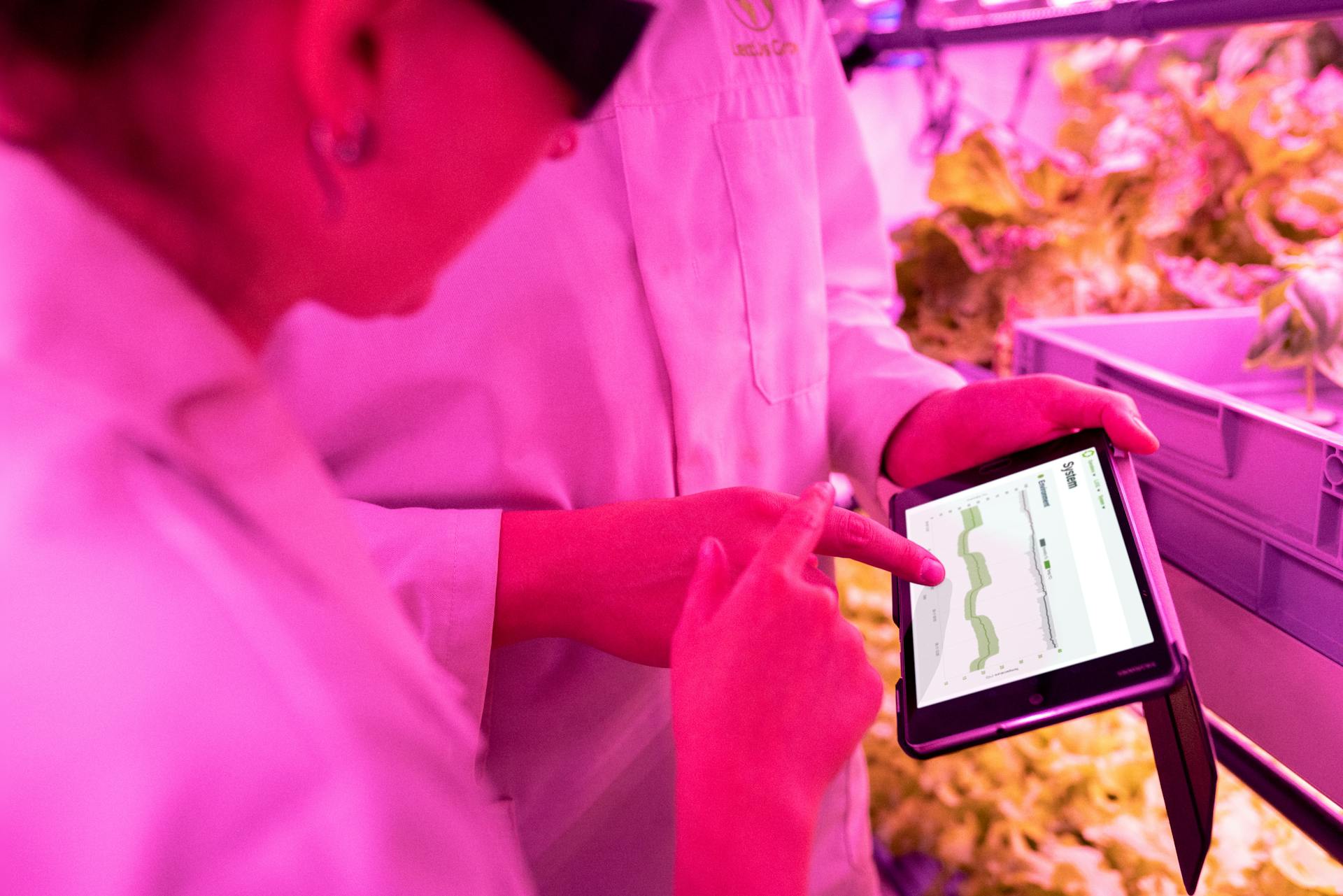
Data science is revolutionizing the insurance industry, allowing companies to make data-driven decisions and improve customer experiences. According to a study, 85% of insurers believe that data science is crucial to their business success.
With the help of machine learning algorithms, insurers can now identify high-risk customers and offer them personalized policies. This approach has been shown to reduce claims by 30% and increase customer satisfaction by 25%.
Data science also enables insurers to analyze large amounts of data quickly and accurately, making it easier to identify trends and patterns. For example, a leading insurer used data science to analyze customer behavior and identified a 20% increase in claims among customers who had recently moved.
By leveraging data science, insurers can transform their business operations and stay ahead of the competition.
Data Science in Insurance
Data science in insurance is a game-changer, and its applications are vast. It helps insurers identify new growth opportunities, protect their businesses from potential risks, and make informed decisions.
Predictive modeling is a key concept in data science for insurance, allowing for the forecast of future events using historical data. This is like the bread and butter of data science in insurance. Machine learning algorithms can also be used to detect fraud and assess risk, improving over time as they learn from data.
Data mining involves digging through large sets of data to find relevant patterns, which can be used to identify common factors in claims. Statistical models can be used to estimate the risk associated with insuring an individual or business, predicting the "what ifs." This is crucial for insurers to make informed decisions.
Data science in insurance helps insurers make decisions across various areas of their business, including risk assessment, fraud detection, claims processing, customer insights, operations, and market analysis. Small insurance companies can utilize data analytics in these ways:
- Risk Assessment: Use predictive analytics for accurate underwriting and pricing
- Fraud Detection: Implement ML algorithms to identify suspicious claims patterns
- Claims Processing: Automate routine claims for faster settlement
- Customer Insights: Analyze behavior data for targeted product offerings
- Operations: Optimize workflows and resource allocation through process analytics
- Market Analysis: Track trends and competitor data for strategic decisions
Data science in insurance also helps insurers optimize their pricing and service offerings. By analyzing consumer behavior, lifestyles, pricing sensitivity, and buying preferences, insurers can offer more tailored services and reduce the risk of over or underpaying claims. This is achieved through the use of predictive models, machine learning algorithms, and advanced analytics.
Insurance data analytics enhances claims processing through automation, resulting in enhanced efficiency and reduced costs. Predictive models can categorize claims according to priority, complexity, and potential costs, enabling automatic approval of simple claims. This helps insurers streamline their claims process, making it more efficient and customer-friendly.
Fraudulent claims detection is another critical application of data science in insurance. By leveraging predictive analytics and machine learning algorithms, insurers can identify suspicious claims patterns and behavioral patterns, subjecting them to further investigation. This proactive approach helps insurers detect and prevent fraud, maintaining the integrity of their operations.
Data science in insurance is a shield against fraud and deception, powered by advanced AI analytics and sophisticated algorithms. Insurers can analyze patterns and anomalies in vast datasets of claims history and customer interactions, identifying potentially fraudulent activities before they impact the bottom line. This proactive approach saves millions and maintains the integrity of insurance operations.
The application of data science in the insurance industry is like a goldmine, swimming in data from personal details to claims history. The trick is to sift through this data, find patterns, learn from them, and make predictions, using this data to make smarter decisions about pricing policies, assessing risks, or understanding customer needs.
Artificial Intelligence in Insurance
Artificial intelligence (AI) is transforming the insurance industry in numerous ways. AI-powered chatbots are being used to power intelligent customer interactions, generating personalized communications and significantly increasing customer satisfaction.
AI algorithms can analyze vast amounts of structured and unstructured data to predict claim probabilities with better accuracy. This enables insurers to detect fraudulent claims while fast-tracking legitimate ones.
Gen AI and Large Language Models (LLMs) are being used to extract key information from policy documents, delivering concise summaries and automating claims analysis. This technology is also being used to power intelligent chatbots and generate personalized communications.
State Farm, the largest auto insurance provider in the US, is using machine learning (ML) algorithms to analyze user driving patterns in real-time. They're also using ML algorithms in fraud detection and claims processing.
AXA, a global insurance company, is investing heavily in AI and data tools. They're using machine learning models to offer personalized recommendations to their customers, improving user satisfaction by tailoring policies based on their history and behavior.
Here are some key ways AI is being used in insurance:
- Predictive analytics for accurate underwriting and pricing
- Fraud detection using machine learning algorithms
- Claims processing automation for faster settlement
- Customer insights for targeted product offerings
- Operations optimization through process analytics
These are just a few examples of how AI is revolutionizing the insurance industry. As technology continues to advance, we can expect to see even more innovative applications of AI in insurance.
Benefits and Challenges
Data science in insurance has brought numerous benefits, including visual representation that brings insights to life for organizations managing high volumes of data. This allows brokers and underwriters to make fast, informed decisions from their preferred location or device.
Smart analytics solutions help organizations make decisions across various areas of their business. They help prioritize different risk categories, prevent fraud losses, and optimize expenses.
Data science has also changed the way insurance is priced, moving away from a one-size-fits-all approach. This is because data science analyzes a wider variety of impact factors for risk mitigation and pricing, which is especially important for large risk pools, such as the population of over 300 million in the US.
Evolving Demands
Modern consumers are driving change in the insurance industry, seeking tailored solutions that meet their unique needs and are delivered conveniently. They're also becoming increasingly price-conscious, prompting insurers to develop innovative strategies to meet these evolving expectations effectively.
Consumers expect personalized offerings that cater to their individual needs and preferences, which is where data analytics comes in. By analyzing customer data and preferences, insurers can create targeted insurance products that align with evolving customer demands.
Pricing optimization is another key area where data analytics plays a crucial role. Insurers can use data analytics to develop dynamic pricing models that respond to real-time market trends and customer behavior, allowing them to offer competitive pricing while meeting the price-conscious expectations of modern consumers.
Here are some key benefits of data-driven pricing:
- Personalized Offerings: Data analytics enables insurers to create targeted insurance products that cater to individual needs and preferences.
- Pricing Optimization: Insurers can use data analytics to develop dynamic pricing models that respond to real-time market trends and customer behavior.
These innovative strategies are essential for insurers to stay competitive in today's market, where traditional insurers face formidable challenges from newcomers who leverage advanced technology to introduce inventive insurance solutions.
Regulatory Impact on Volatility
Health insurance companies operate in a highly regulated environment, with federal, state, and local regulations posing challenges for planning and future business operations.
Changes in regulations can significantly impact business operations, introducing uncertainty and making it difficult to predict outcomes.
Data analytics can help track key metrics and monitor compliance with regulatory changes at all levels, allowing insurers to stay ahead of the curve.
Predictive analysis can also be used to anticipate forthcoming regulations, assess their potential impact, and prepare for compliance in advance, reducing the element of surprise.
Regulatory monitoring and predictive analysis can help health insurers navigate the complex regulatory landscape and make informed decisions.
Here are some ways data analytics can help with regulatory compliance:
- Regulatory Monitoring: Track key metrics and monitor compliance with regulatory changes.
- Predictive Analysis: Anticipate forthcoming regulations, assess their potential impact, and prepare for compliance in advance.
Overcoming Challenges
Data quality and accuracy are major challenges in insurance's data science integration. This is evident from the case of a health insurance company that faced data quality issues and resolved them by implementing an AI-driven system.
Integration with legacy systems is another common challenge. A legacy insurer overcame this challenge by adopting a hybrid cloud approach, allowing them to gradually migrate their processes without disrupting existing operations.
To overcome challenges, insurers need to evaluate their success or failure. This involves figuring out why something worked or didn't work, and identifying areas for improvement.
Here are some key takeaways from the challenges faced by insurers:
A Shield Against Fraud
Data analytics has revolutionized the insurance industry by providing a shield against fraud. Advanced AI analytics and sophisticated algorithms have made it possible to detect suspicious claims and fraudulent activities.
Predictive analytics has been instrumental in identifying patterns and anomalies in vast datasets of claims history and customer interactions. This allows insurers to detect potentially fraudulent activities before they impact the bottom line.
Fraud detection and prevention due to data science in insurance has saved millions of dollars. It effectively maintains the integrity of insurance operations by streamlining the claims process.
Predictive modeling techniques are applied to analyze patterns in fraud and screen false claims. This proactive approach deters fraud and ensures that legitimate claims are processed efficiently.
Here are some key ways data analytics helps prevent fraud in the insurance industry:
- Improvements in technologies have made it possible to detect suspicious claims and fraudulent activities.
- Predictive modeling techniques are applied to analyze patterns in fraud and screen false claims.
- Fraud detection and prevention due to data science in insurance has saved millions of dollars.
Data Science Process
The data science process in insurance involves several key steps. Data extraction, cleaning, and organization are essential before analysis can begin.
There are four types of analyses: descriptive, diagnostic, predictive, and prescriptive. Descriptive analysis tells you what has happened in the past, such as summarizing customer base and claims data to identify trends and patterns.
Predictive analysis predicts future trends based on past data, allowing insurance companies to develop and train predictive models. These models can be used to predict customer behaviors, market trends, and risk factors.
Here's a breakdown of the four types of analyses:
- Descriptive analysis: Summarizes past data to identify trends and patterns.
- Diagnostic analysis: Helps understand why something occurred.
- Predictive analysis: Predicts future trends based on past data.
- Prescriptive analytics: Provides recommendations for the future.
Future Trends
The data science process is constantly evolving, and understanding the future trends can help you stay ahead of the curve.
Future trends in data science are transforming the way insurance companies leverage data analytics.
One key emerging trend is the use of advanced analytics to optimize insurance underwriting, which can lead to more accurate risk assessments and better policy pricing.
Predictive analytics is also becoming increasingly important, allowing insurance companies to forecast future events and make data-driven decisions.
Machine learning algorithms are being used to analyze large datasets and identify patterns that can inform business decisions.
Real-time data analytics is also becoming more prevalent, enabling insurance companies to respond quickly to changing market conditions and customer needs.
These trends are transforming the insurance industry and will continue to shape the data science process in the years to come.
Problem Framing
Before diving into data analysis, it's essential to understand the problem you're trying to solve. A well-defined problem serves as a foundation for the entire analytics process, guiding subsequent stages.
Insurance companies need to identify specific challenges or questions they aim to address using data insights. These problems can range from optimizing premium pricing to predicting customer churn.
A clear problem statement helps focus the analytics process and ensures that data analysis is targeted towards solving a specific issue. This stage is crucial in setting the direction for the entire analytics process.
The problem framing stage helps insurance companies to identify areas where data insights can bring the most value. By understanding the problem they're trying to solve, they can create a roadmap for the analytics process.
A well-defined problem statement is essential for effective data analysis in insurance. It helps to ensure that the analytics process is targeted, efficient, and effective in solving the problem at hand.
Insurance companies can use data insights to identify new growth opportunities and protect their businesses from potential risks. By framing the problem correctly, they can create a solid foundation for the analytics process.
Collection
Collection is a crucial step in the data science process, and insurance companies have a wealth of data to work with.
Insurance companies have a vast amount of data, including customer details and past claims, which can be used to improve their services.
Data collection involves gathering the right information, as mentioned in Example 2, which highlights the importance of ensuring that the data is relevant and includes first-party and second-party data.
Here are some critical sources for collecting data in insurance:
- Customer Data and Demographics
- Device Data (e.g. car telematics, fitness trackers)
- Social Media Data
- Third-Party Data for Risk Assessment (e.g. credit history, public records)
- Historical Claims Data for Trend Analysis
These sources provide a comprehensive view of the customer, allowing insurers to create personalized policies and services.
Cleaning
Cleaning is a crucial step in the data science process. It involves extracting, cleaning, and processing valuable data from legacy systems, which can be a daunting task.
Extracting data requires expertise and efficient analytics software to handle complex data sets. This step is essential to uncover hidden insights and patterns within the data.
Data cleaning involves checking for errors, eliminating duplicates, handling outliers, filling in missing data, and ensuring the data is structured correctly. This process can be time-consuming, but it's necessary to ensure the accuracy of the data.
Imagine trying to complete a jigsaw puzzle with missing pieces – it's similar to dealing with data quality issues. Insurers often struggle with complete, accurate, and updated data, which can lead to misleading insights and destroy trust in data science.
To give you an idea of the challenges involved in data cleaning, here are some common issues that arise:
- Errors in data entry
- Duplicates in the data set
- Outliers that skew the data
- Missing data that needs to be filled in
Data cleaning is a critical step in the data science process, and it requires attention to detail and a thorough understanding of the data. By cleaning and processing the data, you can uncover valuable insights and make informed decisions.
Model Development
Model Development is a crucial stage in the data science process, where you analyze your data to identify patterns, trends, and correlations. This stage is all about asking the right questions and getting the right answers.
There are four types of analyses that you can perform during this stage: Descriptive, Diagnostic, Predictive, and Prescriptive. Descriptive analysis tells you what has happened in the past, while Diagnostic analysis helps you understand why something occurred. Predictive analysis predicts future trends based on past data, and Prescriptive analytics provides recommendations for the future.
Predictive modeling is a key component of predictive analysis, and it involves using historical data to forecast future events. This can be used in risk assessment and pricing to ensure that insurance premiums are fair and accurate. By identifying patterns and correlations in past events, insurers can more accurately forecast potential risks and determine the right price for their policies.
Here are the four types of analyses in more detail:
- Descriptive analysis: tells you what has happened in the past
- Diagnostic analysis: helps you understand why something occurred
- Predictive analysis: predicts future trends based on past data
- Prescriptive analytics: provides recommendations for the future
Industry and Company
The insurance industry has seen significant changes in recent years, with companies like MetLife and Zurich Insurance Group leveraging data science to stay competitive.
MetLife, with over 90 million customers in 60 countries, relies heavily on predictive modeling to combat fraud and assess mortality risk more precisely.
Their data science teams continuously work on improving risk assessment models, allowing them to offer customers better policy rates and manage financial risks more efficiently.
Zurich Insurance Group, operating in over 215 countries, has also harnessed the power of AI to automate routine claims and settle them faster.
AI models assist claims handlers by identifying potential frauds and predicting outcomes based on previous data, enhancing their claims process.
Industry Improvement
The insurance industry is constantly evolving, and data science is playing a significant role in its improvement. With the help of predictive analytics, companies can now assess risk more accurately and offer better policy rates to customers. This is evident in the case of MetLife, which has been using predictive modeling to combat fraud and improve risk assessment models.
Data science is also being used to enhance customer satisfaction. By digitally capturing customer feedback and analyzing their buying behavior, companies can provide a better experience. For instance, Progressive Insurance's Snapshot Program uses real-time driving data to offer personalized insurance rates based on customers' driving habits.
Automating routine claims has also become a reality, thanks to the use of AI and machine learning algorithms. Companies like Zurich Insurance Group and Lemonade are using these technologies to settle claims faster and detect potential frauds. In fact, Lemonade's AI claims bot, A.I. Jim, can review a claim in just three seconds and initiate a bank transfer.
Here's a list of some of the key benefits of using data science in the insurance industry:
- Improved risk assessment and underwriting
- Enhanced customer satisfaction and experience
- Increased efficiency in claims processing
- Reduced risk of fraud
- More accurate pricing and policy rates
As a result of these advancements, the insurance industry is becoming more efficient and customer-centric. Companies are now able to offer more personalized services and products, which is leading to improved customer satisfaction and loyalty.
Industry Standards
Industry Standards are crucial in the insurance industry, where data science plays a vital role in extracting insights and knowledge from data.
Data science in insurance involves various disciplines, including statistics, machine learning, predictive analytics, and AI generally. These disciplines work together to provide a comprehensive understanding of the industry and its trends.
To ensure consistency and accuracy, insurance companies must adhere to established industry standards. This includes implementing robust data management systems and using proven methodologies to analyze data.
Insurance companies must also invest in ongoing training and education for their data science teams, to keep up with the latest advancements in machine learning and AI. This will enable them to stay competitive and provide better insights to their clients.
Sources
- https://www.rishabhsoft.com/blog/data-analytics-in-insurance
- https://www.damcogroup.com/blogs/insurance-data-analytics-for-insurers
- https://www.discoverdatascience.org/industries/insurance/
- https://dataforest.ai/blog/reshaping-the-insurance-landscape-the-power-of-data-science
- https://datalemur.com/blog/insurance-data-science
Featured Images: pexels.com