explains what happened in the past, while diagnostic analysis explains why something happened. Predictive analysis uses historical data to forecast future patterns, and prescriptive analytics makes suggestions for the future.
Here are the four categories of analyses:
- Descriptive analysis: Explains what happened in the past.
- Diagnostic analysis: Explains why something happened.
- Predictive analysis: Uses historical data to forecast future patterns.
- Prescriptive analytics: Makes suggestions for the future.
Insurance firms can use these analyses to create new insurance products, such as per-per-mile models, and price policies more effectively.
Insurtech Revolution
The Insurtech Revolution is upon us, and it's changing the face of the insurance industry forever. According to Grand View Research, the worldwide insurance analytics market is predicted to grow at an 11.7% CAGR from 2016 to 2027.
Insurtech companies are utilizing advanced analytics and innovative business operations to challenge traditional insurance models. They're using data analytics to price policies and create new insurance products that traditional insurers never even considered.
Rising market competitiveness is driving insurance businesses to modernize their corporation models, simplify operations, and optimize procedures. This is leading to the adoption of new technologies and business strategies.
Insurtech companies are leveraging data analytics to process claims instantly, disrupting traditional insurance pricing models with per-per-mile and other innovative pricing models.
If this caught your attention, see: Axa Group Operations
Analysis
Analysis is a crucial step in insurance data analysis. It's where you take the data you've collected and make sense of it.
Descriptive analysis is a type of analysis that explains what happened in the past. For example, an insurance firm can summarize its client base and claims data to uncover patterns and trends.
Descriptive analysis can help identify areas of improvement in the business. For instance, if an insurance company finds that a certain type of claim is more frequent than others, they can adjust their pricing or marketing strategies accordingly.
Predictive analysis uses historical data to forecast future patterns. Insurance companies can use this type of analysis to create predictive models that forecast client behavior, market trends, or risk factors.
Predictive maintenance for insured assets is a great example of predictive analysis in action. By analyzing data trends, insurers can identify potential issues before they become major problems.
Broaden your view: Which Type of Life Insurance Policy Generates Immediate Cash Value
Here are the four categories of analyses:
- Descriptive analysis: explains what happened in the past
- Diagnostic analysis: explains why something happened
- Predictive analysis: uses historical data to forecast future patterns
- Prescriptive analytics: makes suggestions for the future
Prescriptive analytics can be used by insurance firms to assist consumers in managing risks or to offer fraud-prevention measures. This type of analysis can help insurers provide better services to their customers.
Data visualization is also an important part of the analysis process. Insurers need to communicate their findings to a large number of stakeholders, so clear visualizations and explicit explanations are essential.
Benefits and Advantages
Insurance data analysis offers numerous benefits for insurance companies. By leveraging data analytics, organizations can make fast, informed decisions from anywhere, speeding up decision-making overall.
Data visualization brings insights to life, collating information components across accident statistics and policyholders' personal information. This helps identify different risk categories, prevent fraud losses, and optimize expenses.
Smart analytics solutions enable organizations to make decisions across various areas of their business. The key insurance data analytics benefits include improved financial stability and growth, an enhanced customer experience, and more effective long-term planning.
You might like: Employee Benefits Insurance Broker
Data-driven decision-making empowers the insurance industry by providing customized customer experiences, optimizing risk assessment, and boosting operational efficiency. Advanced algorithms can analyze vast amounts of structured and unstructured data to predict claim probabilities with better accuracy.
Here are some ways small insurance companies can utilize data analytics effectively:
- Risk Assessment: Use predictive analytics for accurate underwriting and pricing
- Fraud Detection: Implement ML algorithms to identify suspicious claims patterns
- Claims Processing: Automate routine claims for faster settlement
- Customer Insights: Analyze behavior data for targeted product offerings
- Operations: Optimize workflows and resource allocation through process analytics
- Market Analysis: Track trends and competitor data for strategic decisions
Data analytics can also enhance operational efficiency by streamlining processes and automating routine tasks. This allows brokers and underwriters to make fast, informed decisions from their preferred location or device.
Data Analysis Process
Data analysis is a crucial step in insurance data analysis. It helps insurance companies make informed decisions by extracting valuable insights from their data.
There are four types of analyses: descriptive, diagnostic, predictive, and prescriptive. Descriptive analysis tells you what has happened in the past, while diagnostic analysis helps you understand why something occurred.
Predictive analysis predicts future trends based on past data, and prescriptive analytics provides recommendations for the future. For example, an insurance company might use predictive analysis to develop and train models that predict customer behaviors, market trends, and risk factors.
Here are the four types of analyses in a concise table:
Collection
Collecting the right data is crucial for accurate results. Insurance companies have a wealth of data, such as customer details and past claims.
To get started, insurance firms need to identify specific difficulties or concerns they want to answer with data insights. These issues might include optimizing premium pricing, recognizing fraud, forecasting client turnover, and simplifying claims processing.
A clearly defined issue serves as the cornerstone for the whole analytics process, directing future steps. The data collected must be relevant and comprise both first and second-party data.
Insurance firms have a variety of data, such as client information and previous claims. However, in order to obtain good findings, the data must be relevant and comprise both first and second-party data.
Model
Modeling is a crucial step in the data analysis process. It involves using data to develop statistical models that can predict future trends and behaviors.
There are four types of analyses: descriptive, diagnostic, predictive, and prescriptive. Descriptive analysis tells you what has happened in the past, while diagnostic analysis helps you understand why something occurred. Predictive analysis predicts future trends based on past data, and prescriptive analytics provides recommendations for the future.
Predictive analytics in underwriting is particularly useful for assessing risk and detecting potential claims. By analyzing various factors, such as age, employment, health condition, and credit score, insurers can create statistical models that help them make informed decisions.
Here are the four types of analyses in more detail:
By using data-driven decision-making, insurers can optimize each step in their insurance value chain and provide responsive and reliable service to customers.
Results and Sharing
After you've completed the data analysis, it's essential to share the results with all stakeholders involved. Insurance companies have many stakeholders, and to ensure everyone understands the results, explain the information thoroughly with clear visualizations and precise explanations.
Insurance companies have a lot of data to analyze, and the results can be complex. To simplify the information, use clear visualizations such as charts, graphs, and tables to help stakeholders quickly grasp the key findings.
The results must be shared with many people, including executives, customers, and other stakeholders. To ensure everyone understands the results, provide precise explanations of the data and how it was analyzed.
Predictive Maintenance
Predictive maintenance is a game-changer for property insurers. By using data analytics and IoT, insurers can analyze data and notify policyholders about necessary maintenance actions based on real-time conditions.
For example, if sensors detect wear in machinery or vehicles, insurers can alert customers to perform maintenance, thus avoiding costly repairs or accidents.
Predictive maintenance can also help insurers identify maintenance needs and mitigate risks proactively. This proactive approach can save policyholders money and reduce the risk of accidents.
Here are some key benefits of predictive maintenance:
- Identifies maintenance needs and mitigates risks proactively
- Notifies policyholders about necessary maintenance actions
- Avoids costly repairs or accidents
Predictive Maintenance for Insured Assets
Predictive Maintenance for Insured Assets helps insurers identify maintenance needs and mitigate risks proactively. By analyzing data from sensors and IoT devices, insurers can notify policyholders about necessary maintenance actions based on real-time conditions.
Insurers can use predictive data analytics to discover and target prospective markets by analyzing behavioral trends and common features. This enables them to focus their marketing efforts more effectively.
Predictive analytics specializes in segmenting items based on data trends. In claim processing, this enables insurance claims analysis to detect high-cost and difficult claims and assign them to skilled adjusters.
With predictive maintenance, insurers can alert customers to perform maintenance, thus avoiding costly repairs or accidents. For example, if sensors detect wear in machinery or vehicles.
Predictive maintenance can help insurers transform their business by providing detailed insights and mitigating risks.
Enhanced Detection
Predictive maintenance is a game-changer for property insurers, allowing them to identify maintenance needs and mitigate risks proactively.
With the help of data analytics and IoT, insurers can analyze data and notify policyholders about necessary maintenance actions based on real-time conditions.
Predictive analytics can detect wear in machinery or vehicles, alerting customers to perform maintenance, thus avoiding costly repairs or accidents.
Here are some benefits of predictive maintenance:
- Identifies maintenance needs and mitigates risks proactively
- Analyzes data and notifies policyholders about necessary maintenance actions
- Detects wear in machinery or vehicles, avoiding costly repairs or accidents
Predictive analytics also helps insurers to detect potential fraudulent claims by cross-verifying with customer history.
Fraudulent claims detection is a critical aspect of predictive maintenance, and insurers can use predictive analytics to identify suspicious claims and prevent financial malfeasance.
Continuous learning models improve over time in their ability to detect new fraudulent methods, and automated methods can save both time and resources by promptly identifying suspicious claims for further examination.
By applying data analytics for fraudulent identification and mitigation, insurers can maintain financial stability and policyholder trust.
You might like: California Insurance Claim Time Limit
Product Optimization
Data analytics in insurance helps insurers optimize their products to better meet customer needs. This is done by analyzing consumer behavior and purchasing preferences.
Insurers can now customize policies at the individual level, using pay-as-you-go and dynamic pricing models based on clients' behavioral signals, predicted risk factors, and shopping preferences.
By analyzing product performance and profit by distribution channel, geographic region, customer segment, and other factors, insurers can identify areas for improvement and optimize their products accordingly.
Here are some key benefits of product optimization in insurance:
- Improved product relevance and competitiveness
- Increased profitability through targeted product development
- Enhanced customer experience through tailored products
With data analytics, insurers can also develop new insurance products that are relevant and competitive. This is done by analyzing customer needs, market trends, and emerging risks.
By leveraging data to inform their product development process, insurers can respond quickly to market changes and create products that meet evolving customer needs.
Operational Optimization
Insurance data analysis helps insurers uncover areas ripe for improvement through meticulous analysis of internal operations, from workflow efficiency to resource allocation. This is done by identifying bottlenecks and streamlining repetitive processes.
With data analytics, insurers can reduce operational costs and improve employee productivity. Automation of manual processes like underwriting and claims reduces costs and improves employee productivity.
Real-time performance indicators make it possible to continually track and improve operational activities. This leads to increased operational efficiency, which helps both policyholders and the insurer by lowering costs and increasing the quality of service.
Insurers can optimize each step in their insurance value chain through data-driven decision-making. Data analytics also enable insurers to check out the vast amount of historical information, creating precise risk evaluation.
Here are some key benefits of operational optimization in insurance:
- 30% more efficiency
- 40% to 70% cost savings
- 60% increase in fraud detection rates
By continuously refining and optimizing internal operations, insurers can translate this into an enhanced bottom line for the company and a superior experience for customers.
Compliance to Regulation
Compliance to Regulation is a top priority in the insurance industry, and data analytics plays a crucial role in facilitating this. By integrating data analytics, insurers can identify and mitigate risks based on regulatory standards.
Automated data reporting and gathering ensure precise and accurate reporting, making regulatory audits smoother. This is because detailed data documentation and audit trails are easily accessible.
Regulatory compliance monitoring is an ongoing process, and insurers must remain vigilant to avoid penalties and maintain their reputations. Data analytics helps track and manage the extensive data required for regulatory reporting, ensuring accuracy and timeliness.
AI tools for data analytics can identify potential compliance issues before they escalate, allowing insurers to uphold high standards of regulatory adherence. This proactive approach reduces the element of surprise and enables insurers to prepare for compliance well in advance.
Here are some key benefits of using data analytics for regulatory compliance:
- Automated data reporting and gathering
- Precise and accurate reporting
- Detailed data documentation and audit trails
- Regulatory compliance monitoring
- Predictive analysis of regulatory trends and changes
Data Analysis Tools
Insurance companies are using data analysis tools to gain a better understanding of their business. They're creating dashboards to visualize key data points.
One example of a dashboard is the Claims Summary, which provides an overview of claims data. This can help insurers identify trends and patterns in their claims process.
Another dashboard is the Risk Overview, which gives insurers a bird's eye view of their risk exposure. This can help them make more informed decisions about pricing and underwriting.
Submission Insights is another type of dashboard that's being used by insurers. It provides detailed information about submissions, such as claims frequency and severity.
Here are some examples of dashboards that insurers are using:
- Claims Summary
- Risk Overview
- Submission Insights
Business Benefits
Insurance data analytics provides a multitude of significant benefits, including improved financial stability and growth.
By accurately predicting claims and understanding risk profiles, insurers can allocate reserves more effectively and prepare for future payouts.
The use of data analytics in insurance contributes to financial stability and growth, since by accurately predicting claims and understanding risk profiles, insurers can allocate reserves more effectively and prepare for future payouts.
Recommended read: Understanding Insurance Claims
Data analytics allows insurers to base their decisions on comprehensive data analysis, leading to more accurate pricing and improved risk assessment.
Insurance companies that invested in big data analytics have seen 30% more efficiency, 40% to 70% cost savings, and a 60% increase in fraud detection rates.
Leveraging data analytics enables personalized customer experiences by analyzing customer data, allowing you to tailor products and services to individual needs.
Embracing data analytics offers numerous benefits for insurance companies, from providing customized customer experiences to optimizing risk assessment and boosting operational efficiency.
Data analytics significantly impacts the insurance industry, transforming how insurance companies operate and serve their customers.
It allows insurers to predict future trends and risks, reducing costs and making insurance more efficient and customer-centric.
By employing data analysis methods and methodologies, underwriting analytics can assess risk, detect possible claims, and determine suitable premiums to maintain long-term earnings and sustainability.
Improved risk estimation is one of the key advantages of predictive analytics in underwriting, enabling insurers to create statistical models that take into account various factors, including age, employment, health condition, credit score, and more.
For your interest: Homeowners Insurance Underwriting Process
Challenges and Limitations
The insurance industry is facing numerous difficulties that impact its business practices and customer-centric strategy. Overcoming these challenges requires effective data management and the use of modern technologies to improve decisions and operational effectiveness in the sector.
Insurance data analytics encounters several pivotal challenges that need to be properly addressed. These challenges include difficulties in data management and the use of modern technologies.
A unique perspective: Should I Get an Insurance Broker
Limitations and Challenges
The insurance industry faces numerous challenges that impact its business practices and customer-centric strategy. One of the major challenges is dealing with potential fraud or life impaired clients, which can create huge problems for insurers.
Customers may find the best company, but there's a possibility that the client is actually a fraud or life impaired, which can lead to significant issues for the insurer. This is a major concern for insurers.
Consistently evolving business environments are increasing competition and risk, making it difficult for insurers to stay ahead. Several other challenges, like theft and fraud, are also plaguing the insurance analytics business.
Consider reading: What Is Health Insurance Fraud
Data analytics collate more precise information about several transactions, product performance, customer satisfaction, etc. This is crucial for insurers to make informed decisions and improve their operations.
Effective data management and the use of modern technologies are essential to improve decisions and operational effectiveness in the sector. This is a key area where insurers can focus to overcome their challenges.
In spite of its capacity to transform the sector, insurance data analytics encounters several pivotal challenges that should be properly addressed before embracing it. These challenges include dealing with potential fraud or life impaired clients, evolving business environments, and other issues like theft and fraud.
A unique perspective: Health Insurance Fraud Penalty
Talent Shortage
The talent shortage in data analytics is a significant obstacle to fully harnessing the potential of data in the insurance sector. Insurers can establish partnerships with academic institutions to attract and cultivate the talent required to lead advanced analytics initiatives.
The integration of data analytics in insurance streamlines numerous operations, but it also highlights the need for skilled professionals.
To address this issue, insurers can provide competitive training programs to help attract and cultivate the talent required. This can help insurers stay ahead in the industry and unlock new opportunities for growth.
For another approach, see: Currency Analytics
How We Can Help
We can help insurance companies make data-driven decisions that optimize operations and maximize profitability. Rishabh Software leverages advanced data analytics tools and methodologies to empower insurance companies to make informed decisions.
With a deep understanding of insurance dynamics, our team of expert professionals helps build impeccable Data Analytics solutions. This includes data engineering, BI & visualization, advanced analytics, AI/ML, data science, or managed analytics services.
TriState Technology offers cutting-edge solutions to maximize the value of data. We use advanced analytics tools and methodologies to assist insurance companies reach unparalleled levels of personalization in customer interactions, risk management strategy optimization, and decision-making process improvement.
Our unified and 360 data analytics capabilities will keep your organization at the forefront of digital innovation. This means you can streamline your business and witness the change with the right data-driven innovation.
We use cutting-edge data analytics services tools and processes to help insurance firms make data-driven choices that improve processes and increase profitability.
Curious to learn more? Check out: Payment for Medical Services
Sources
- https://www.rishabhsoft.com/blog/data-analytics-in-insurance
- https://www.xenonstack.com/blog/data-analytics-in-insurance
- https://www.gooddata.com/solutions/insurance/
- https://www.tristatetechnology.com/blog/data-analytics-driving-innovation-in-insurance-sector
- https://pixelplex.io/blog/insurance-data-analytics-explained/
Featured Images: pexels.com