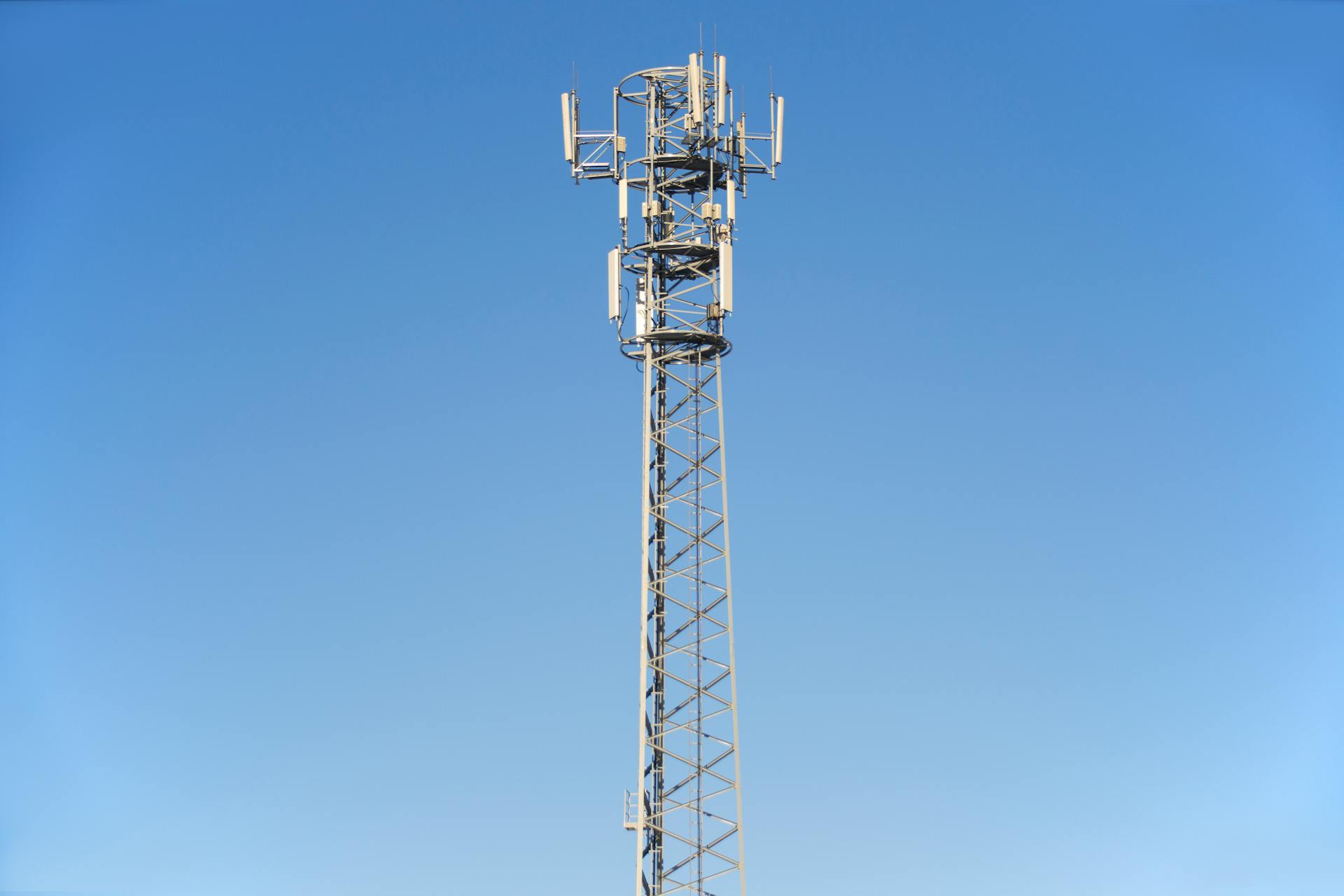
Algorithmic trading uses pre-programmed instructions to automatically execute trades based on specific conditions, such as price movements or time of day. This type of trading can be done on various markets and asset classes.
High frequency trading involves executing a large number of trades in a very short period of time, often in fractions of a second. This can be done using sophisticated algorithms and high-speed computers.
The goal of algorithmic trading is to capitalize on small price discrepancies and execute trades more quickly and efficiently than human traders. Algorithmic trading can be used to trade a wide range of assets, including stocks, bonds, and currencies.
Algorithmic trading can be classified into two main categories: discretionary and non-discretionary. Discretionary algorithmic trading allows for human intervention and decision-making, while non-discretionary algorithmic trading relies solely on pre-programmed instructions.
For more insights, see: Algorithmic Trading with Python
History and Development
The history of high-frequency trading is a fascinating story that's been unfolding since 1983, when NASDAQ introduced a purely electronic form of trading.
This marked the beginning of a rapid evolution, where execution times decreased from several seconds to milli- and even microseconds by 2010.
High-frequency trading was still a little-known topic outside the financial sector until 2009, when the New York Times published an article that brought it to the public's attention in July.
In 2013, Italy became the world's first country to introduce a tax specifically targeted at HFT, charging a levy of 0.02% on equity transactions lasting less than 0.5 seconds on September 2.
Types of High-Frequency Trading
High-frequency trading encompasses various strategies, but four key categories stand out. Market-making based on order flow is one such strategy, where firms provide liquidity to the market by buying and selling securities.
High-frequency funds started to become especially popular in 2007 and 2008, and many HFT firms are market makers, which has lowered volatility and helped narrow bid–offer spreads, making trading and investing cheaper for other market participants.
Another category is market-making based on tick data information, which uses real-time data to make trades. This strategy is often used by firms like Chicago Trading Company and Optiver.
Event arbitrage and statistical arbitrage are also key HFT strategies. Event arbitrage involves exploiting temporary deviations from stable relationships among securities, while statistical arbitrage uses complex models to identify profitable trades.
Statistical arbitrage at high frequencies is actively used in all liquid securities, including equities, bonds, futures, foreign exchange, etc., and has generated annual aggregate profits exceeding $21 billion in 2009.
Statistical Arbitrage
Statistical arbitrage is a type of high-frequency trading that exploits predictable temporary deviations from stable statistical relationships among securities.
This strategy involves using models of greater complexity involving many more than four securities to identify potential arbitrage opportunities.
High-frequency trading allows for similar arbitrages to be made, and The TABB Group estimates that annual aggregate profits of high-frequency arbitrage strategies exceeded US$21 billion in 2009.
Statistical arbitrage strategies can be applied in all asset classes, including equities, bonds, futures, foreign exchange, and more.
These strategies may also involve classical arbitrage strategies, such as covered interest rate parity in the foreign exchange market.
High-frequency trading allows for the use of more complex models, enabling traders to identify potential arbitrage opportunities that might have gone unnoticed before.
The Purdue study estimates that the profits for all high-frequency trading were US$5 billion in 2009, although this figure is likely an underestimate.
Statistical arbitrage at high frequencies is actively used in all liquid securities, including equities, bonds, futures, foreign exchange, etc.
By leveraging statistical relationships and models, traders can identify potential arbitrage opportunities and capitalize on them before the market adjusts.
A fresh viewpoint: Thinkorswim Futures
Scalping
Scalping is liquidity provision by non-traditional market makers, whereby traders attempt to earn the bid-ask spread by establishing and liquidating a position quickly, usually within minutes or less.
Scalpers normally involve sophisticated trading systems and technology, trading volumes that are many times more than the average individual, and are bound by exchange rules stipulating their minimum quote obligations.
Market makers are basically specialized scalpers, referred to as dealers, and are required to post at least one bid and one ask at some price level to maintain a two-sided market for each stock represented.
Scalping can be a profitable strategy as long as price movements are less than the bid-ask spread, which can be a significant advantage for high-frequency traders.
Some high-frequency trading firms, such as Chicago Trading Company and Optiver, may engage in scalping as part of their trading strategies, using their advanced technology and systems to execute trades quickly and efficiently.
Scalping is one of the four key categories of HFT strategies, along with market-making based on order flow, market-making based on tick data information, and event arbitrage and statistical arbitrage.
Discover more: Equity Market Making
Indicator-Based vs Manual
Indicator-Based trading relies on algorithms that analyze vast amounts of market data to make decisions. These algorithms can process data faster than humans, making them ideal for high-frequency trading.
The key difference between Indicator-Based and Manual trading is the level of human intervention. Manual trading relies on human traders to make decisions based on their analysis of the market.
Indicator-Based trading can execute trades in as little as 10 milliseconds, compared to Manual trading which can take several seconds or even minutes. This speed advantage is crucial in high-frequency trading where market conditions can change rapidly.
In a high-frequency trading environment, Manual trading can be too slow to keep up with the pace of the market. Indicator-Based trading can analyze market data and make decisions without the need for human intervention, making it a more efficient option.
Some Popular Firms
Some of the popular High-Frequency Trading (HFT) firms in the US are Citadel Securities, Virtu Financial, and Jump Trading.
These firms are well-established players in the US market, with a strong presence in the HFT space.
In the Netherlands, two notable HFT organisations are Optiver and Flow Traders, which have gained significant traction in the region.
Tower Research, based in Gurgaon, is one of the largest HFT companies in India, along with Goldman Sachs, Morgan Stanley, and iRageCapital, all of which have a presence in major Indian cities like Bengaluru, Mumbai, and Gurgaon.
See what others are reading: Best Brokerage Firms for Day Trading
Technological Aspects
Algorithmic trading strategies are implemented using modern programming languages, but some still use spreadsheets. Increasingly, large brokerages and asset managers write their algorithms to the FIX Protocol's Algorithmic Trading Definition Language (FIXatdl), which allows firms to specify exactly how their electronic orders should be expressed.
Basic models can rely on as little as a linear regression, while more complex game-theoretic and pattern recognition or predictive models can be used to initiate trading. More complex methods such as Markov chain Monte Carlo have been used to create these models.
Low-latency trading systems are designed to rapidly execute financial transactions, with most HFT firms relying on low latency execution of their trading strategies. Network-induced latency, or delay, is a critical factor in low-latency trading, with most HFT firms aiming for ultra-low latency networks.
Low-latency traders profit by providing information to their algorithms microseconds faster than their competitors. They use advanced technology, such as microwave and shortwave transmission, to achieve ultra-low latency. Some HFT firms also use satellites to transmit market data.
You might enjoy: Schwab Algorithmic Trading
The traditional trading system consists of two blocks: market data reception and order request sending. However, an algorithmic trading system can be broken down into three parts: exchange, server, and application. The server receives data and acts as a store for historical database, while the application side analyzes the data and sends orders to the exchange.
Low-latency trading systems have evolved to include complex event processing engines (CEP), which are used for order routing and risk management. The FIX protocol has also made it easier to connect to different destinations, reducing the go-to market time.
Here are some key features of advanced trading platforms:
- Ultra-low latency direct market access (ULLDMA) systems can handle high amounts of volume and boast round-trip order execution speeds of 10 milliseconds or less.
- Hardware acceleration or full-hardware processing of incoming market data is used to achieve these speeds.
- Some companies provide full-hardware appliances based on FPGA technology to obtain sub-microsecond end-to-end market data processing.
HFT operates at incredibly high speeds, with trades executed in fractions of a second. Some of the fastest can execute trades in microseconds or even nanoseconds.
Risks and Controversy
Algorithmic and high frequency trading have faced criticism for their potential to distort the market. Frontrunning by wholesalers is a notable concern, as seen in the case of Citadel Securities being fined $700,000 by FINRA in 2020.
The use of immediate-or-cancel orders and filling gaps in bid prices can also lead to market manipulation. Citadel Securities was fined ₩11.88 billion ($9.66 million) by South Korea's financial regulator in January 2023 for distorting stock prices through these practices.
Athena Capital Research LLC was fined $1 million in 2014 for price manipulation charges, using $40 million to rig prices of thousands of stocks, including eBay. This case highlighted the use of high-powered computers and complex algorithms to manipulate closing prices.
Risks and Controversy
High-speed trading firms have been involved in some shady practices. Citadel Securities was fined $700,000 in 2020 for frontrunning customer orders.
Market manipulation is a serious issue in the world of high-frequency trading. Athena Capital Research LLC was fined $1 million in 2014 for price manipulation charges, using $40 million to rig prices of thousands of stocks.
The high-speed trading industry has faced accusations of cheating slower investors. Reporting by Bloomberg noted that the HFT industry is "besieged by accusations that it cheats slower investors".
Immediate-or-cancel orders and filling gaps in bid prices can be used to distort stock prices. Citadel Securities was fined ₩11.88 billion ($9.66 million) in 2023 for using these tactics in South Korea.
Manipulation
Manipulation is a serious issue in the financial world, and it's not just a matter of getting a good deal. Spoofing, a type of manipulation, involves placing orders to create a false impression of market demand, allowing traders to buy or sell at a more favorable price. This can be done by placing limit orders outside the current bid or ask price, making it seem like there's more demand or supply than there really is.
Spoofing can be done to temporarily manipulate the market, allowing traders to place trades based on the artificial change in price before canceling the limit orders. For example, a trader might place a buy order at $20.10, creating a false bid price, and then execute a market order to sell shares at the artificially inflated price.
In 2013, Panther Energy Trading LLC was ordered to pay $4.5 million to U.S. and U.K. regulators for manipulating commodity markets through spoofing. The firm's algorithms placed and quickly canceled bids and offers in futures contracts, creating a false impression of market demand.
Here's an interesting read: Great Day Traders
Spoofing is not the only form of manipulation; quote stuffing is another tactic used by malicious traders. This involves quickly entering and withdrawing large quantities of orders to flood the market and gain an advantage over slower traders. The rapidly placed and canceled orders can cause market data feeds to delay price quotes, giving high-frequency traders an edge.
In 2014, Athena Capital Research LLC was fined $1 million for price manipulation charges. The firm used high-powered computers and complex algorithms to rig prices of thousands of stocks, including eBay. The SEC said the firm manipulated closing prices commonly used to track stock performance.
Market manipulation can take many forms, and it's not just limited to spoofing and quote stuffing. Frontrunning, for example, involves trading ahead of customer orders, which can give traders an unfair advantage. In 2020, Citadel Securities was fined $700,000 by FINRA for frontrunning customer orders.
Here are some examples of market manipulation:
- Spoofing: placing orders to create a false impression of market demand
- Quote stuffing: quickly entering and withdrawing large quantities of orders to flood the market
- Frontrunning: trading ahead of customer orders
- Price manipulation: rigging prices of stocks or other financial instruments
These tactics can have serious consequences for investors and the market as a whole. It's essential to be aware of the risks and take steps to protect yourself from manipulation.
Sources
- https://en.wikipedia.org/wiki/Algorithmic_trading
- https://en.wikipedia.org/wiki/High-frequency_trading
- https://www.schwab.com/learn/story/high-frequency-algorithmic-trading
- https://www.utradealgos.com/blog/high-frequency-algorithmic-trading
- https://cryptoslate.com/algorithms-for-all-demystifying-algo-trading-in-crypto-markets/
Featured Images: pexels.com